Explainability in Deep Reinforcement Learning: A Review into Current Methods and Applications
arxiv(2024)
摘要
The use of Deep Reinforcement Learning (DRL) schemes has increased dramatically since their first introduction in 2015. Though uses in many different applications are being found, they still have a problem with the lack of interpretability. This has bread a lack of understanding and trust in the use of DRL solutions from researchers and the general public. To solve this problem, the field of Explainable Artificial Intelligence has emerged. This entails a variety of different methods that look to open the DRL black boxes, ranging from the use of interpretable symbolic Decision Trees to numerical methods like Shapley Values. This review looks at which methods are being used and for which applications. This is done to identify which models are the best suited to each application or if a method is being underutilised.
更多查看译文
关键词
Deep reinforcement learning,DRL,Explainable AI,XAI,neural networks,Survey,Review
AI 理解论文
溯源树
样例
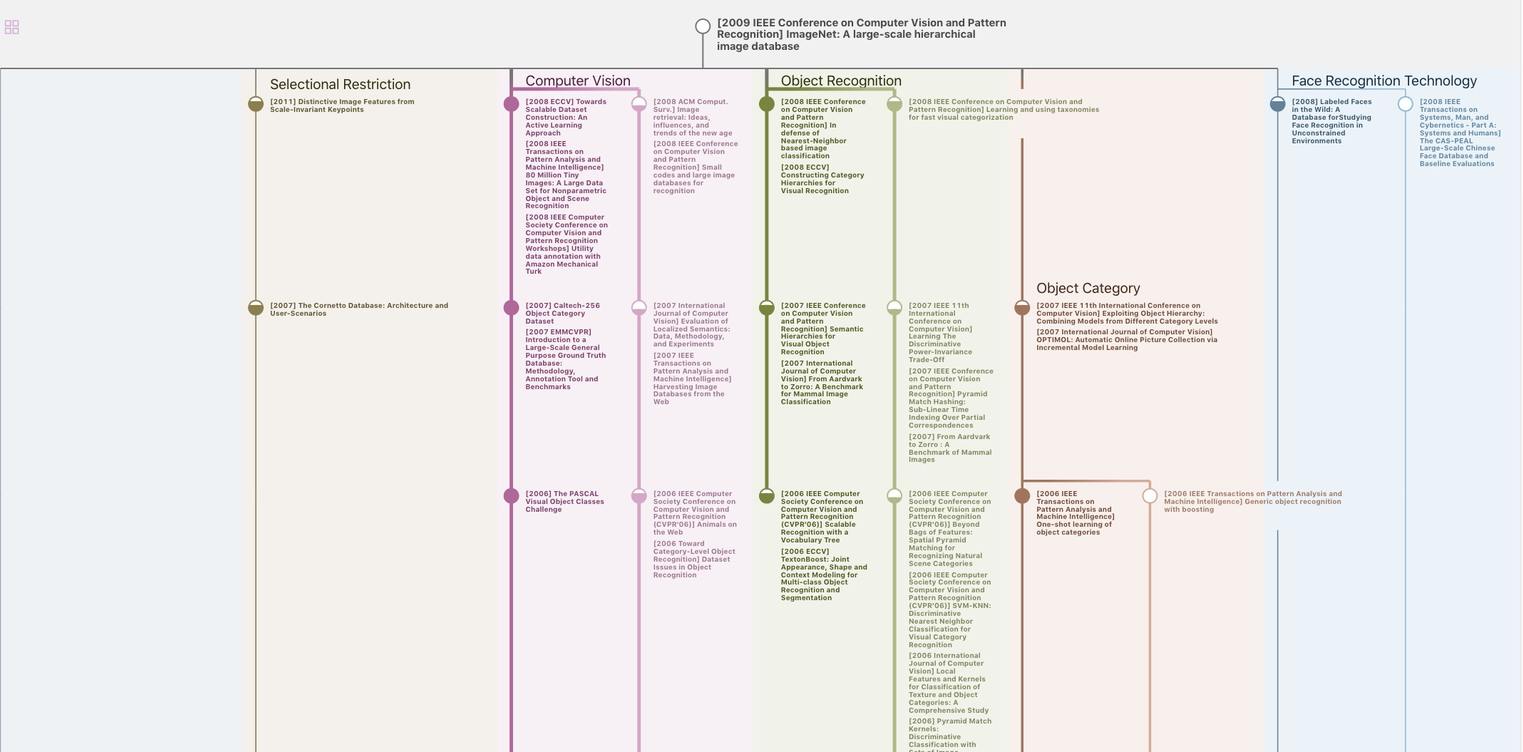
生成溯源树,研究论文发展脉络
Chat Paper
正在生成论文摘要