A Lightweight Learning Method for Stochastic Configuration Networks Using Non-Inverse Solution
ELECTRONICS(2022)
摘要
Stochastic configuration networks (SCNs) face time-consuming issues when dealing with complex modeling tasks that usually require a mass of hidden nodes to build an enormous network. An important reason behind this issue is that SCNs always employ the Moore-Penrose generalized inverse method with high complexity to update the output weights in each increment. To tackle this problem, this paper proposes a lightweight SCNs, called L-SCNs. First, to avoid using the Moore-Penrose generalized inverse method, a positive definite equation is proposed to replace the over-determined equation, and the consistency of their solution is proved. Then, to reduce the complexity of calculating the output weight, a low complexity method based on Cholesky decomposition is proposed. The experimental results based on both the benchmark function approximation and real-world problems including regression and classification applications show that L-SCNs are sufficiently lightweight.
更多查看译文
关键词
stochastic configuration networks,Cholesky decomposition,lightweight
AI 理解论文
溯源树
样例
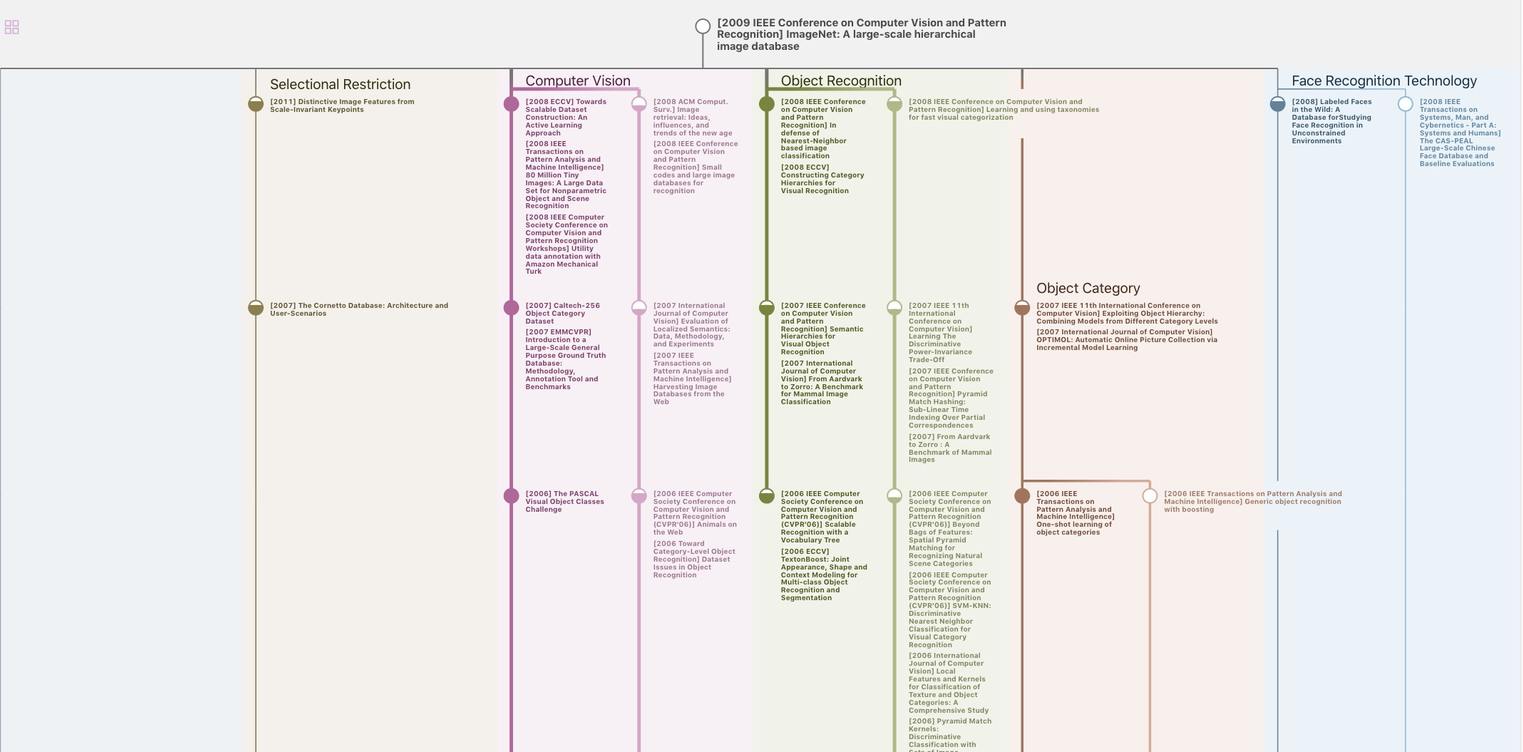
生成溯源树,研究论文发展脉络
Chat Paper
正在生成论文摘要