Machine Learning Based Classification System using Depth-Dependent Variation Encoding for Classifying Cervical Two-Photon Excited Fluorescence Image Stacks
Multimodal Image Exploitation and Learning 2022(2022)
摘要
The World Health Organization (WHO) called for a global fight against cervical cancer. There are an estimated 569,000 new cases and 310,000 deaths annually. Searching for practical approaches to deal with cervical cancer screening and treatment has been an urgent research subject. One solution could be to use label-free two-photon excited fluorescence (TPEF) imaging to address this need. The colposcopy-guided biopsy method is being used for cervical precancer detection relying primarily on morphological and organization cell and tissue feature changes. However, the overall performance of colposcopy and biopsy remains unsatisfactory. Label-free TPEF provides images with high morphological and functional (metabolic) content and could lead to enhanced detection of cervical pre-cancers. This paper uses the cell texture and morphology features to classify stacks of such TPEF images acquired from freshly excised healthy and pre-cancerous human cervical tissues. Herein, an automated denoising algorithm and a parametrized edge enhancement method is used for pre-processing the images in the stack. The computer simulations performed on the privately available dataset of 10 healthy stacks, 53 precancer stacks, and the recall and specificity of 100 %, respectively, were observed for both texture and morphology features. However, the dataset used to acquire these results is small. The presented model can be used as a base model for further research and analysis of a larger data set to identify early cervical cancerous changes and potentially significantly improve diagnosis and treatment.
更多查看译文
关键词
Cervical Cancer detection, Two-photon excited fluorescence image, machine learning, LBP, Fibonacci-p patterns, depth-dependent variation, Edge enhancement, denoising optimization
AI 理解论文
溯源树
样例
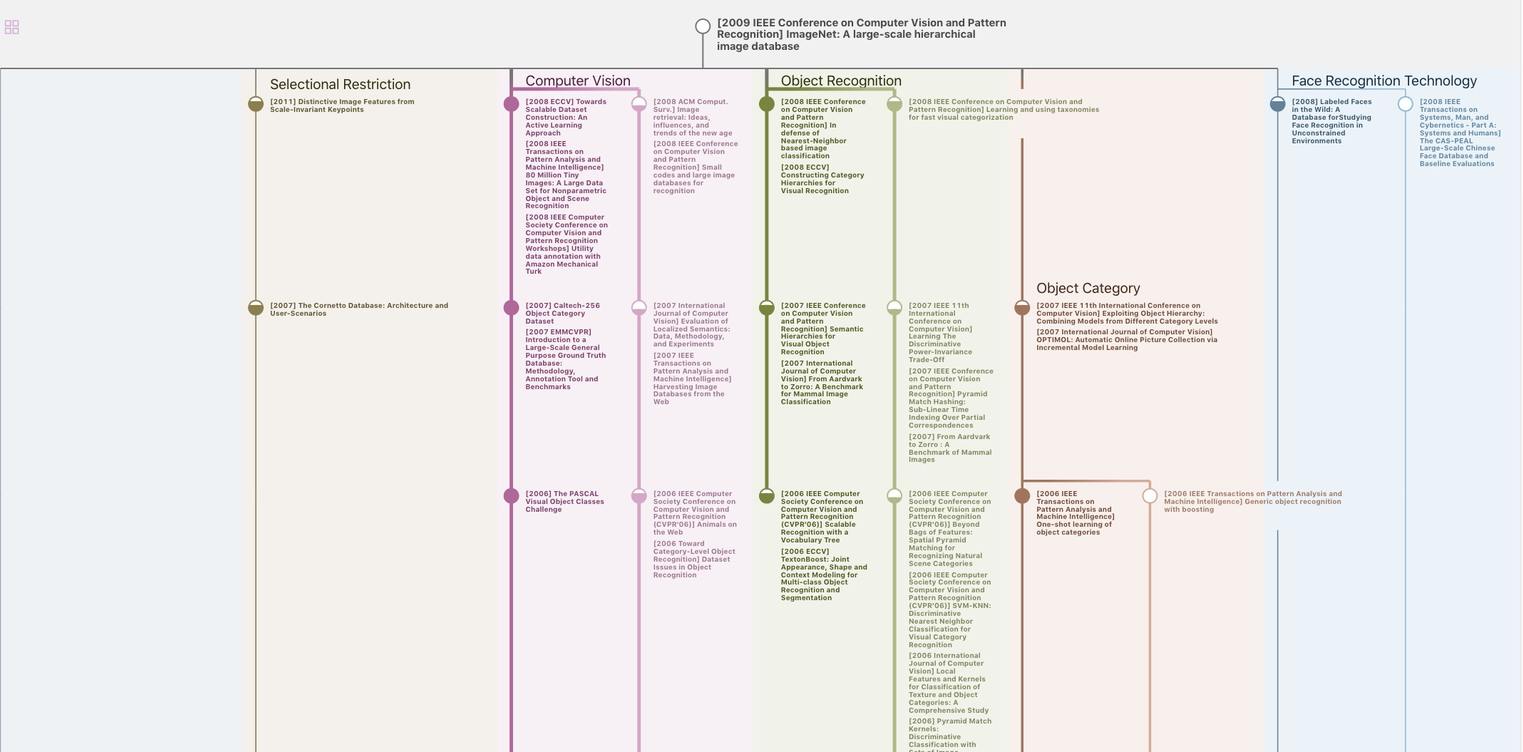
生成溯源树,研究论文发展脉络
Chat Paper
正在生成论文摘要