Continuous On‐board Behaviour Classification Using Accelerometry: A Case Study with a New GPS‐3G‐Bluetooth System in Pacific Black Ducks
Methods in ecology and evolution(2022)
摘要
Over the past two decades, accelerometer (ACC) data have been increasingly used to study animal behaviours and energetics. However, the large amount of raw ACC data can be a burden to device storage and power consumption and in many cases may also require device retrieval for data collection. On-board data processing to reduce data volume and power consumption for data transmission may hold promise to alleviate these problems and allow for next-generation, smart trackers. We developed a tracking system processing raw ACC data on-board of trackers into behaviours using an XGBoost machine learning model. We used this system on six free-ranging Pacific black ducks Anas superciliosa to study eight behaviours every 2 s. The on-board XGBoost model for behaviour classification had 92.04% overall accuracy. One day of continuous behaviour records was compressed on-board to as little as 17.28 kB for routine transmission via the 3G network. We received behaviour data from the six ducks continuously for periods ranging between 56 days and 14 months. On-board processing of raw ACC data and data transmission proofed highly energy efficient and came at a minimal weight cost to the trackers, providing great potential to open up new areas in ecological and behavioural research.
更多查看译文
关键词
accelerometer,machine learning,time-activity budget,wildlife tracking,XGBoost
AI 理解论文
溯源树
样例
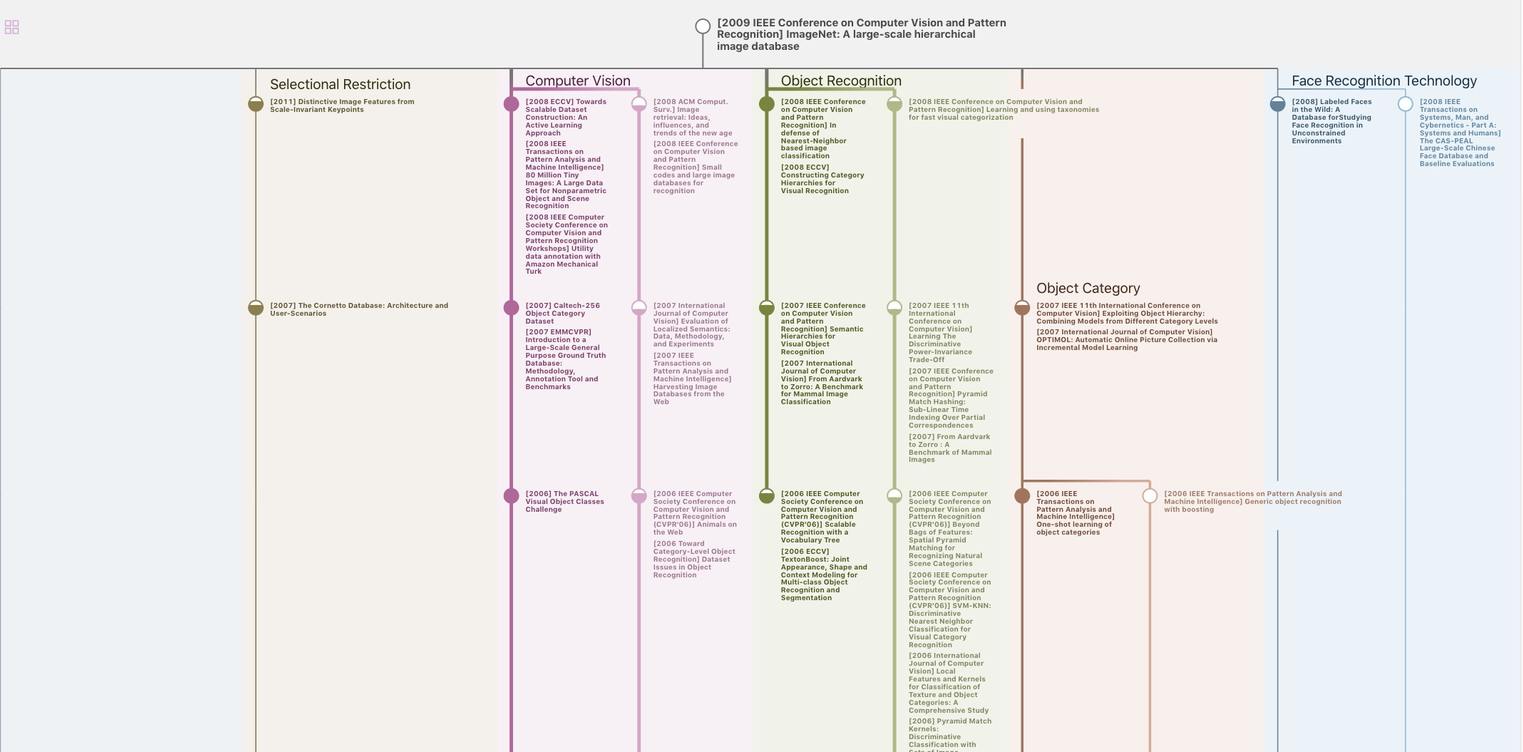
生成溯源树,研究论文发展脉络
Chat Paper
正在生成论文摘要