Mitigating pseudoreplication and bias in resource selection functions with autocorrelation-informed weighting
METHODS IN ECOLOGY AND EVOLUTION(2023)
摘要
Resource selection functions (RSFs) are among the most commonly used statistical tools in both basic and applied animal ecology. They are typically parameterized using animal tracking data, and advances in animal tracking technology have led to increasing levels of autocorrelation between locations in such data sets. Because RSFs assume that data are independent and identically distributed, such autocorrelation can cause misleadingly narrow confidence intervals and biased parameter estimates. Data thinning, generalized estimating equations and step selection functions (SSFs) have been suggested as techniques for mitigating the statistical problems posed by autocorrelation, but these approaches have notable limitations that include statistical inefficiency, unclear or arbitrary targets for adequate levels of statistical independence, constraints in input data and (in the case of SSFs) scale-dependent inference. To remedy these problems, we introduce a method for likelihood weighting of animal locations to mitigate the negative consequences of autocorrelation on RSFs. In this study, we demonstrate that this method weights each observed location in an animal's movement track according to its level of non-independence, expanding confidence intervals and reducing bias that can arise when there are missing data in the movement track. Ecologists and conservation biologists can use this method to improve the quality of inferences derived from RSFs. We also provide a complete, annotated analytical workflow to help new users apply our method to their own animal tracking data using the ctmm R package.
更多查看译文
关键词
continuous-time movement models,habitat selection,home range,Ornstein-Uhlenbeck process,space use,spatial point process,stochastic process model,utilization distribution
AI 理解论文
溯源树
样例
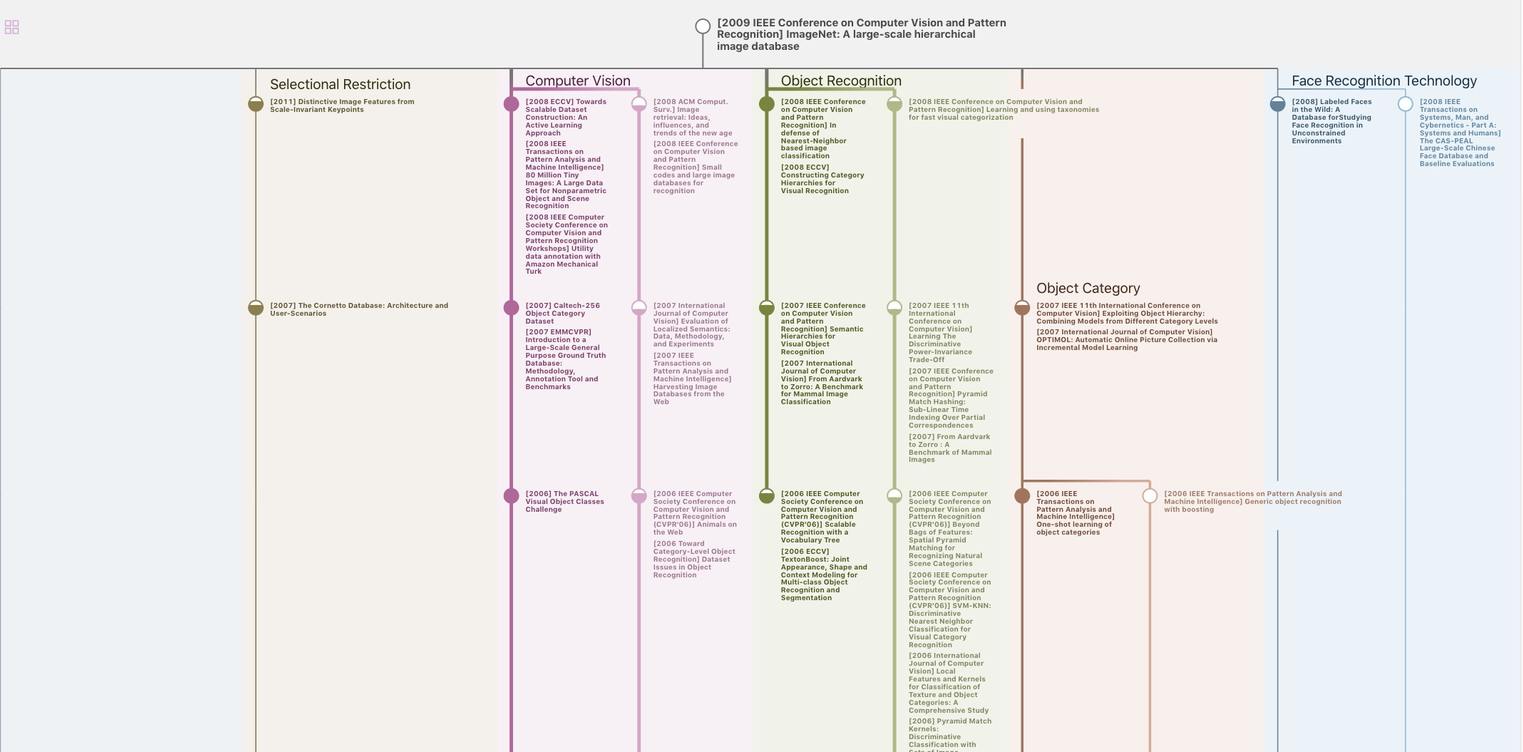
生成溯源树,研究论文发展脉络
Chat Paper
正在生成论文摘要