Dynamic selective Gaussian process regression for forecasting temperature of molten steel in ladle furnace
Engineering Applications of Artificial Intelligence(2022)
摘要
The requirement for intelligent steelmaking has underlined the significance of data-driven predictions of molten steel temperature in ladle furnace. Recently, predictors based on ensemble learning have shown their superiority over single ones. However, the strong reliability on the ensemble diversity can hardly insure their generalization ability. Moreover, most existing predictors cannot provide statistical meaning to their outputs. This has degraded their engineering value. In this paper, we aim to address these two problems in one scheme, where a dynamic regression ensemble of Gaussian process models is built. Our dynamic ensemble will select the most competent individual for each test pattern according to the competence estimated by informative neighbors. To this end, a distance measure based on RReliefF is constructed to search for these neighbors, rather than traditional K-nearest neighbor. Several evaluation indexes are combined by a meta regressor so that more robust estimation of competence can be achieved. A Bayesian nonparametric model is used for ensemble generation in order to obtain statistical predictions. A data set from real-world ladle furnace is used to verify the effectiveness of the proposed predictor. According to the comparative results, we have found the superiority of our dynamic ensemble over static ensembles and single predictors. Furthermore, the improvement over existing dynamic ensembles has also been confirmed.
更多查看译文
关键词
Molten steel temperature prediction,Gaussian process regression,Dynamic ensemble,Ladle furnace
AI 理解论文
溯源树
样例
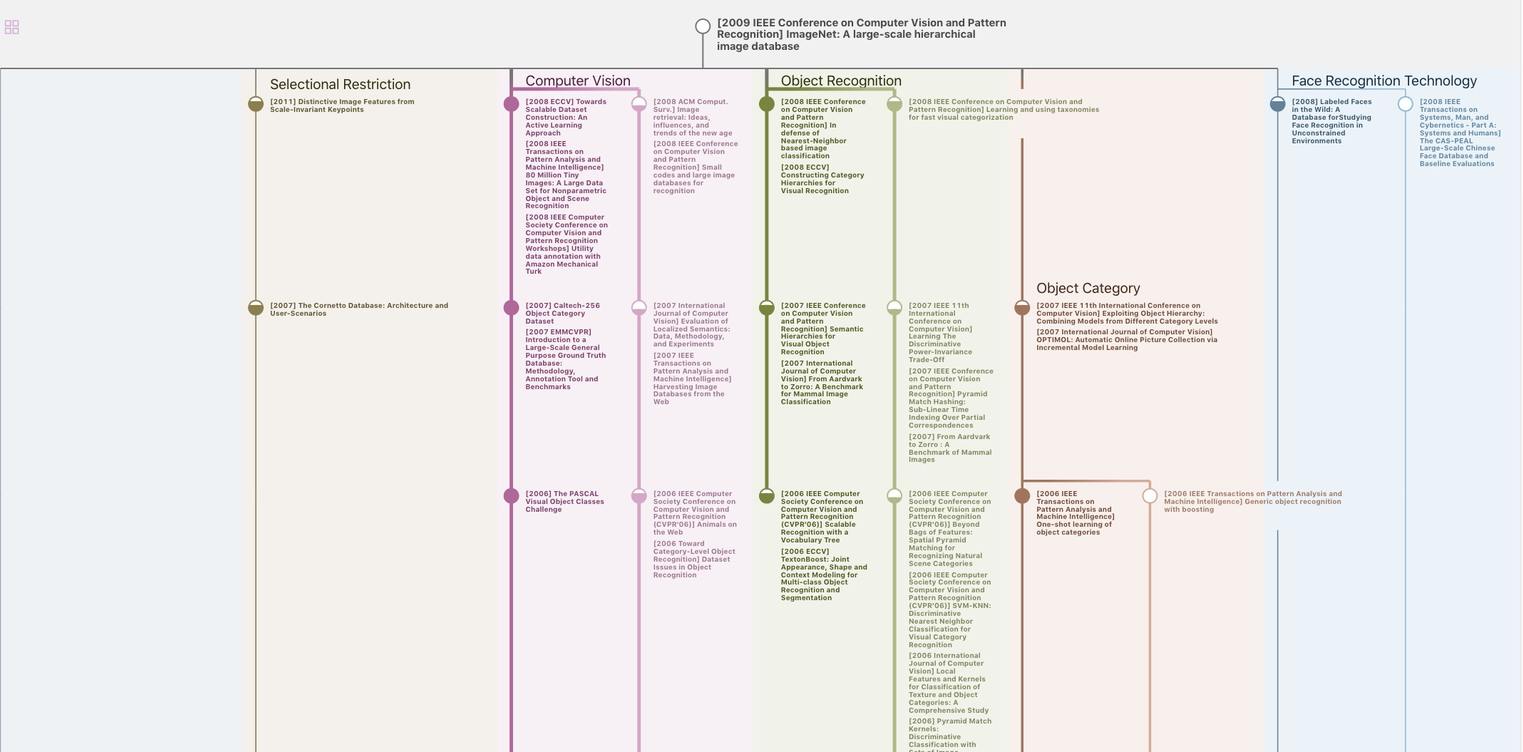
生成溯源树,研究论文发展脉络
Chat Paper
正在生成论文摘要