Learning 3D Object Shape and Layout without 3D Supervision
IEEE Conference on Computer Vision and Pattern Recognition(2022)
摘要
A 3D scene consists of a set of objects, each with a shape and a layout giving their position in space. Understanding 3D scenes from 2D images is an important goal, with ap-plications in robotics and graphics. While there have been recent advances in predicting 3D shape and layout from a single image, most approaches rely on 3D ground truth for training which is expensive to collect at scale. We overcome these limitations and propose a method that learns to predict 3D shape and layout for objects without any ground truth shape or layout information: instead we rely on multi-view images with 2D supervision which can more easily be col-lected at scale. Through extensive experiments on ShapeNet, Hypersim, and ScanNet we demonstrate that our approach scales to large datasets of realistic images, and compares favorably to methods relying on 3D ground truth. On Hy-persim and ScanNet where reliable 3D ground truth is not available, our approach outperforms supervised approaches trained on smaller and less diverse datasets. 1 1 Project page https://gkioxari.github.io/usl/
更多查看译文
关键词
3D from single images, Recognition: detection,categorization,retrieval, Scene analysis and understanding, Segmentation,grouping and shape analysis
AI 理解论文
溯源树
样例
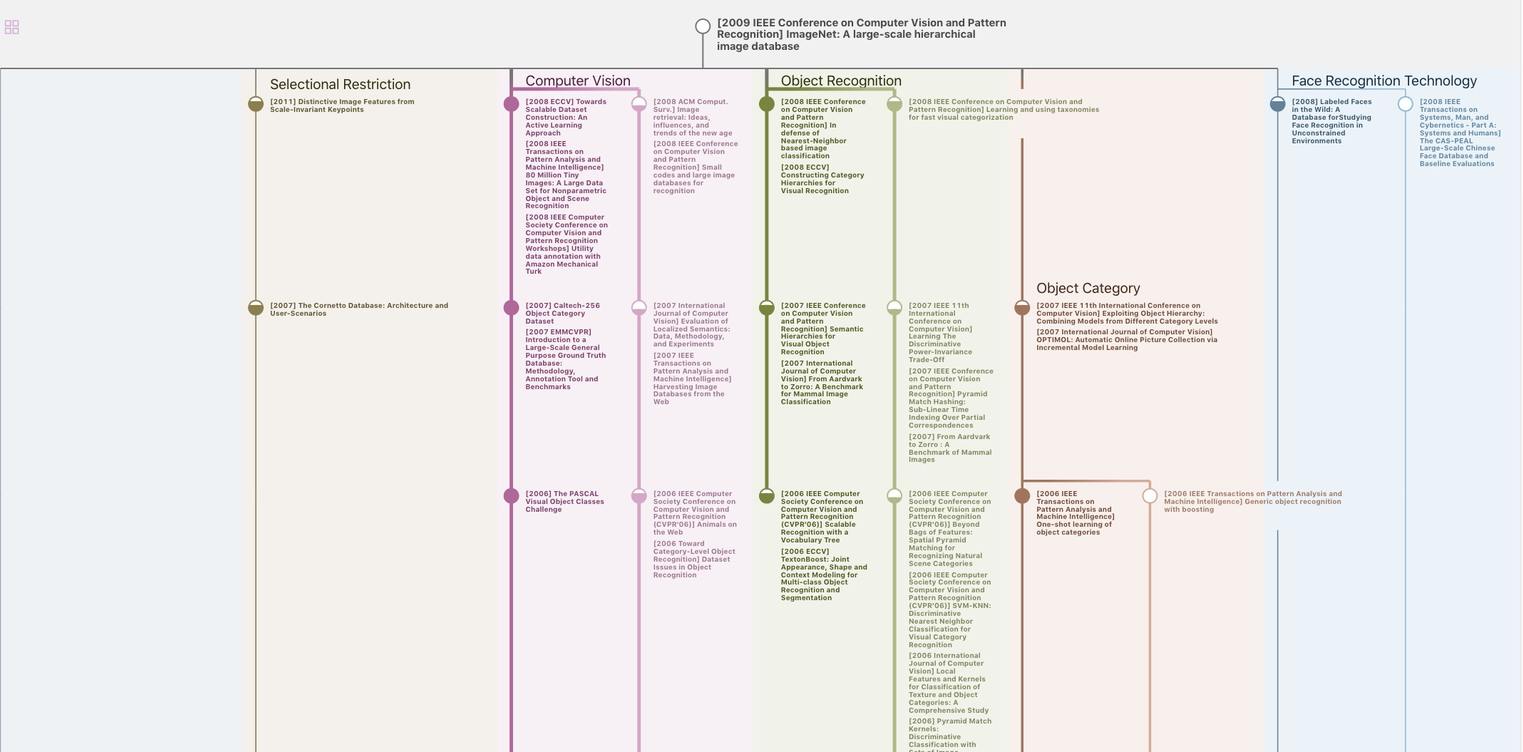
生成溯源树,研究论文发展脉络
Chat Paper
正在生成论文摘要