Fat-Free Mass and Body Fat in Patients with Myocardial Infarction Who Underwent Percutaneous Coronary Intervention
American Journal of Cardiology(2022)SCI 3区
Abstract
There are no data on the effects of fat-free mass (FFM) and body fat (BF) on prognosis in patients with myocardial infarction (MI). We investigated the effects of FFM and BF (which were estimated using formulas rather than direct measurements) on 30-day and long-term all-cause mortality in patients with MI who underwent percutaneous coronary intervention. We analyzed data from 6,453 patients with MI. The patients were divided into 2 categories (high/low) according to the fat-free mass index (FFMI) and 2 categories (low/high) according to the BF. The resultant 4 patient groups: High FFMI-Low BF, High FFMI-High BF, Low FFMI-Low BF, and Low FFMI-High BF, were compared. The lowest crude mortality after 30 days and in the long term was observed in the High FFMI-Low BF group (3.0%,9.8%, respectively), followed by the High FFMI-High BF group (6.6%, 27.0%, respectively), the Low FFMI-Low BF group (10.4%, 36.0%, respectively), and the Low FFMI-High BF group (14.7%, 56.8%, respectively). The difference was significant (p < 0.0001), as was the difference between groups. After adjustment, the FFMI-BF groups independently predicted 30-day mortality (p = 0.003), but the risk was similar in all groups. Compared with the High FFMI-Low BF group, the long-term mortality risk was similar in the High FFMI-High BF group (hazard ratio [HR] 1.11, 95% confidence interval [CI] 0.84 to 1.47, p = 0.47), but the Low FFMI-Low BF and Low FFMI-High BF patients had a higher risk (HR 1.59, 95% CI 1.20 to 2.11, p = 0.001, HR 1.40, 95% CI 1.03 to 1.91, p = 0.033, respectively). Body composition predicted mortality better than body mass index in patients with MI. Mortality appeared to be inversely related to FFM, with patients with low FFM and low BF having a particularly high mortality risk. The body composition groups also confirmed the obesity paradox. (C) 2022 The Author(s). Published by Elsevier Inc.
MoreTranslated text
求助PDF
上传PDF
View via Publisher
AI Read Science
AI Summary
AI Summary is the key point extracted automatically understanding the full text of the paper, including the background, methods, results, conclusions, icons and other key content, so that you can get the outline of the paper at a glance.
Example
Background
Key content
Introduction
Methods
Results
Related work
Fund
Key content
- Pretraining has recently greatly promoted the development of natural language processing (NLP)
- We show that M6 outperforms the baselines in multimodal downstream tasks, and the large M6 with 10 parameters can reach a better performance
- We propose a method called M6 that is able to process information of multiple modalities and perform both single-modal and cross-modal understanding and generation
- The model is scaled to large model with 10 billion parameters with sophisticated deployment, and the 10 -parameter M6-large is the largest pretrained model in Chinese
- Experimental results show that our proposed M6 outperforms the baseline in a number of downstream tasks concerning both single modality and multiple modalities We will continue the pretraining of extremely large models by increasing data to explore the limit of its performance
Upload PDF to Generate Summary
Must-Reading Tree
Example
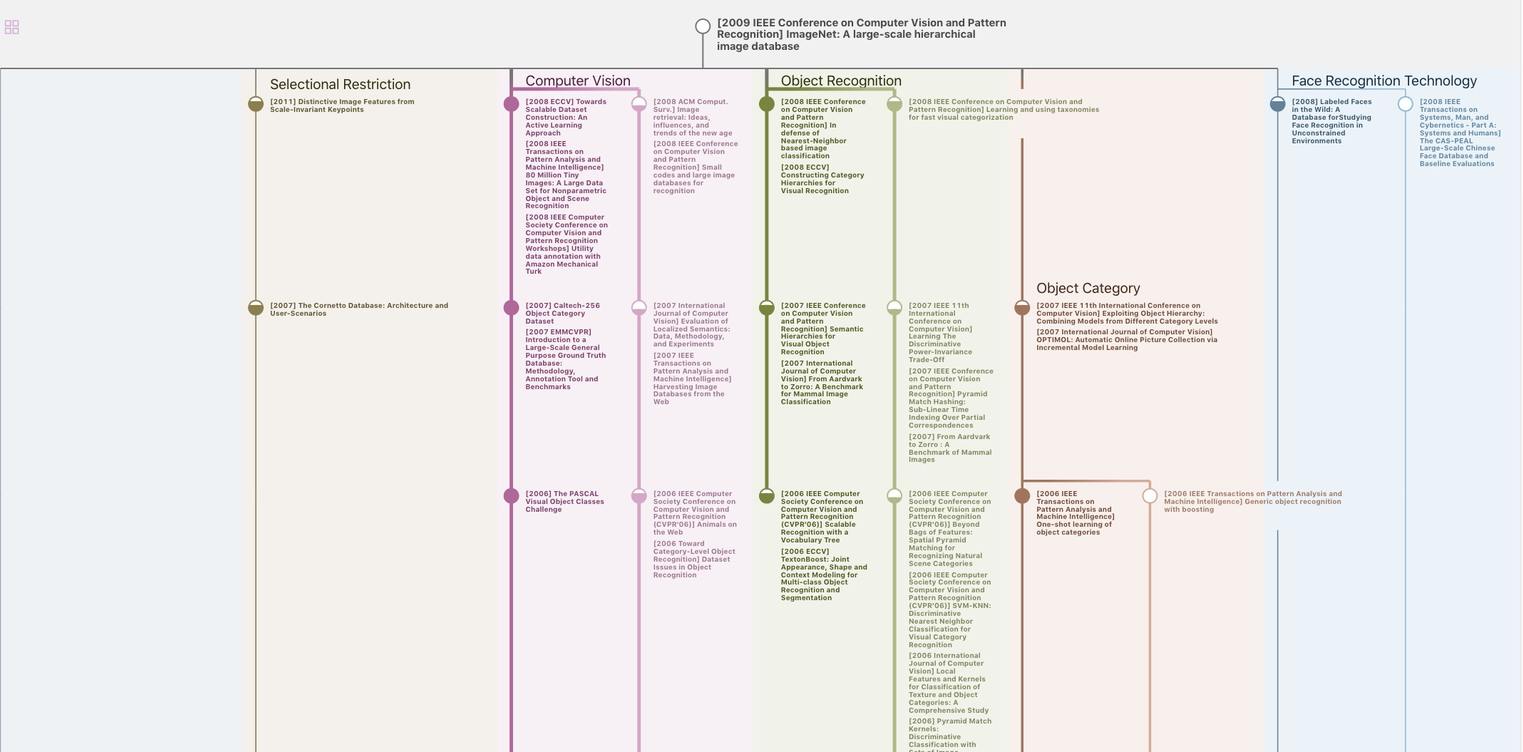
Generate MRT to find the research sequence of this paper
Related Papers
Sex Differences in the Relationship Between Body Mass Index and Outcome in Myocardial Infarction.
AMERICAN JOURNAL OF THE MEDICAL SCIENCES 2023
被引用1
FRONTIERS IN NUTRITION 2023
被引用0
Obesity and Acute Kidney Injury in Patients with ST-Elevation Myocardial Infarction
JOURNAL OF CLINICAL MEDICINE 2023
被引用0
Heart, Lung and Circulation 2024
被引用0
Data Disclaimer
The page data are from open Internet sources, cooperative publishers and automatic analysis results through AI technology. We do not make any commitments and guarantees for the validity, accuracy, correctness, reliability, completeness and timeliness of the page data. If you have any questions, please contact us by email: report@aminer.cn
Chat Paper