A Cognitive-Driven Ordinal Preservation for Multimodal Imbalanced Brain Disease Diagnosis
IEEE TRANSACTIONS ON COGNITIVE AND DEVELOPMENTAL SYSTEMS(2023)
摘要
The multimodal imbalanced data learning problems are becoming increasingly common in the real world, especially in brain disease diagnosis. Although multimodal data provide complementary information for decision making, it can also lead the model to be more sensitive to the adverse effects of imbalance. The existing imbalance learning methods are mostly based on single modality. In this article, we design a cognitive driven ordinal preservation model optimized in both feature and sample levels for multimodal imbalanced data. At the feature level, we project the original data into the label space by a second-order Laplacian manifold for better capturing the minor changes and preserving the discriminative information among samples. At the sample level, we derive the class-specific self-paced learning, simulating human cognitive mechanism, to drive data participating in learning from balance subset to the whole set, which can reduce the negative effects of imbalance on the learning model. Meanwhile, we impose the group sparse constraint on the projection matrix to embed the latent relationship pattern among different modalities, and theoretically prove its convergence. The proposed method is applied to multimodal brain disease diagnosis, including Alzheimer's disease (AD) and epilepsy. The experimental results show that our method outperforms the existing imbalance and fusion algorithms.
更多查看译文
关键词
Imbalanced data learning,multimodal fusion,second-order structure,self-paced learning
AI 理解论文
溯源树
样例
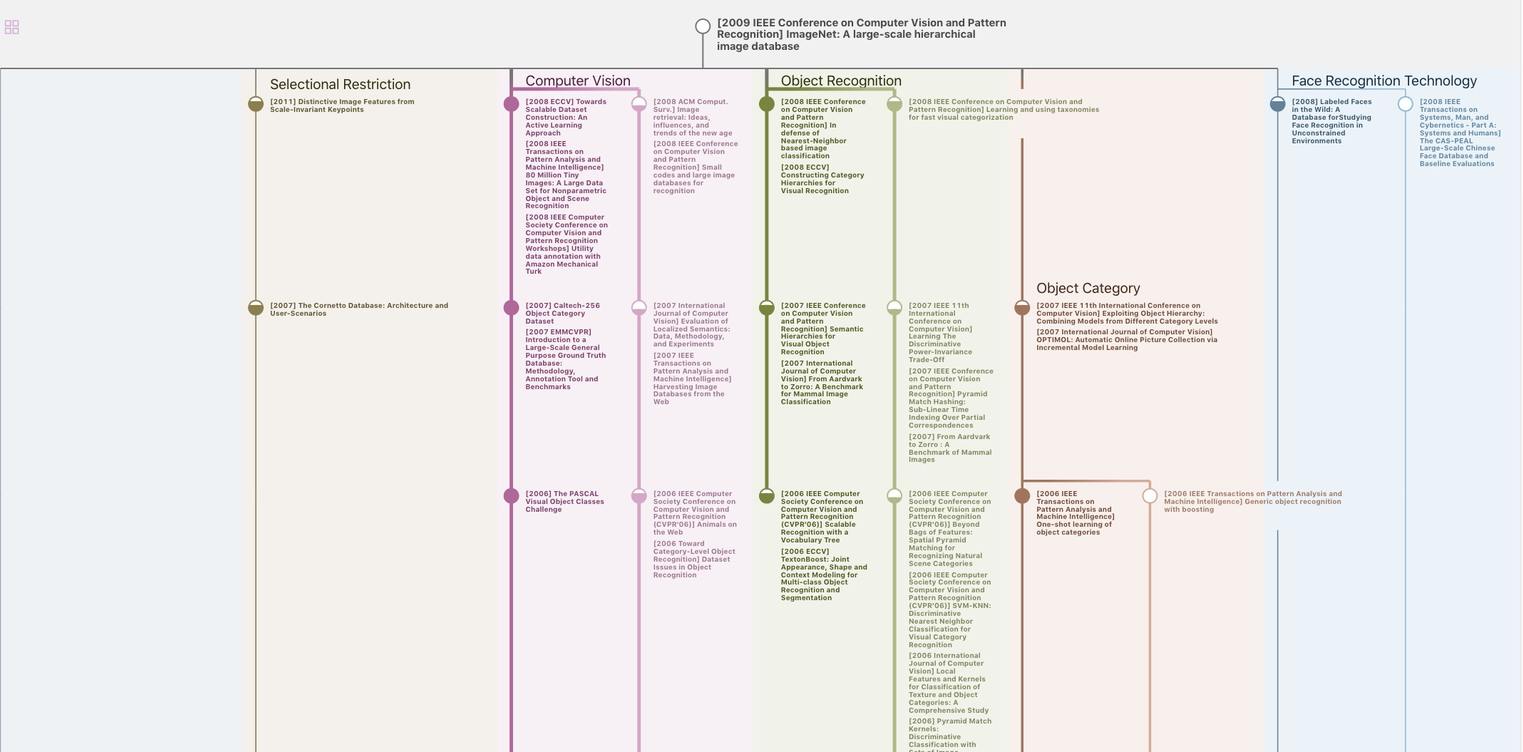
生成溯源树,研究论文发展脉络
Chat Paper
正在生成论文摘要