Predicting patient response to cancer therapy via histopathology images.
Journal of clinical oncology(2022)
摘要
e13561 Background: Histopathology has long been considered the gold standard of clinical diagnosis and prognosis in cancer. In recent years, molecular markers including tumor gene expression have proven increasingly valuable for enhancing diagnosis and precision oncology. Here we ask if we can predict tumor gene expression from its histopathology images and based on the latter, predict patient survival and treatment response. Methods: We developed DeepPT (Deep Pathology for Treatment), a deep learning framework that predicts gene expression directly from histopathology tumor images. DeepPT is composed of three main components: a pre-trained convolutional neural network model for feature extraction, an auto-encoder for feature compression, and a multiple-layer perceptron for regression. This architecture enables the model to capitalize on the similarity among the gene expressions and benefit from the advantages of multitask learning. Results: DeepPT was trained with haematoxylin and eosin stained (H&E) tumor slides from lung and breast cancer patients and their corresponding gene expression profiles. The models were then used to predict gene expression from five different held-out datasets, using nested cross validation. A total of approximately 23,000 genes were considered in this study; out of these, over 99% had a positive correlation between predicted and actual values, commonly for lung and breast cancer. Furthermore, a record number of genes (2,541 and 1,197 genes for lung and breast cancer, respectively) had a correlation above 0.4, well over the results of the current state-of-the-art approach (1,550 and 786 genes, respectively). We next studied if the inferred gene expression could be used for H&E-based personalized medicine. To this end, we used the predicted tumor transcriptomics generated by DeepPT as input to ENLIGHT, a platform that predicts a patient’s response to treatment from their tumor transcriptomics. We found that ENLIGHT matching scores based on DeepPT outputs were indeed associated with response to treatment. Conclusions: DeepPT is the first computational approach for building response predictors that can infer therapy response directly from whole slide images of patient biopsies. Importantly, its future application promises to make precision oncology more accessible to physicians and patients in the developing world.
更多查看译文
AI 理解论文
溯源树
样例
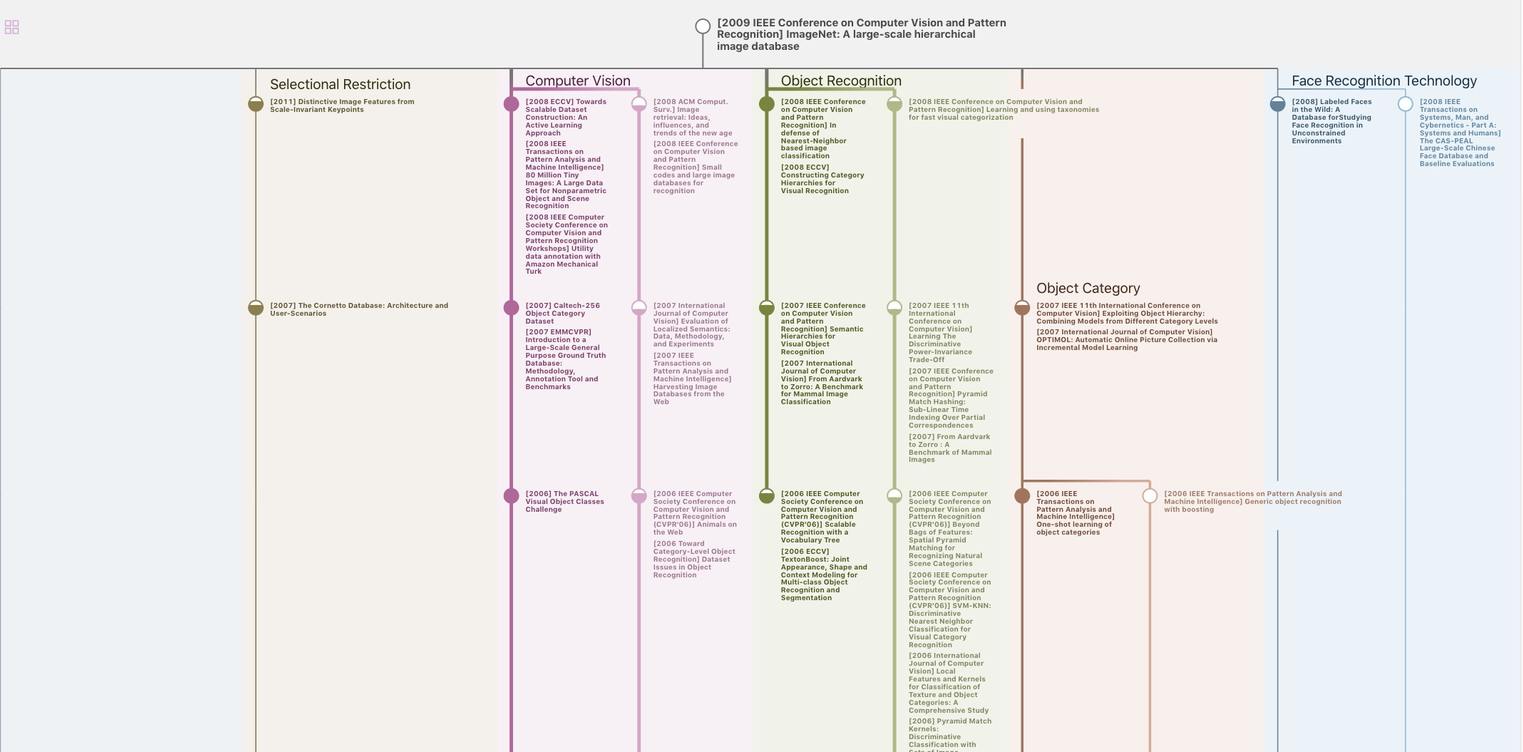
生成溯源树,研究论文发展脉络
Chat Paper
正在生成论文摘要