LinK3D: Linear Keypoints Representation for 3D LiDAR Point Cloud
IEEE ROBOTICS AND AUTOMATION LETTERS(2024)
摘要
Feature extraction and matching are the basic parts of many robotic vision tasks, such as 2D or 3D object detection, recognition, and registration. As is known, 2D feature extraction and matching have already achieved great success. Unfortunately, in the field of 3D, the current methods may fail to support the extensive application of 3D LiDAR sensors in robotic vision tasks due to their poor descriptiveness and inefficiency. To address this limitation, we propose a novel 3D feature representation method: Lin ear K eypoints representation for 3D LiDAR point cloud, called LinK3D. The novelty of LinK3D lies in that it fully considers the characteristics (such as the sparsity and complexity) of LiDAR point clouds and represents the keypoint with its robust neighbor keypoints, which provide strong constraints in the description of the keypoint. The proposed LinK3D has been evaluated on three public datasets, and the experimental results show that our method achieves great matching performance. More importantly, LinK3D also shows excellent real-time performance, faster than the sensor frame rate at 10 Hz of a typical rotating LiDAR sensor. LinK3D only takes an average of 30 milliseconds to extract features from the point cloud collected by a 64-beam LiDAR and takes merely about 20 milliseconds to match two LiDAR scans when executed on a computer with an Intel Core i7 processor. Moreover, our method can be extended to LiDAR odometry task, and shows good scalability.
更多查看译文
关键词
Laser radar,Feature extraction,Three-dimensional displays,Point cloud compression,Task analysis,Histograms,Simultaneous localization and mapping,3D LiDAR point cloud,feature extraction and matching,real-time,LiDAR SLAM
AI 理解论文
溯源树
样例
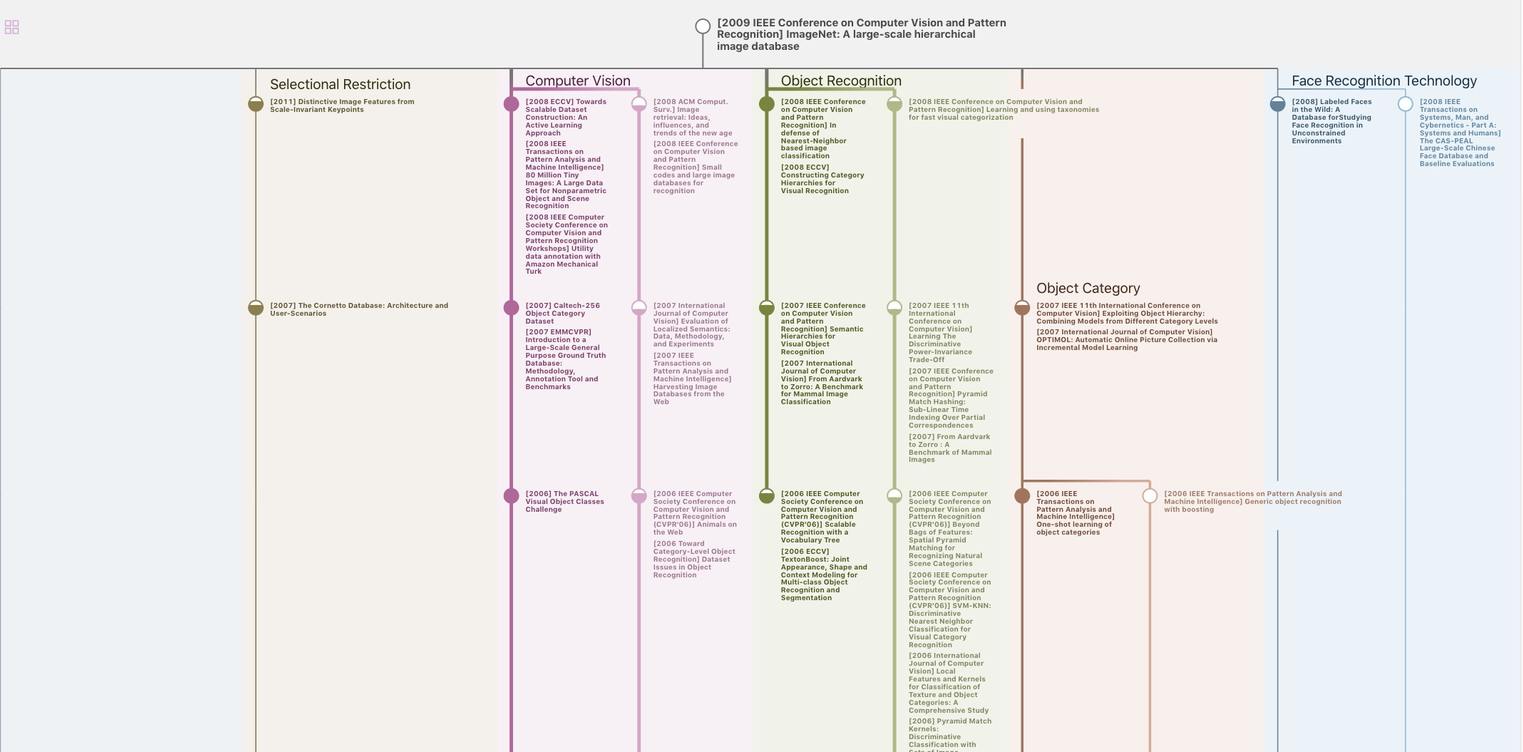
生成溯源树,研究论文发展脉络
Chat Paper
正在生成论文摘要