Computational Prediction of N- and O-Linked Glycosylation Sites for Human and Mouse Proteins.
Methods in molecular biology (Clifton, N.J.)(2022)
摘要
Protein glycosylation is one of the most complex posttranslational modifications (PTM) that play a fundamental role in protein function. Identification and annotation of these sites using experimental approaches are challenging and time consuming. Hence, there is a demand to build fast and efficient computational methods to address this problem. Here, we present the SPRINT-Gly framework containing the largest dataset and a prediction model of glycosylation sites for a given protein sequence. In this framework, we construct a large dataset containing N- and O-linked glycosylation sites of human and mouse proteins, collected from different sources. We then introduce the SPRINT-Gly method to predict putative N- and O-linked sites. SPRINT-Gly is a machine learning-based approach consisting of a number of trained predictive models for glycosylation sites in both human and mouse proteins, separately. The method is built by incorporating sequence-based, predicted structural, and physicochemical information of the neighboring residues of each N- and O-linked glycosylation site and by training deep learning neural network and support vector machine as classifiers. SPRINT-Gly outperformed other existing methods by achieving 18% and 50% higher Matthew's correlation coefficient for N- and O-linked glycosylation site prediction, respectively. SPRINT-Gly is publicly available as an online and stand-alone predictor at https://sparks-lab.org/server/sprint-gly/ .
更多查看译文
关键词
Deep learning,Glycosylation,Glycosylation sites prediction,Machine learning,N- and O-linked glycosylation sites,Posttranslational modifications
AI 理解论文
溯源树
样例
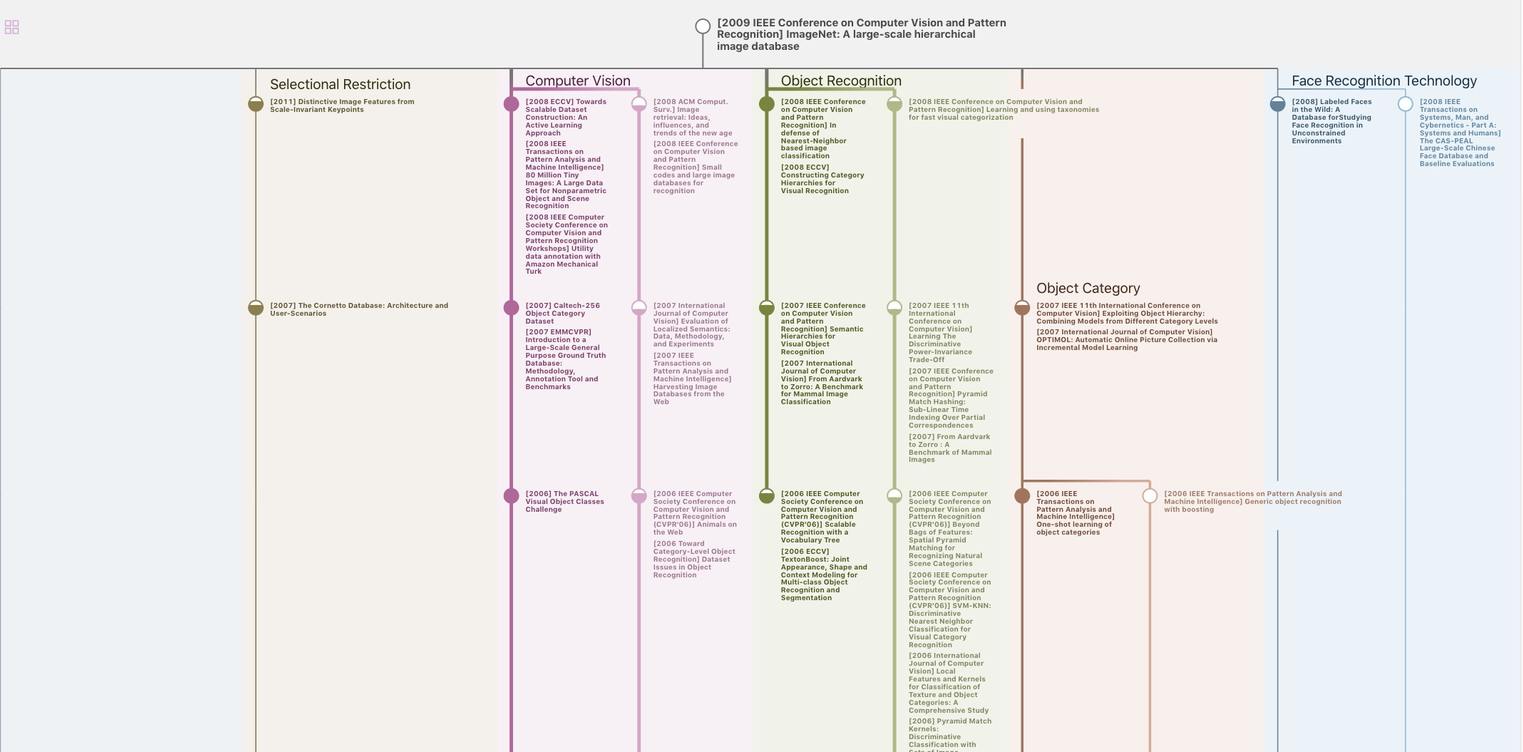
生成溯源树,研究论文发展脉络
Chat Paper
正在生成论文摘要