Power-Based Intrusion Detection for Additive Manufacturing: A Deep Learning Approach
Lecture Notes of the Institute for Computer Sciences, Social Informatics and Telecommunications EngineeringIndustrial IoT Technologies and Applications(2021)
摘要
Due to the ability of 3D-printers to build a wide range of objects at low costs, many industries are rapidly adopting additive manufacturing. However due to their sensing and communications capabilities, 3D-printers are Internet of Things (IoT) devices that are vulnerable to sophisticated cyberattacks, such as defect injection attacks. By maliciously manipulating the behavior of a 3D-printer, an attacker can compromise the integrity of a manufactured objects. To avoid detection, the adversary also compromises the sensor data reported by the 3D-printer that the operator could use to detect the attack. In this paper, we design a deep neural network that can detect such attacks by predicting the power consumption of a 3D-printer based on the object design and previous power consumption observations. By analyzing the difference between the predicted power consumption and the observed one, we can determine if the 3D-printer is under attack. By measuring the power consumption of the 3D-printer at the power line with an independent sensor, we can determine the true behavior of the 3D-printer without relying on sensor data reported by the potentially compromised 3D-printer. Compared to previous works, our proposed detection technique only requires cheap power sensors that can be easily installed. We conduct extensive experiments on a real-world additive manufacturing testbed and observe that our proposed method can detect defect injection attacks with up to 96% accuracy.
更多查看译文
关键词
Security,Intrusion detection,Side-channel defense,3D-printing,Additive manufacturing
AI 理解论文
溯源树
样例
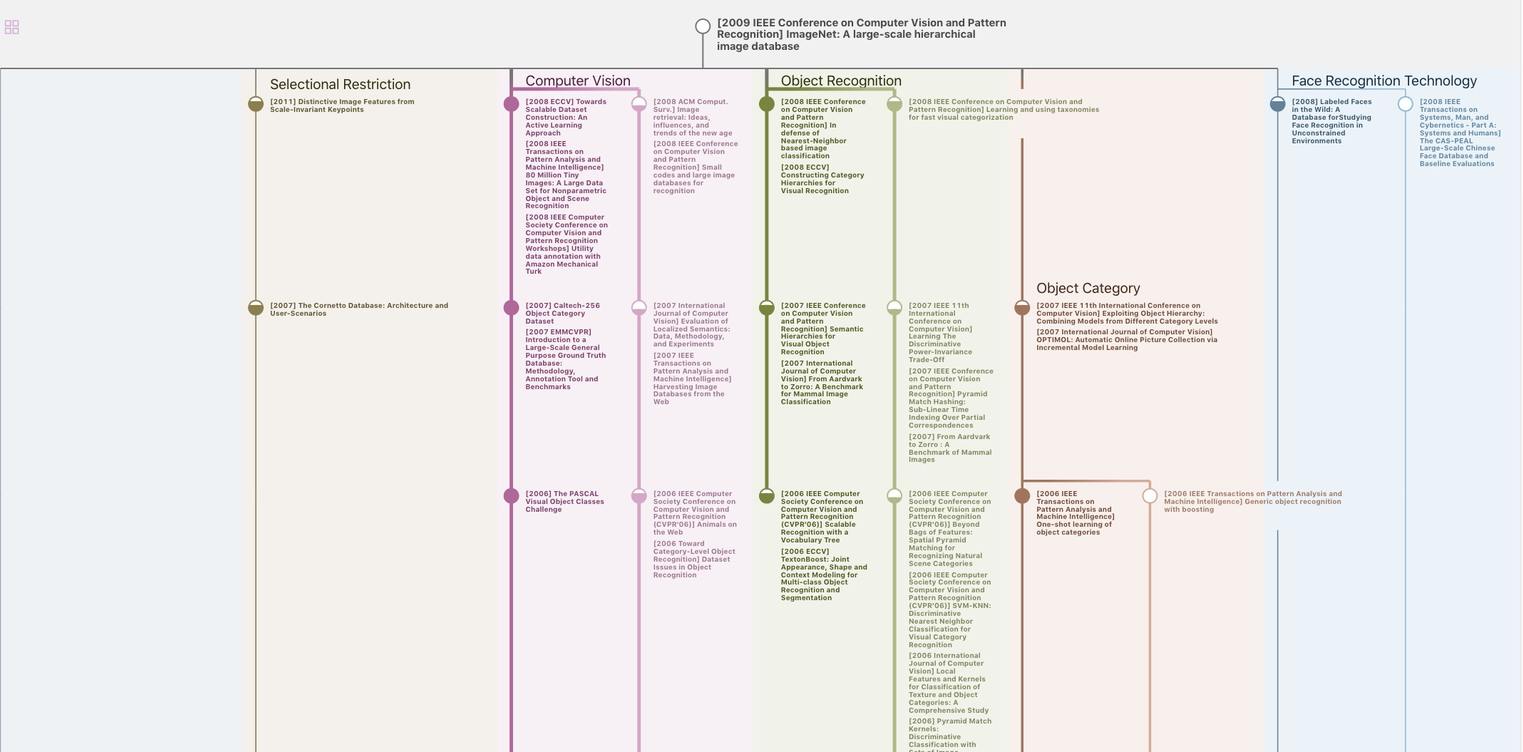
生成溯源树,研究论文发展脉络
Chat Paper
正在生成论文摘要