NeuPSL: neural probabilistic soft logic
arxiv(2023)
摘要
In this paper, we introduce Neural Probabilistic Soft Logic (NeuPSL), a novel neurosymbolic (NeSy) framework that unites state-of-the-art symbolic reasoning with the low-level perception of deep neural networks. To model the boundary between neural and symbolic representations, we propose a family of energy-based models, NeSy Energy-Based Models , and show that they are general enough to include NeuPSL and many other NeSy approaches. Using this framework, we show how to seamlessly integrate neural and symbolic parameter learning and inference in NeuPSL. Through an extensive empirical evaluation, we demonstrate the benefits of using NeSy methods, achieving upwards of 30% improvement over independent neural network models. On a well-established NeSy task, MNIST-Addition, NeuPSL demonstrates its joint reasoning capabilities by outperforming existing NeSy approaches by up to 10% in low-data settings. Furthermore, NeuPSL achieves a 5% boost in performance over state-of-the-art NeSy methods in a canonical citation network task with up to a 40 times speed up.
更多查看译文
关键词
neural probabilistic soft logic
AI 理解论文
溯源树
样例
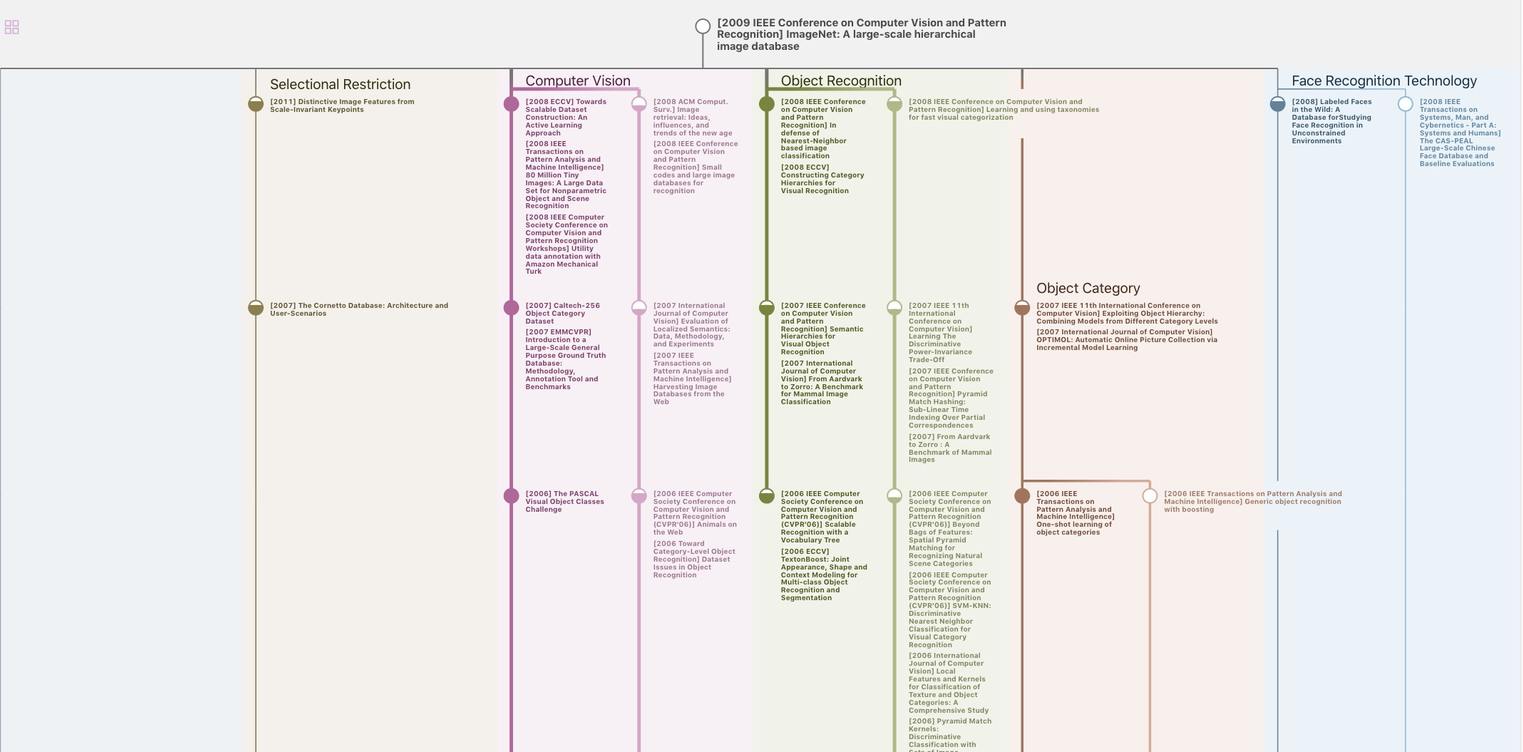
生成溯源树,研究论文发展脉络
Chat Paper
正在生成论文摘要