Debubbling seismic data using a generalized neural network
GEOPHYSICS(2022)
摘要
Estimating the far-field source signature has always been an important part of seismic processing. However, estimating the source signature from an air-gun array is difficult because of the complex interaction between the air bubble oscillations from each air gun, the state of the sea surface, variations in air pressure, the air guns' geometry, etc. Removing the bubble noise is important because proper seismic imaging requires a zero-phased, spiky wavelet. Debubbling has conventionally been done by deconvolution using an (assumed) known source signature. Several methods to estimate the signature and debubble the data have been implemented, for instance, source modeling or using near-field hydrophone measurements. We describe an alternative approach using a convolutional neural network for debubbling. The network is trained on real data containing a large range of source signatures to make the network robust and adaptive to signature variations. If the signature in the test data is equal to one of the signatures used in the training, the network performs well. In addition, if the signature changes in the middle of a sail line, the network can adapt to this change. Moreover, we investigate the network's sensitivity to changing geology within a survey and on two different surveys on the Norwegian Continental Shelf (NCS). If the test data are from geology similar to the training data, the network performs better than if not. Even when applied to a different part of the NCS, the network is still able to remove most of the bubble noise.
更多查看译文
关键词
seismic data,generalized neural network,neural network
AI 理解论文
溯源树
样例
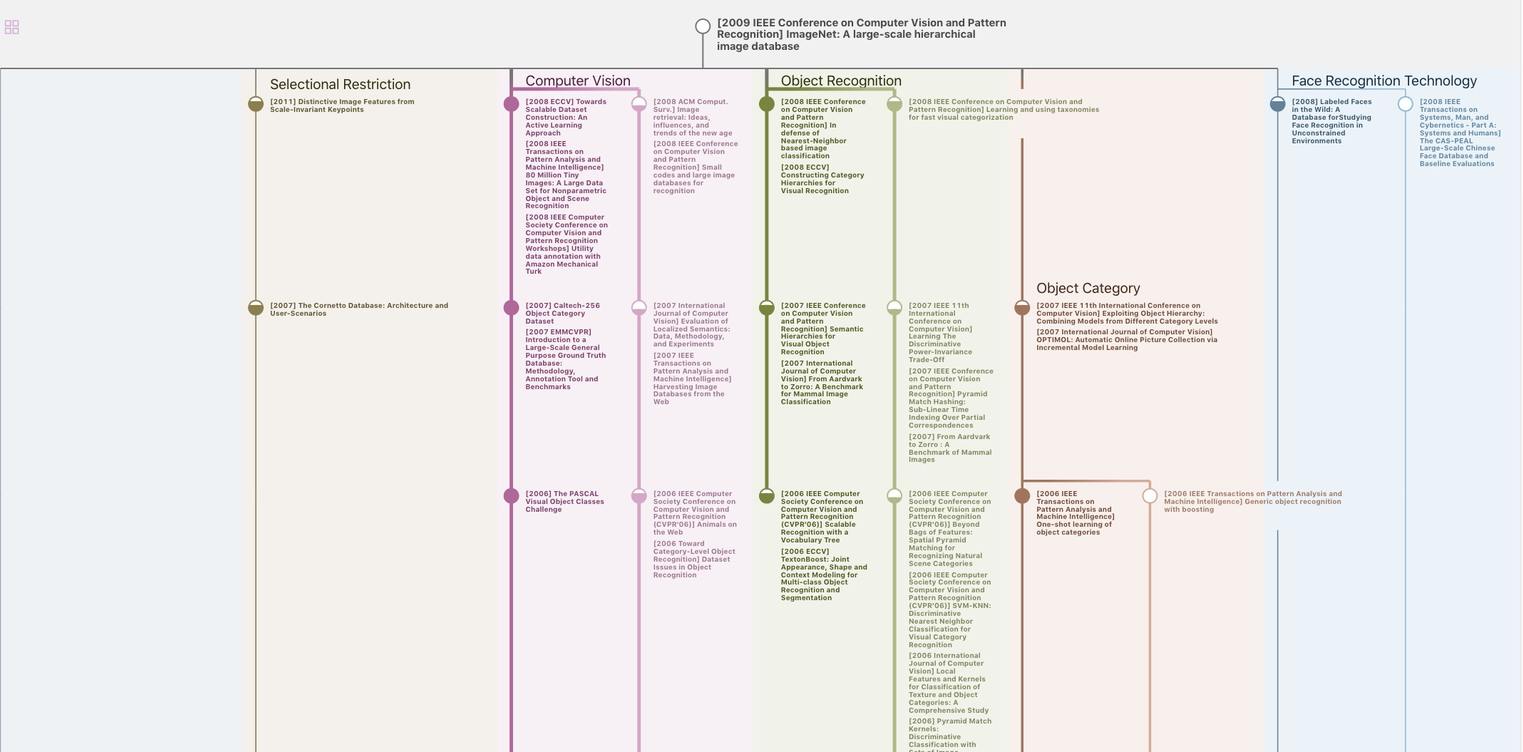
生成溯源树,研究论文发展脉络
Chat Paper
正在生成论文摘要