Time Is MattEr: Temporal Self-supervision for Video Transformers.
International Conference on Machine Learning(2022)
摘要
Understanding temporal dynamics of video is an essential aspect of learning better video representations. Recently, transformer-based architectural designs have been extensively explored for video tasks due to their capability to capture long-term dependency of input sequences. However, we found that these Video Transformers are still biased to learn spatial dynamics rather than temporal ones, and debiasing the spurious correlation is critical for their performance. Based on the observations, we design simple yet effective self-supervised tasks for video models to learn temporal dynamics better. Specifically, for debiasing the spatial bias, our method learns the temporal order of video frames as extra self-supervision and enforces the randomly shuffled frames to have low-confidence outputs. Also, our method learns the temporal flow direction of video tokens among consecutive frames for enhancing the correlation toward temporal dynamics. Under various video action recognition tasks, we demonstrate the effectiveness of our method and its compatibility with state-of-the-art Video Transformers.
更多查看译文
关键词
video transformers,temporal,self-supervision
AI 理解论文
溯源树
样例
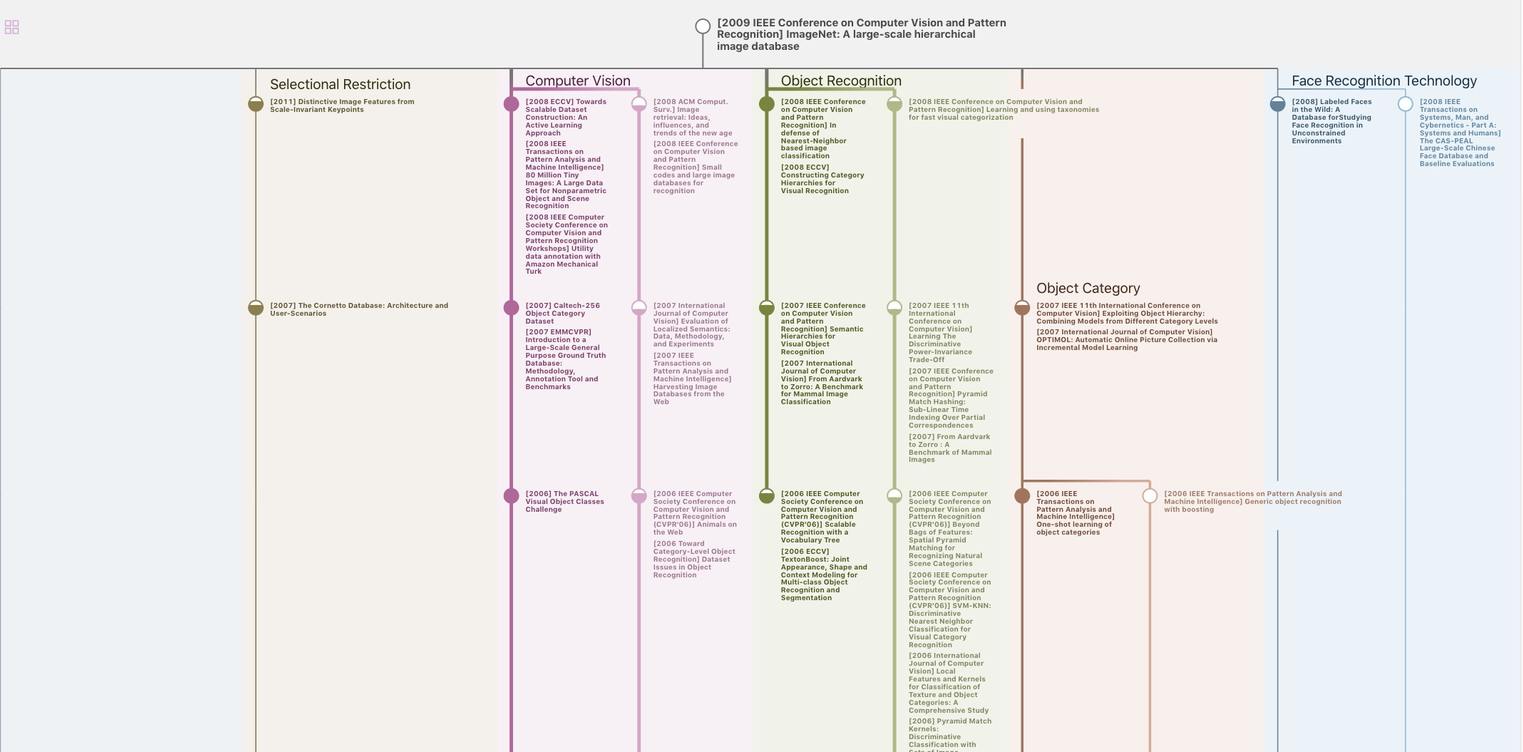
生成溯源树,研究论文发展脉络
Chat Paper
正在生成论文摘要