Measuring the Availability of Human Resources for Health and Its Relationship to Universal Health Coverage for 204 Countries and Territories from 1990 to 2019: a Systematic Analysis for the Global Burden of Disease Study 2019
The Lancet(2022)
Univ Washington | Philippine Inst Dev Studies | Harvard Univ | Univ Coll Hosp | McMaster Univ | Mayo Clin Fdn Med Educ & Res | Washington Univ | Murdoch Univ | Imam Abdulrahman Bin Faisal Univ | Natl Inst Publ Hlth | Iran Univ Med Sci | Univ Coast | Ateneo Manila Univ | Carol Davila Univ Med & Pharm | Bucharest Univ Econ Studies | Univ Philippines Manila | Birmingham City Univ | Univ Hlth & Allied Sci | Med Univ Lodz | Shiraz Univ Med Sci | NYU | Augusta Univ | Babol Univ Med Sci | Mario Negri Inst Pharmacol Res | Univ Catania | Manipal Acad Higher Educ | Tech Univ Berlin | Univ Waterloo | Hosp Italian Buenos Aires | Metropolitan Autonomous Univ | Univ Porto | Heidelberg Univ | Texila Amer Univ | Datta Meghe Inst Med Sci | VNU Int Sch | Mazandaran Univ Med Sci | Nepal Hlth Res Council | Univ Ibadan | Minist Hlth & Med Educ | Inst Hlth Econ & Technol | Univ Southern Calif | Tabriz Univ Med Sci | Natl Inst Hlth Res | Alexandria Univ | Charite Med Univ | Natl Acad Med Sci Ukraine | KA Timiryazev Inst Plant Physiol | Abadan Univ Med Sci | Kobe Univ | Cleveland Clin | Kermanshah Univ Med Sci | Qazvin Univ Med Sci | Hokkaido Univ | Univ South Carolina | Arabian Gulf Univ | Hamdan Bin Mohammed Smart Univ | Univ Barcelona | Maragheh Univ Med Sci | Cairo Univ | Univ Oulu | Univ Oxford | Univ Texas Austin | Bangladesh Ind Gases | Hamad Bin Khalifa Univ | Lorestan Univ Med Sci | Deakin Univ | All India Inst Med Sci | Int Res Ctr Excellence | Univ Management & Technol | Sheffield Hallam Univ | Kristiania Univ Coll | Panjab Univ | Univ Montreal | Publ Hlth Fdn India | Univ Baghdad | Uppsala Univ | Nova Southeastern Univ | Univ Malaya | Tribhuvan Univ | Imperial Coll London | Univ Tehran Med Sci | Natl Inst Mental Hlth & Neurosci | Ananthapuri Hosp & Res Inst | Kyrgyz State Med Acad | King Saud Univ | South African Med Res Council | Univ Embu | Duy Tan Univ | Ctr Addict & Mental Hlth | Univ Nigeria Nsukka | Moscow Inst Phys & Technol | Nguyen Tat Thanh Univ | Integrated Dev Fdn Nepal | Amirkabir Univ Technol | Macquarie Univ | CQ Univ | Western Sydney Univ | Univ New South Wales | Universal Sci Educ & Res Network | Univ Teaching Hosp Kigali | Ain Shams Univ | Univ Fed Uberlandia | Univ Belgrade | Stellenbosch Univ | Manian Med Ctr | Univ Fed Santa Catarina | Russian Acad Sci | James Cook Univ | Univ Sao Paulo | Univ Agr Faisalabad | Argentine Soc Med | UKK Inst | Raffles Hosp | Natl Res Univ Higher Sch Econ | Gates Ventures | Natl Ctr Neurol & Psychiat | Jackson State Univ | Wuhan Univ | Duke Univ | Monash Univ | Univ Edinburgh
- Pretraining has recently greatly promoted the development of natural language processing (NLP)
- We show that M6 outperforms the baselines in multimodal downstream tasks, and the large M6 with 10 parameters can reach a better performance
- We propose a method called M6 that is able to process information of multiple modalities and perform both single-modal and cross-modal understanding and generation
- The model is scaled to large model with 10 billion parameters with sophisticated deployment, and the 10 -parameter M6-large is the largest pretrained model in Chinese
- Experimental results show that our proposed M6 outperforms the baseline in a number of downstream tasks concerning both single modality and multiple modalities We will continue the pretraining of extremely large models by increasing data to explore the limit of its performance
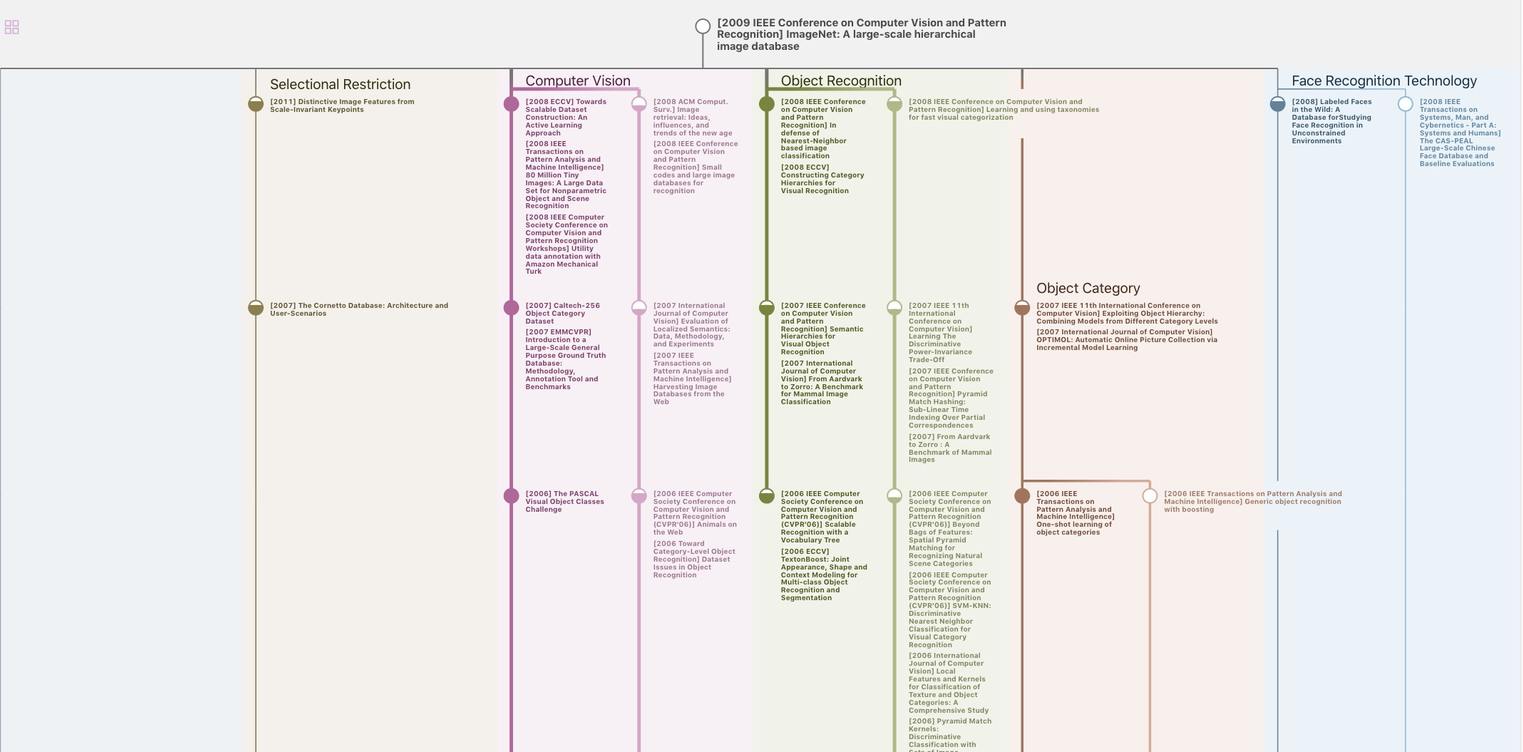
被引用18 | 浏览
被引用139 | 浏览
被引用71 | 浏览
被引用63 | 浏览
被引用25 | 浏览
被引用53 | 浏览
被引用176 | 浏览
被引用39 | 浏览
被引用339 | 浏览
被引用76 | 浏览
被引用44 | 浏览
被引用204 | 浏览