Segmentation-Free PVC for Cardiac SPECT Using a Densely-Connected Multi-Dimensional Dynamic Network.
IEEE transactions on medical imaging(2023)
摘要
In nuclear imaging, limited resolution causes partial volume effects (PVEs) that affect image sharpness and quantitative accuracy. Partial volume correction (PVC) methods incorporating high-resolution anatomical information from CT or MRI have been demonstrated to be effective. However, such anatomical-guided methods typically require tedious image registration and segmentation steps. Accurately segmented organ templates are also hard to obtain, particularly in cardiac SPECT imaging, due to the lack of hybrid SPECT/CT scanners with high-end CT and associated motion artifacts. Slight mis-registration/mis-segmentation would result in severe degradation in image quality after PVC. In this work, we develop a deep-learning-based method for fast cardiac SPECT PVC without anatomical information and associated organ segmentation. The proposed network involves a densely-connected multi-dimensional dynamic mechanism, allowing the convolutional kernels to be adapted based on the input images, even after the network is fully trained. Intramyocardial blood volume (IMBV) is introduced as an additional clinical-relevant loss function for network optimization. The proposed network demonstrated promising performance on 28 canine studies acquired on a GE Discovery NM/CT 570c dedicated cardiac SPECT scanner with a 64-slice CT using Technetium-99m-labeled red blood cells. This work showed that the proposed network with densely-connected dynamic mechanism produced superior results compared with the same network without such mechanism. Results also showed that the proposed network without anatomical information could produce images with statistically comparable IMBV measurements to the images generated by anatomical-guided PVC methods, which could be helpful in clinical translation.
更多查看译文
关键词
Single photon emission computed tomography,Imaging,Image segmentation,Image reconstruction,Kernel,Blood,Photonics,Cardiac SPECT,coronary microvascular disease,dynamic convolution,deep learning,intramyocardial blood volume,partial volume correction
AI 理解论文
溯源树
样例
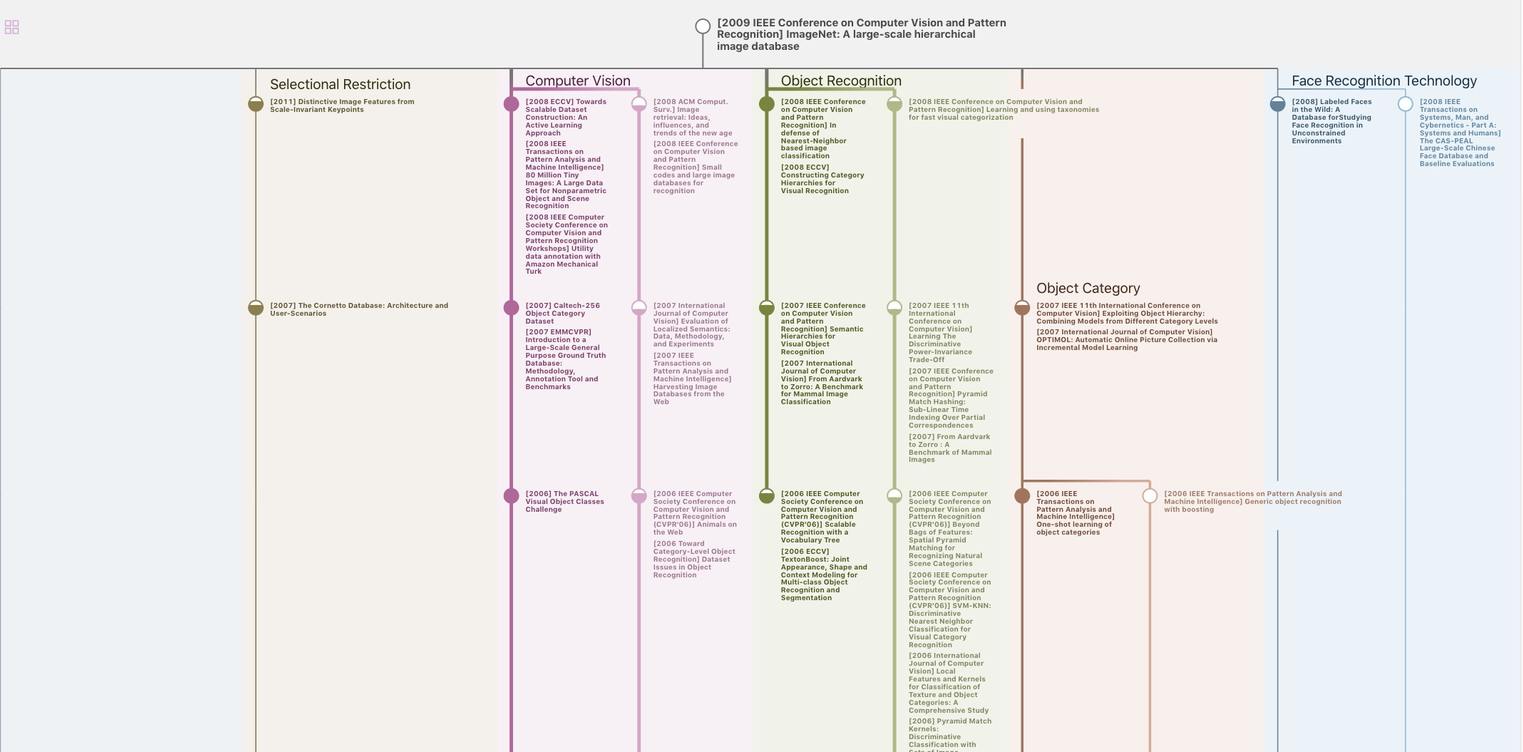
生成溯源树,研究论文发展脉络
Chat Paper
正在生成论文摘要