ProcTHOR: Large-Scale Embodied AI Using Procedural Generation
arxiv(2022)
摘要
Massive datasets and high-capacity models have driven many recent advancements in computer vision and natural language understanding. This work presents a platform to enable similar success stories in Embodied AI. We propose ProcTHOR, a framework for procedural generation of Embodied AI environments. ProcTHOR enables us to sample arbitrarily large datasets of diverse, interactive, customizable, and performant virtual environments to train and evaluate embodied agents across navigation, interaction, and manipulation tasks. We demonstrate the power and potential of ProcTHOR via a sample of 10,000 generated houses and a simple neural model. Models trained using only RGB images on ProcTHOR, with no explicit mapping and no human task supervision produce state-of-the-art results across 6 embodied AI benchmarks for navigation, rearrangement, and arm manipulation, including the presently running Habitat 2022, AI2-THOR Rearrangement 2022, and RoboTHOR challenges. We also demonstrate strong 0-shot results on these benchmarks, via pre-training on ProcTHOR with no fine-tuning on the downstream benchmark, often beating previous state-of-the-art systems that access the downstream training data.
更多查看译文
关键词
Embodied AI,Large-Scale Environments,Procedural Generation
AI 理解论文
溯源树
样例
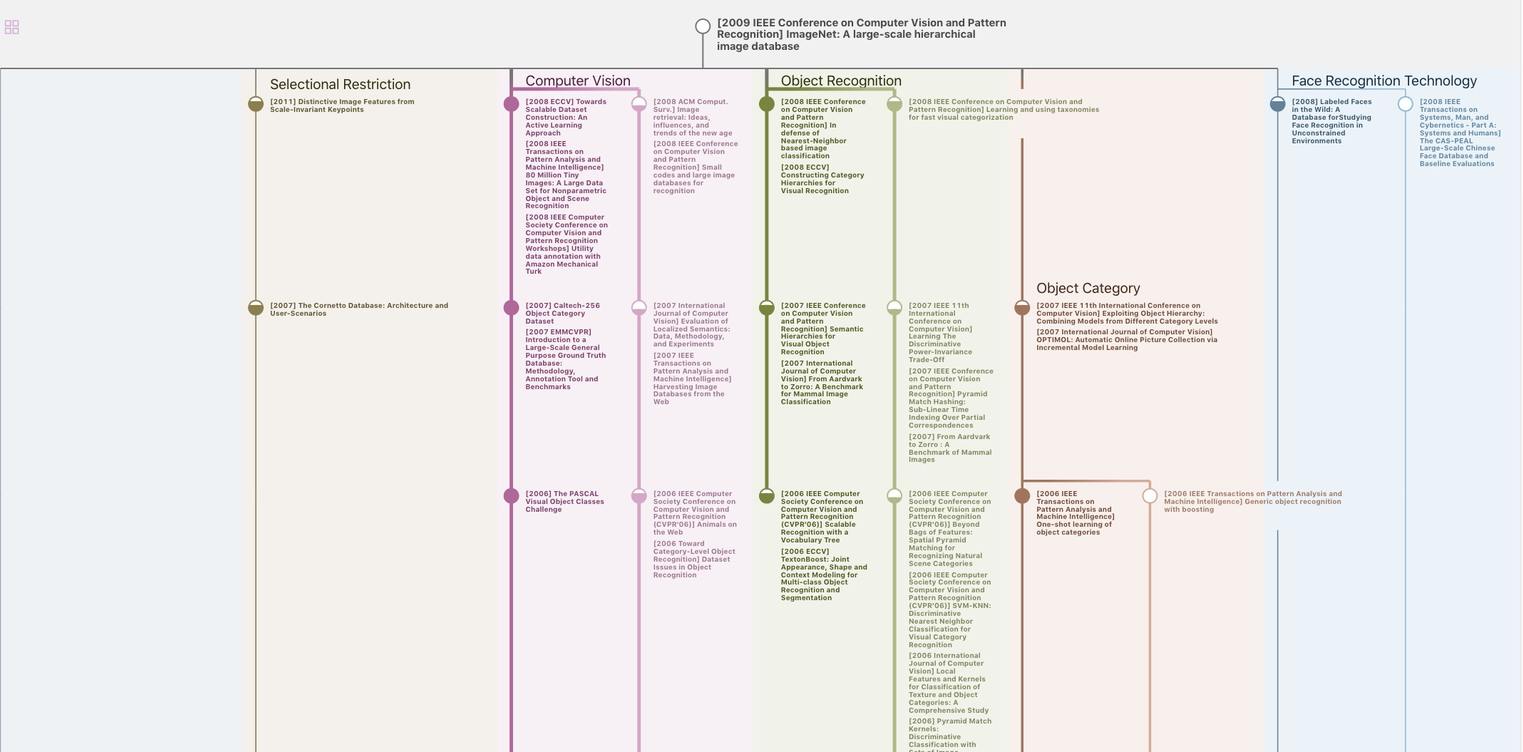
生成溯源树,研究论文发展脉络
Chat Paper
正在生成论文摘要