Show, Deconfound and Tell: Image Captioning with Causal Inference
China Univ Min & Technol | Southeast Univ
Abstract
The transformer-based encoder-decoder framework has shown remarkable performance in image captioning. However, most transformer-based captioning methods ever overlook two kinds of elusive confounders: the visual confounder and the linguistic confounder, which generally lead to harmful bias, induce the spurious correlations during training, and degrade the model generalization. In this paper, we first use Structural Causal Models (SCMs) to show how two confounders damage the image captioning. Then we apply the backdoor adjustment to propose a novel causal inference based image captioning (CIIC) framework, which consists of an interventional object detector (IOD) and an interventional transformer decoder (ITD) to jointly confront both confounders. In the encoding stage, the IOD is able to disentangle the region-based visual features by deconfounding the visual confounder. In the decoding stage, the ITD introduces causal intervention into the transformer decoder and deconfounds the visual and linguistic confounders simultaneously. Two modules collaborate with each other to alleviate the spurious correlations caused by the unobserved confounders. When tested on MSCOCO, our proposal significantly outperforms the state-of-the-art encoder-decoder models on Karpathy split and online test split. Code is published in https://github.com/CUMTGG/CIIC.
MoreTranslated text
Key words
Vision + language,Computer vision theory,Deep learning architectures and techniques,Efficient learning and inferences,Explainable computer vision,Machine learning,Recognition: detection,categorization,retrieval,Representation learning,Robot vision,Vision applications and systems,Visual reasoning
PDF
View via Publisher
AI Read Science
AI Summary
AI Summary is the key point extracted automatically understanding the full text of the paper, including the background, methods, results, conclusions, icons and other key content, so that you can get the outline of the paper at a glance.
Example
Background
Key content
Introduction
Methods
Results
Related work
Fund
Key content
- Pretraining has recently greatly promoted the development of natural language processing (NLP)
- We show that M6 outperforms the baselines in multimodal downstream tasks, and the large M6 with 10 parameters can reach a better performance
- We propose a method called M6 that is able to process information of multiple modalities and perform both single-modal and cross-modal understanding and generation
- The model is scaled to large model with 10 billion parameters with sophisticated deployment, and the 10 -parameter M6-large is the largest pretrained model in Chinese
- Experimental results show that our proposed M6 outperforms the baseline in a number of downstream tasks concerning both single modality and multiple modalities We will continue the pretraining of extremely large models by increasing data to explore the limit of its performance
Try using models to generate summary,it takes about 60s
Must-Reading Tree
Example
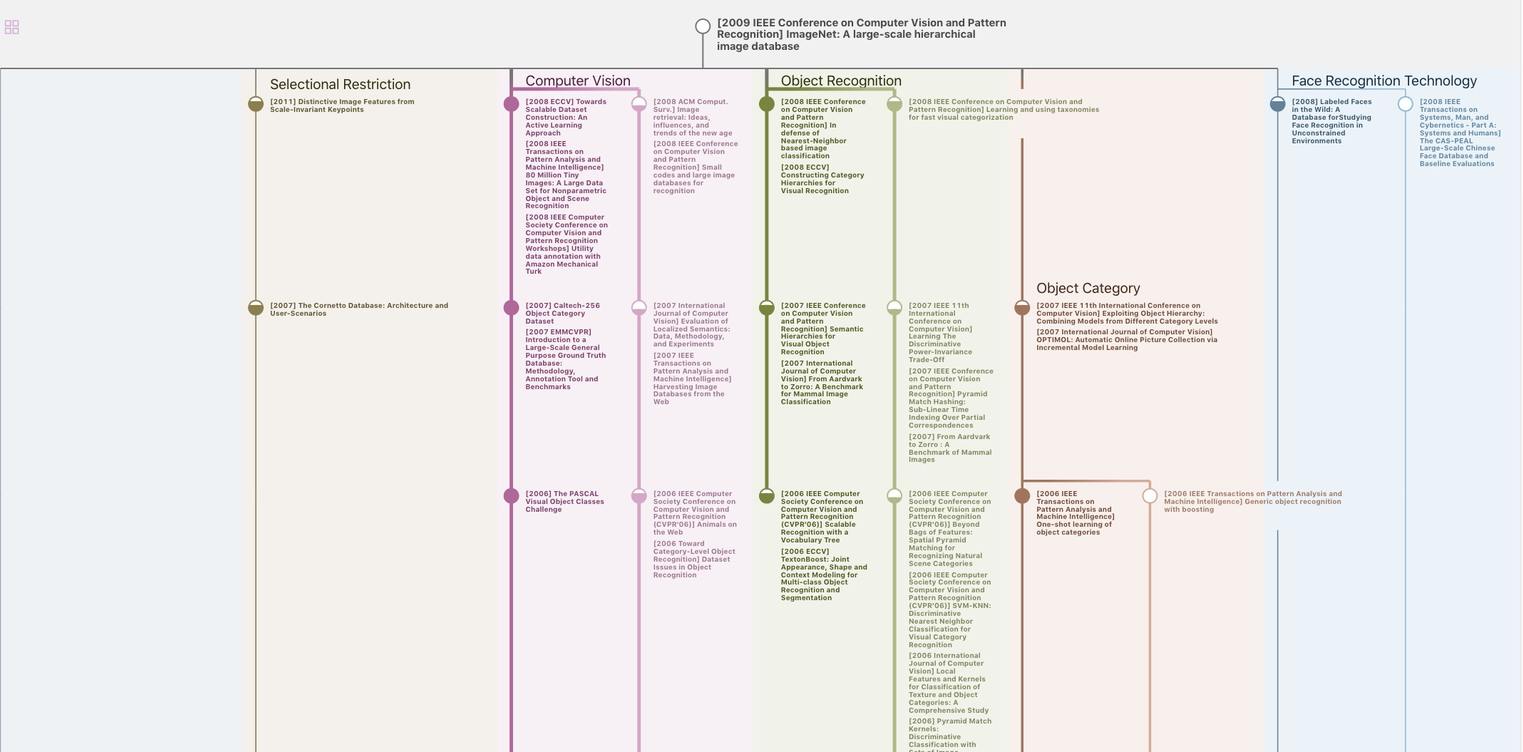
Generate MRT to find the research sequence of this paper
Related Papers
2005
被引用9333 | 浏览
2017
被引用5784 | 浏览
2021
被引用205 | 浏览
Data Disclaimer
The page data are from open Internet sources, cooperative publishers and automatic analysis results through AI technology. We do not make any commitments and guarantees for the validity, accuracy, correctness, reliability, completeness and timeliness of the page data. If you have any questions, please contact us by email: report@aminer.cn
Chat Paper
去 AI 文献库 对话