Safe Decision-making for Lane-change of Autonomous Vehicles via Human Demonstration-aided Reinforcement Learning
2022 IEEE 25TH INTERNATIONAL CONFERENCE ON INTELLIGENT TRANSPORTATION SYSTEMS (ITSC)(2022)
摘要
Decision-making is critical for lane change in autonomous driving. Reinforcement learning (RL) algorithms aim to identify the values of behaviors in various situations and thus they become a promising pathway to address the decisionmaking problem. However, poor runtime safety hinders RLbased decision-making strategies from complex driving tasks in practice. To address this problem, human demonstrations are incorporated into the RL-based decision-making strategy in this paper. Decisions made by human subjects in a driving simulator are treated as safe demonstrations, which are stored into the replay buffer and then utilized to enhance the training process of RL. A complex lane change task in an off-ramp scenario is established to examine the performance of the developed strategy. Simulation results suggest that human demonstrations can effectively improve the safety of decisions of RL. And the proposed strategy surpasses other existing learning-based decision-making strategies with respect to multiple driving performances.
更多查看译文
AI 理解论文
溯源树
样例
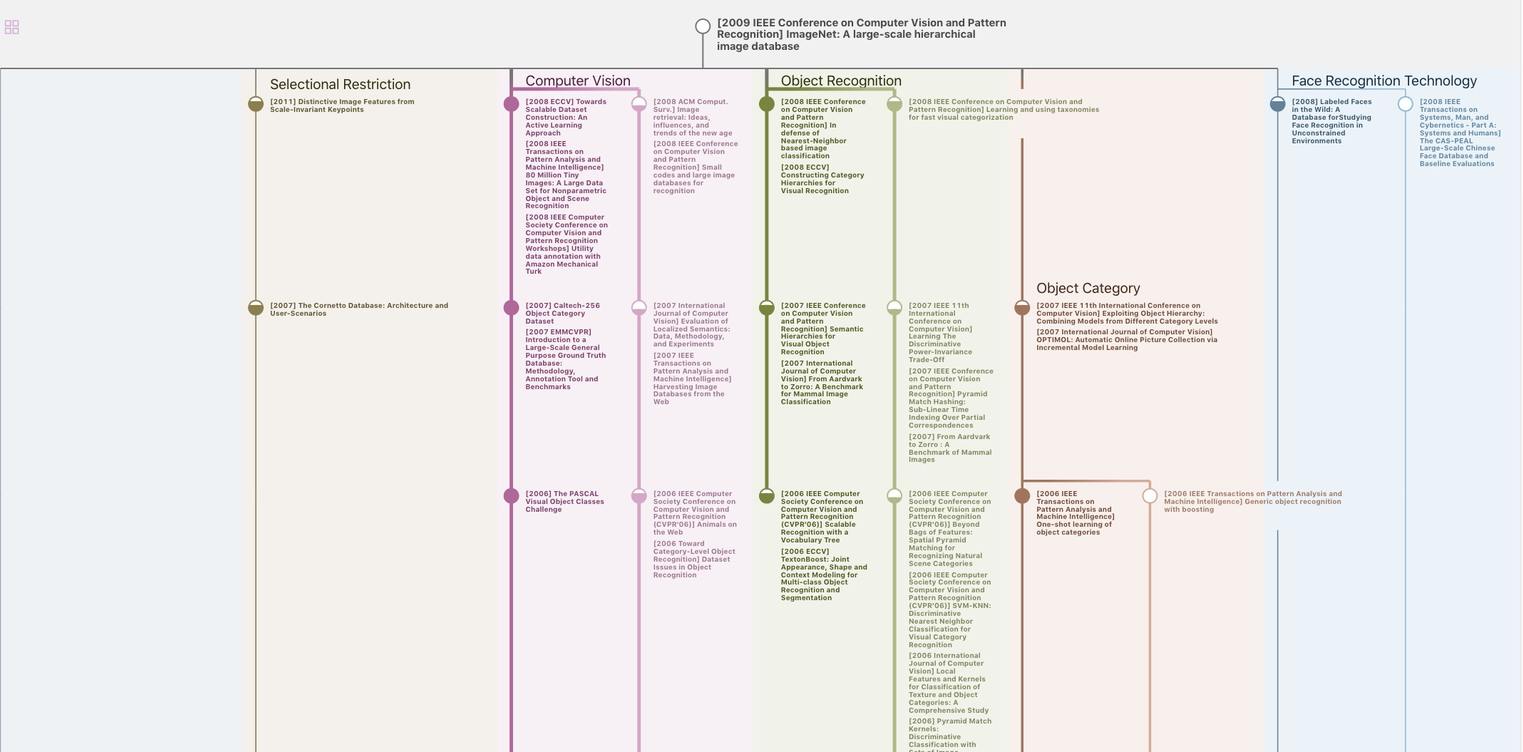
生成溯源树,研究论文发展脉络
Chat Paper
正在生成论文摘要