Transfer Learning with Related Tau PET Radioligands Improves 3D Neural Network Performance
Alzheimer's & Dementia(2021)
摘要
AbstractBackgroundNeural networks are increasingly being applied towards neuroimaging problems in AD, but performance is often limited by lack of adequate numbers of samples. This is commonly alleviated by pre‐training the model on a related task beforehand (i.e. ‘transfer learning’). To our knowledge, there exists no set/standard of widely available pretrained model weights for 3D medical images. Tau PET radiotracers such as AV‐1451 and MK‐6240 have highly similar binding patterns, and so may be well suited to transfer learning tasks.MethodSubjects with AV‐1451 scans (n=446) were downloaded from ADNI, while subjects with MK‐6240 scans (n= 320) were pooled from multiple studies recruiting at CUIMC. We trained multiple 3D neural networks (all with the same modified InceptionV3 architecture) to differentiate patients (i.e. MCI/AD) from controls based on their tau PET scan, both with and without pretraining information from prior training iterations of our models. We compare performance on holdout sets for each validation fold (n= 64 for MK‐6240 and n=89 for AV‐1451) using DeLong’s test for ROC curves and analyze features learned by models with t‐SNE plots.ResultThe AV‐1451 Model pretrained on MK‐6240 scans had superior performance to the AV‐1451 model not using pretraining (AUC = 0.74 vs. 0.91, Z = ‐5.5, p < 0.0001), whereas there was no significant difference with pretraining for MK‐6240 models (AUC = 0.84 vs. 0.83, Z = 0.47 ,p = 0.6). Visually, there appears to be greater separation in model learned features following pretraining in the AV‐1451 model.Conclusion3D pretraining for neural networks involving PET imaging could be accomplished with related but structurally different radioligands, though more work is needed into determining why certain pretraining experiments are successful. This procedure can enable better training of complex models with fewer constraints due to sample size.
更多查看译文
关键词
Imaging
AI 理解论文
溯源树
样例
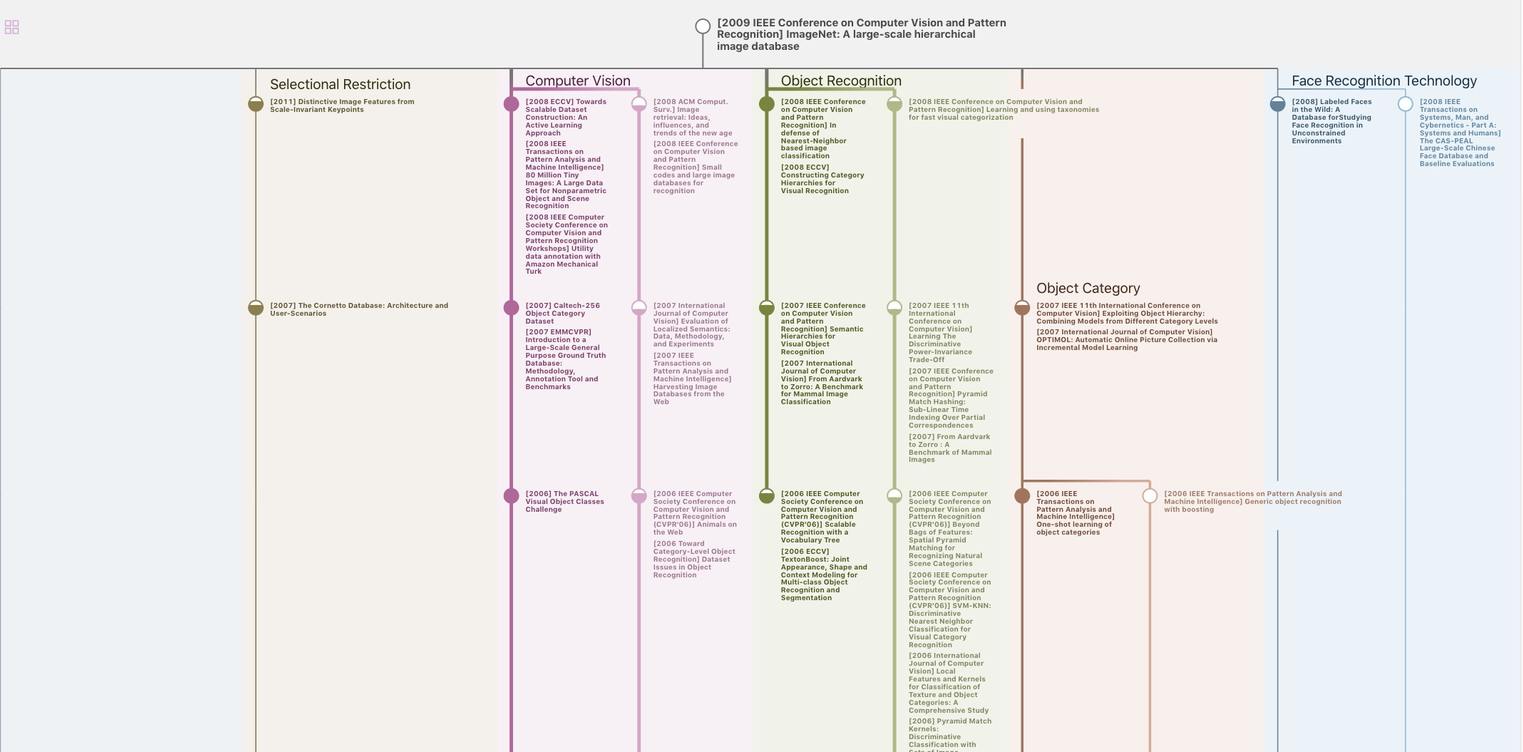
生成溯源树,研究论文发展脉络
Chat Paper
正在生成论文摘要