Hierarchical Residual Embedding Network for Real-Time De-focus Blur Detection
Journal of physics Conference series(2021)
摘要
Defocus blur detection, as an important pre-processing step of image processing, has attracted more and more attention. Albeit great success has been made, there are still several challenges for accurate defocus blur detection, such as the interference of background clutter, sensitivity to scales, missing boundary details and large computational burden. For handling these issues, we present a deep neural network which hierarchically embeds residual learning blocks for defocus blur detection. Based on the feature pyramid structure, we extract deep features with varying scales via utilizing a backbone fully convolutional network and generate a coarse score map by using the last layer of feature maps. Then we design a hierarchical residual embedding module to fuse different levels of features in a layer-wise manner. By embedding different layer-wise features in the top-down pathway, coarse-level semantic information from the deep layers can be seamlessly propagated to shallow layers, while fine details in the shallow layers can be used to refine the boundary between out-of-focus and in-focus regions. For each layer, a side output is generated by using a residual learning block. For capturing multi-scale information, the multiple side outputs of different layers are fed into a designed fusion block for yielding the final blur map result. Experimental results on two commonly used datasets show that our proposed network can more accurately locate the defocus blur regions with sharpened details being well preserved when compared to other previous state-of-the-arts. In addition, our approach is fast as well and can run at a speed of more than 25 FPS when processing an image with size 427 x 640.
更多查看译文
AI 理解论文
溯源树
样例
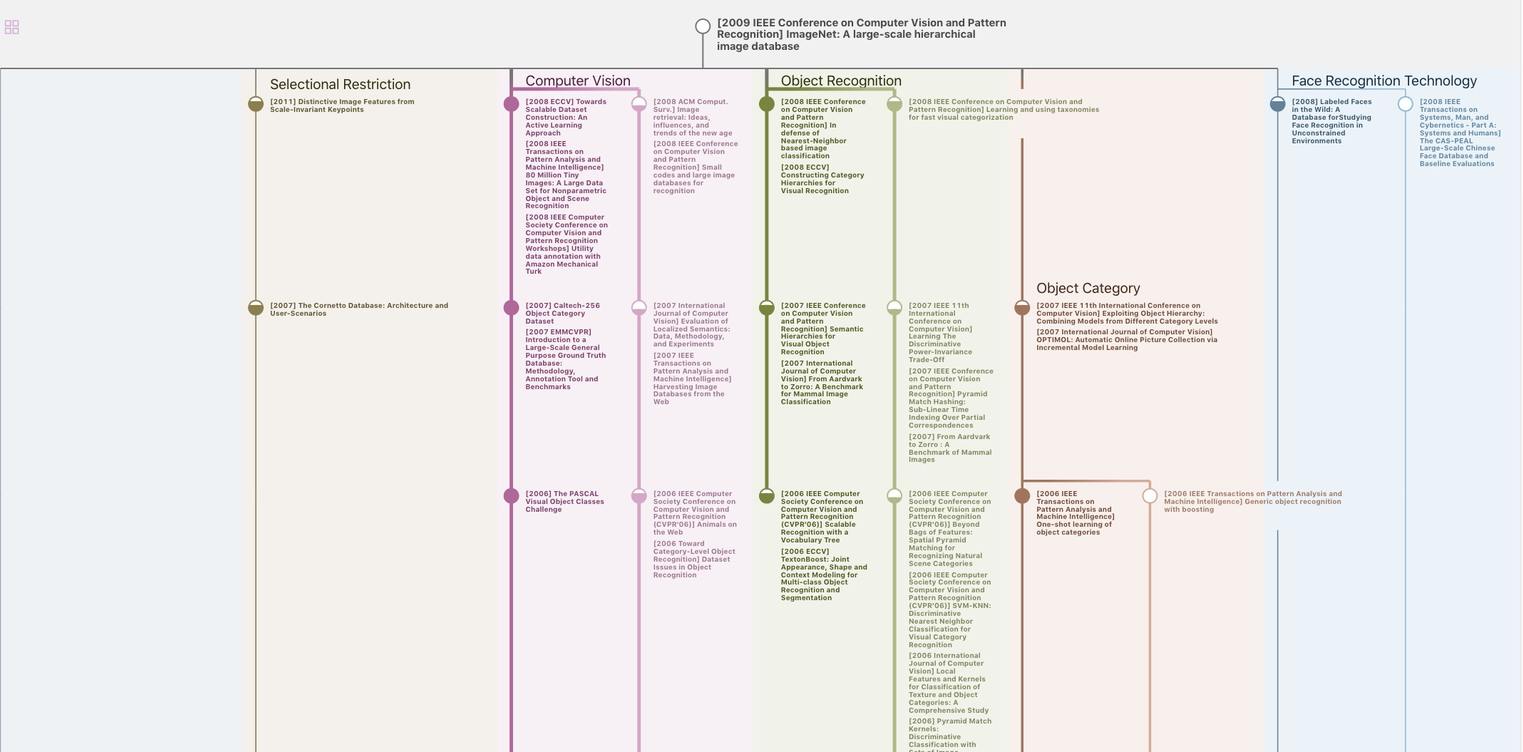
生成溯源树,研究论文发展脉络
Chat Paper
正在生成论文摘要