Graph Neural Networks Intersect Probabilistic Graphical Models: A Survey
arxiv(2023)
摘要
Graphs are a powerful data structure to represent relational data and are widely used to describe complex real-world data structures. Probabilistic Graphical Models (PGMs) have been well-developed in the past years to mathematically model real-world scenarios in compact graphical representations of distributions of variables. Graph Neural Networks (GNNs) are new inference methods developed in recent years and are attracting growing attention due to their effectiveness and flexibility in solving inference and learning problems over graph-structured data. These two powerful approaches have different advantages in capturing relations from observations and how they conduct message passing, and they can benefit each other in various tasks. In this survey, we broadly study the intersection of GNNs and PGMs. Specifically, we first discuss how GNNs can benefit from learning structured representations in PGMs, generate explainable predictions by PGMs, and how PGMs can infer object relationships. Then we discuss how GNNs are implemented in PGMs for more efficient inference and structure learning. In the end, we summarize the benchmark datasets used in recent studies and discuss promising future directions.
更多查看译文
关键词
graph,models,neural networks
AI 理解论文
溯源树
样例
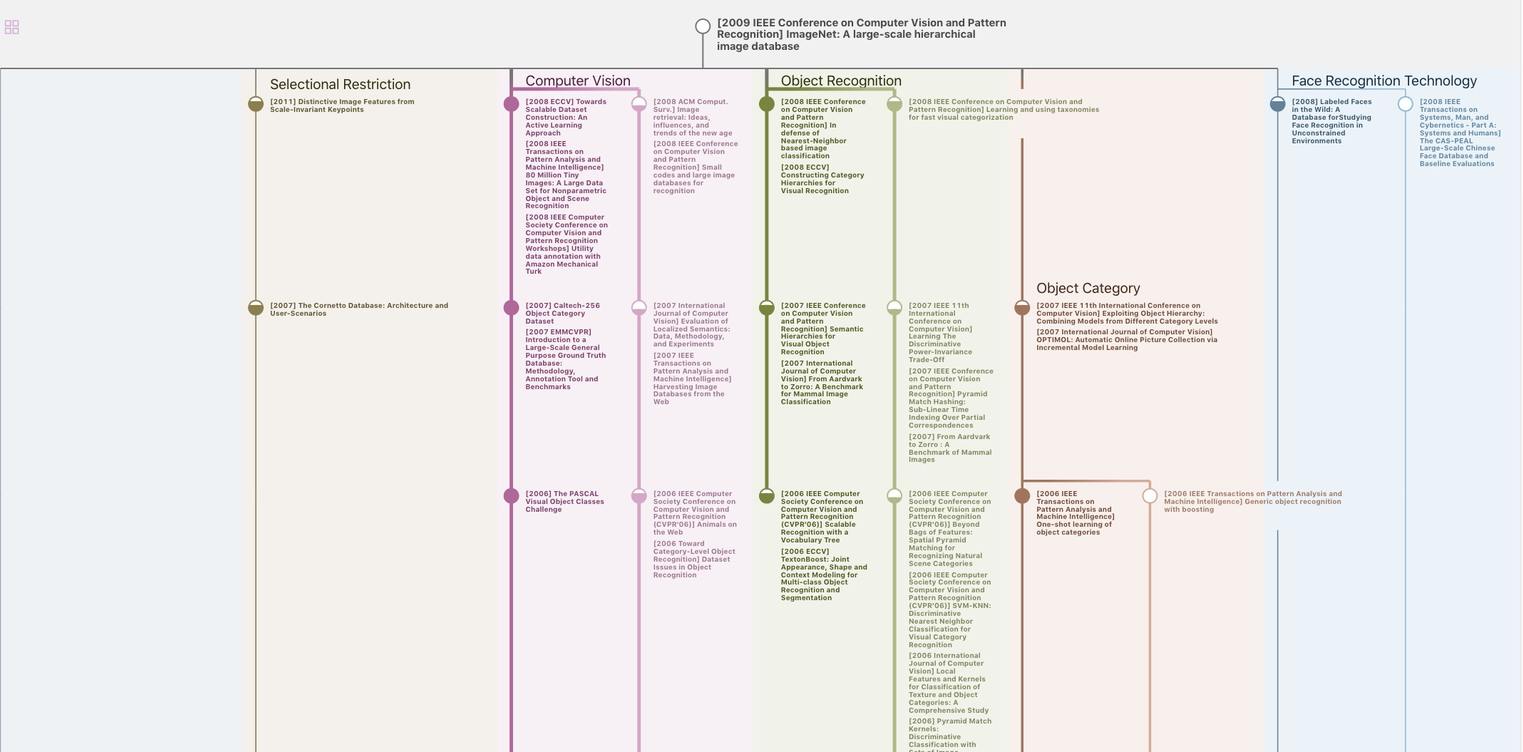
生成溯源树,研究论文发展脉络
Chat Paper
正在生成论文摘要