Multi-Sensor Data Driven with PARAFAC-IPSO-PNN for Identification of Mechanical Nonstationary Multi-Fault Mode
Machines(2022)
Key words
parallel factor analysis (PFA),feature extraction,probabilistic neural network (PNN),fault diagnosis
AI Read Science
Must-Reading Tree
Example
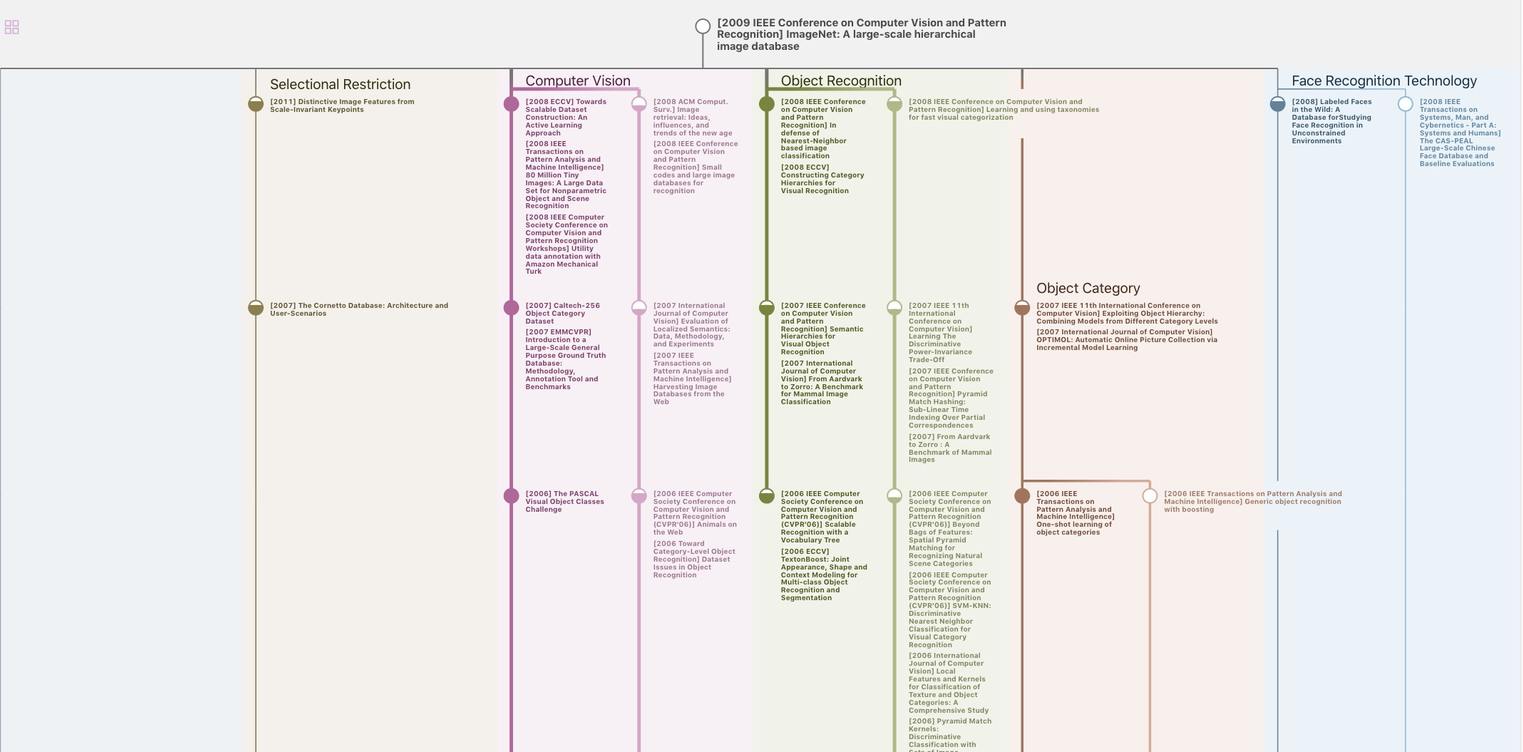
Generate MRT to find the research sequence of this paper
Chat Paper
Summary is being generated by the instructions you defined