Quantitative Spatial Analysis on Whole Slide Images Using U-Net
Computational biology and bioinformatics(2020)
摘要
Advances in whole slide imaging technology have promoted a high use of digital slide images and generated a large volume of image data that is reliable and useful in determining treatment outcome. Recent technologies closely related to machine learning and deep learning algorithms have contributed to the success of digital histopathology by analyzing the digitized slide images providing quantitative information that are useful for faster turnaround times and effective treatment for the patient. The digital histopathological image analysis has received much attention due to its capability of mitigating the problem of the hand-crafted features. Features directly learned from raw data are trainable within the deep learning procedure and can be used for the histopathology image classification task. However, understanding the spatial context of cancer cells is still a challenging issue because of the heterogeneity of the tumor microenvironment which varies greatly, preventing successful diagnosis and leads to inappropriate therapeutic approaches for cancer patients. In this paper, we present a spatial analysis method for tumor microenvironment analysis using the U-Net architecture, a semantic segmentation deep-learning model, for a better understanding of the spatial relations between tissue types. We demonstrate the effectiveness of the U-Net architecture using a dataset created by an international crowdsourcing study. Moreover, we show that the quantitative estimates can be derived from the univariate spatial analysis.
更多查看译文
AI 理解论文
溯源树
样例
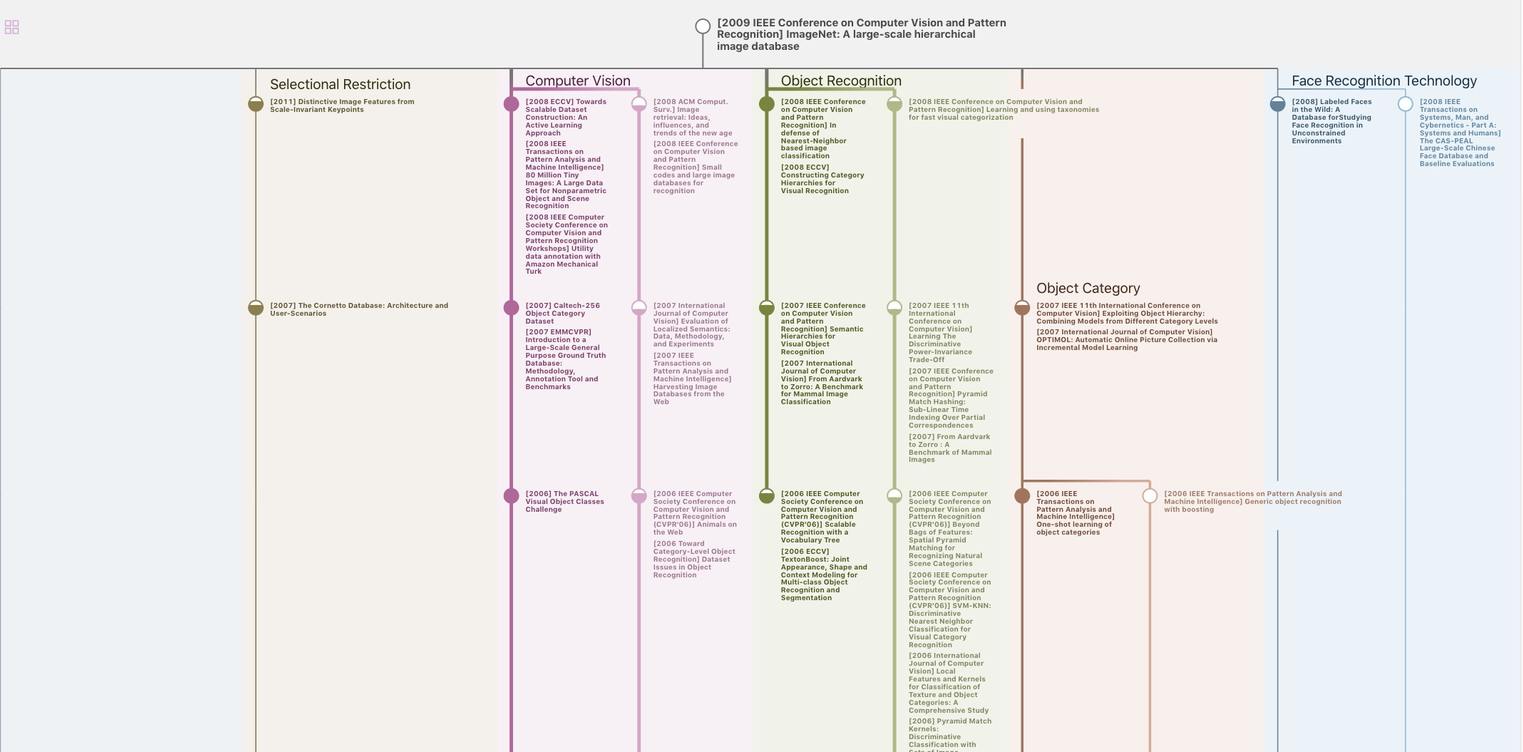
生成溯源树,研究论文发展脉络
Chat Paper
正在生成论文摘要