Learning multi-scale functional representations of proteins from single-cell microscopy data
arxiv(2022)
摘要
Protein function is inherently linked to its localization within the cell, and fluorescent microscopy data is an indispensable resource for learning representations of proteins. Despite major developments in molecular representation learning, extracting functional information from biological images remains a non-trivial computational task. Current state-of-the-art approaches use autoencoder models to learn high-quality features by reconstructing images. However, such methods are prone to capturing noise and imaging artifacts. In this work, we revisit deep learning models used for classifying major subcellular localizations, and evaluate representations extracted from their final layers. We show that simple convolutional networks trained on localization classification can learn protein representations that encapsulate diverse functional information, and significantly outperform autoencoder-based models. We also propose a robust evaluation strategy to assess quality of protein representations across different scales of biological function.
更多查看译文
关键词
proteins,microscopy,learning,multi-scale,single-cell
AI 理解论文
溯源树
样例
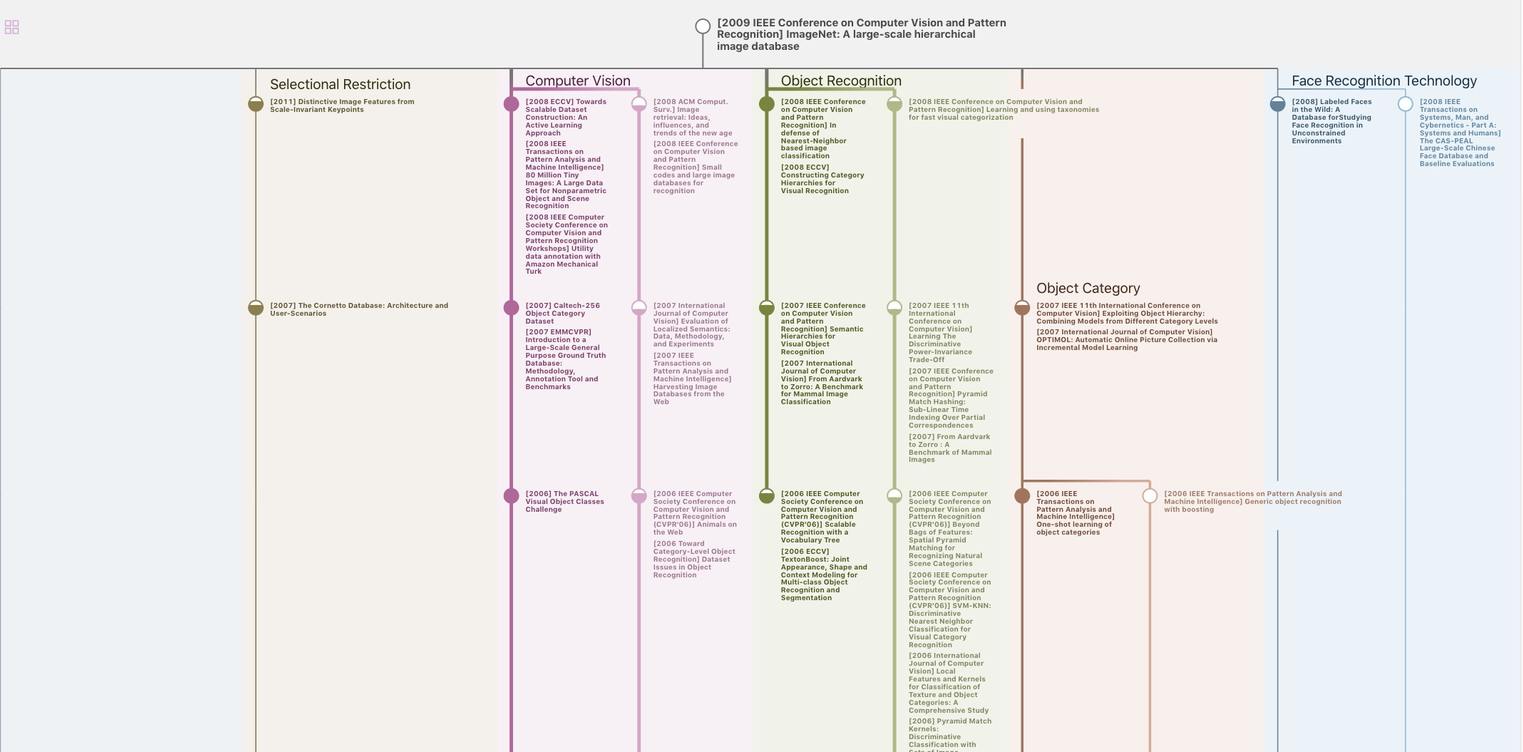
生成溯源树,研究论文发展脉络
Chat Paper
正在生成论文摘要