Propagation with Adaptive Mask then Training for Node Classification on Attributed Networks
arxiv(2022)
摘要
Node classification on attributed networks is a semi-supervised task that is crucial for network analysis. By decoupling two critical operations in Graph Convolutional Networks (GCNs), namely feature transformation and neighborhood aggregation, some recent works of decoupled GCNs could support the information to propagate deeper and achieve advanced performance. However, they follow the traditional structure-aware propagation strategy of GCNs, making it hard to capture the attribute correlation of nodes and sensitive to the structure noise described by edges whose two endpoints belong to different categories. To address these issues, we propose a new method called the itshape Propagation with Adaptive Mask then Training (PAMT). The key idea is to integrate the attribute similarity mask into the structure-aware propagation process. In this way, PAMT could preserve the attribute correlation of adjacent nodes during the propagation and effectively reduce the influence of structure noise. Moreover, we develop an iterative refinement mechanism to update the similarity mask during the training process for improving the training performance. Extensive experiments on four real-world datasets demonstrate the superior performance and robustness of PAMT.
更多查看译文
关键词
attributed networks,node classification,propagation,adaptive mask
AI 理解论文
溯源树
样例
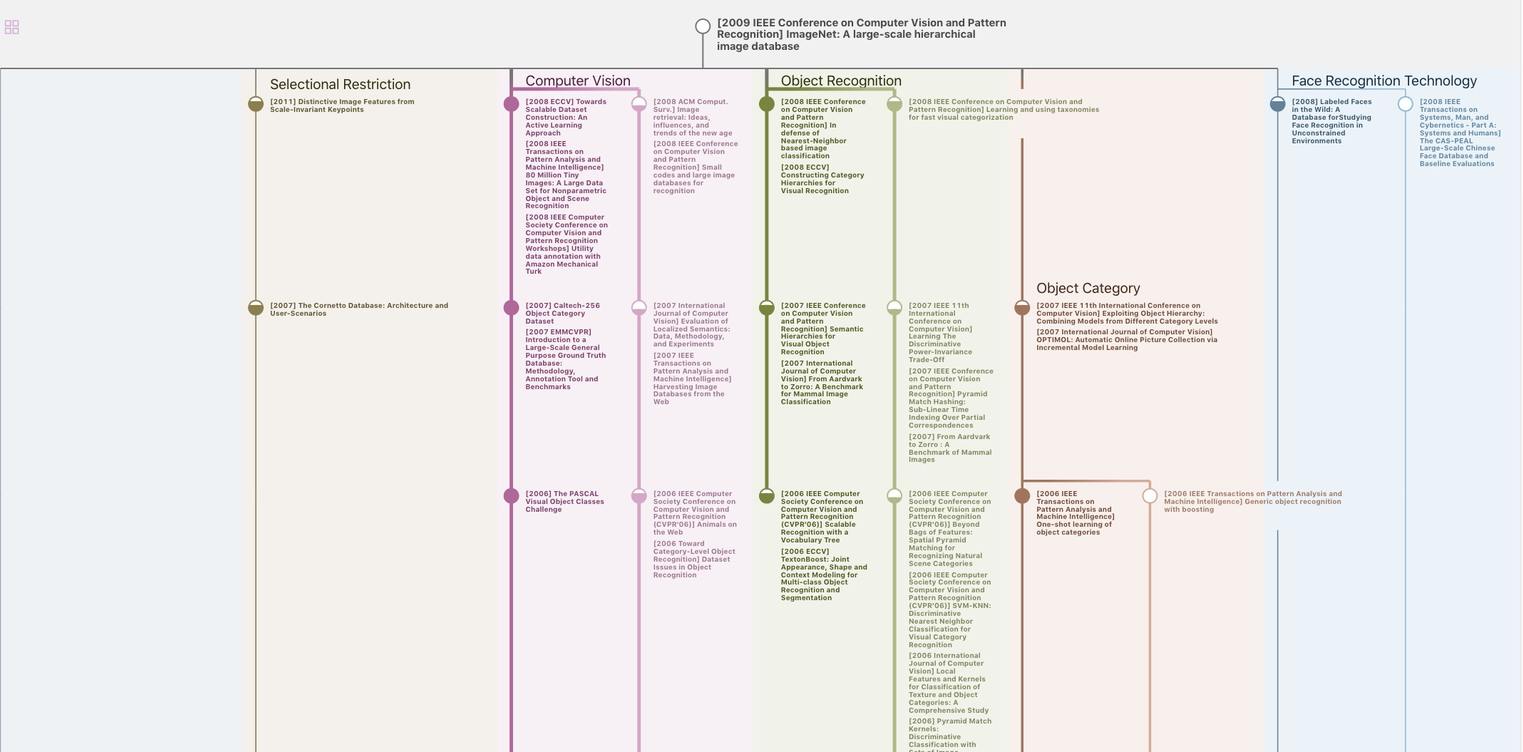
生成溯源树,研究论文发展脉络
Chat Paper
正在生成论文摘要