Variance Reduced Random Relaxed Projection Method for Constrained Finite-sum Minimization Problems
IEEE TRANSACTIONS ON SIGNAL PROCESSING(2024)
摘要
For many applications in signal processing and machine learning, we are tasked with minimizing a large sum of convex functions subject to a large number of convex constraints. In this paper, we devise a new random projection method (RPM) to efficiently solve this problem. Compared with existing RPMs, our proposed algorithm features two useful algorithmic ideas. First, at each iteration, instead of projecting onto the subset defined by one of the constraints, our algorithm only requires projecting onto a half-space approximation of the subset, which significantly reduces the computational cost as it admits a closed-form formula. Second, to exploit the structure that the objective is a sum, variance reduction is incorporated into our algorithm to further improve the performance. As theoretical contributions, under a novel error bound condition and other standard assumptions, we prove that the proposed RPM converges to an optimal solution and that both optimality and feasibility gaps vanish at a sublinear rate. In particular, via a new analysis framework, we show that our RPM attains a faster convergence rate in optimality gap than existing RPMs when the objective function has a Lipschitz continuous gradient, capitalizing the benefit of the variance reduction. We also provide sufficient conditions for the error bound condition to hold. Experiments on a beamforming problem and a robust classification problem are also presented to demonstrate the superiority of our RPM over existing ones.
更多查看译文
关键词
Constrained Optimization,Finite-Sum Minimization,Random Projection Method,Relaxed Projection,Variance Reduction,Convergence Rate Analysis
AI 理解论文
溯源树
样例
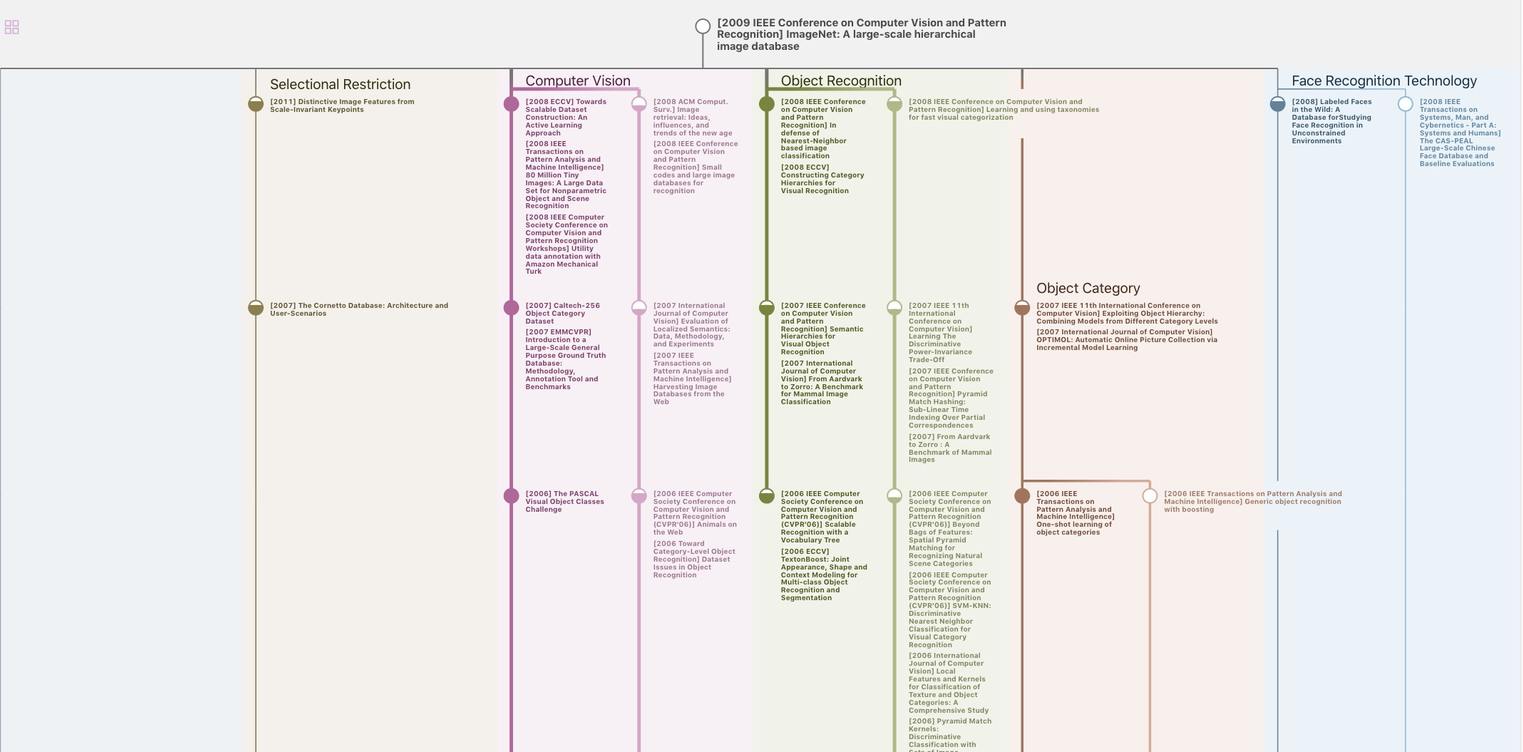
生成溯源树,研究论文发展脉络
Chat Paper
正在生成论文摘要