Multistep forecast of the implied volatility surface using deep learning
JOURNAL OF FUTURES MARKETS(2022)
摘要
Modeling implied volatility surface (IVS) is of paramount importance to price and hedge an option. We contribute to the literature by modeling the entire IVS using convolutional long-short-term memory (ConvLSTM) and long-short-term memory (LSTM) neural networks to produce multivariate and multistep forecasts of the S&P 500 IVS. Using daily SPX options data (2002-2019), we find that both LSTM and ConvLSTM fit the training data extremely well with mean absolute percentage error (MAPE) being 3.56% and 3.88%, respectively. The ConvLSTM (8.26% MAPE) model significantly outperforms LSTM and traditional time series models in predicting the IVS out of sample.
更多查看译文
关键词
ConvLSTM, convolutional neural networks, deep learning, implied volatility, LSTM
AI 理解论文
溯源树
样例
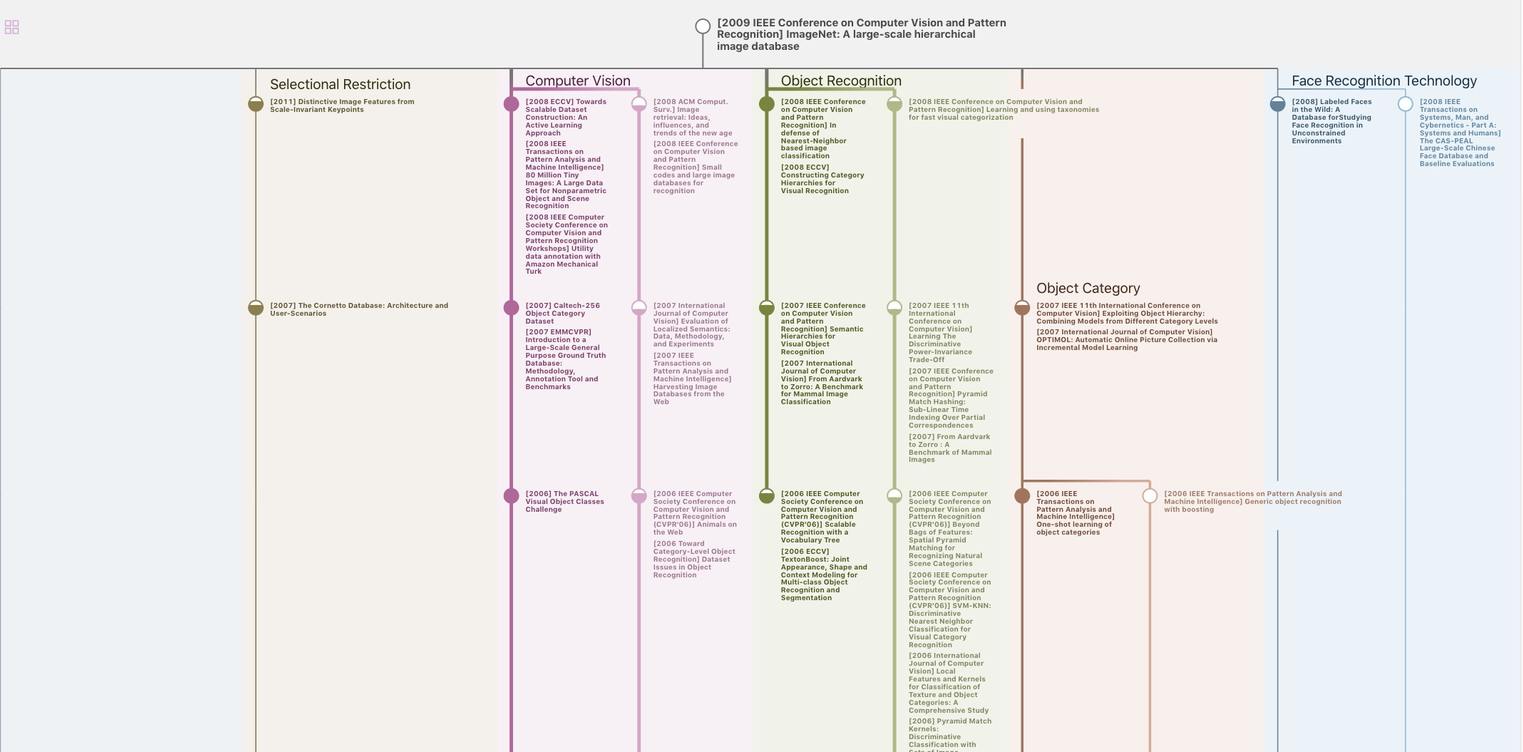
生成溯源树,研究论文发展脉络
Chat Paper
正在生成论文摘要