IoT Dataset Validation Using Machine Learning Techniques for Traffic Anomaly Detection
ELECTRONICS(2021)
Abstract
With advancements in engineering and science, the application of smart systems is increasing, generating a faster growth of the IoT network traffic. The limitations due to IoT restricted power and computing devices also raise concerns about security vulnerabilities. Machine learning-based techniques have recently gained credibility in a successful application for the detection of network anomalies, including IoT networks. However, machine learning techniques cannot work without representative data. Given the scarcity of IoT datasets, the DAD emerged as an instrument for knowing the behavior of dedicated IoT-MQTT networks. This paper aims to validate the DAD dataset by applying Logistic Regression, Naive Bayes, Random Forest, AdaBoost, and Support Vector Machine to detect traffic anomalies in IoT. To obtain the best results, techniques for handling unbalanced data, feature selection, and grid search for hyperparameter optimization have been used. The experimental results show that the proposed dataset can achieve a high detection rate in all the experiments, providing the best mean accuracy of 0.99 for the tree-based models, with a low false-positive rate, ensuring effective anomaly detection.
MoreTranslated text
Key words
IoT,sensors,dataset validation,machine learning,intrusion detection systems,analysis,metric,algorithm design
AI Read Science
Must-Reading Tree
Example
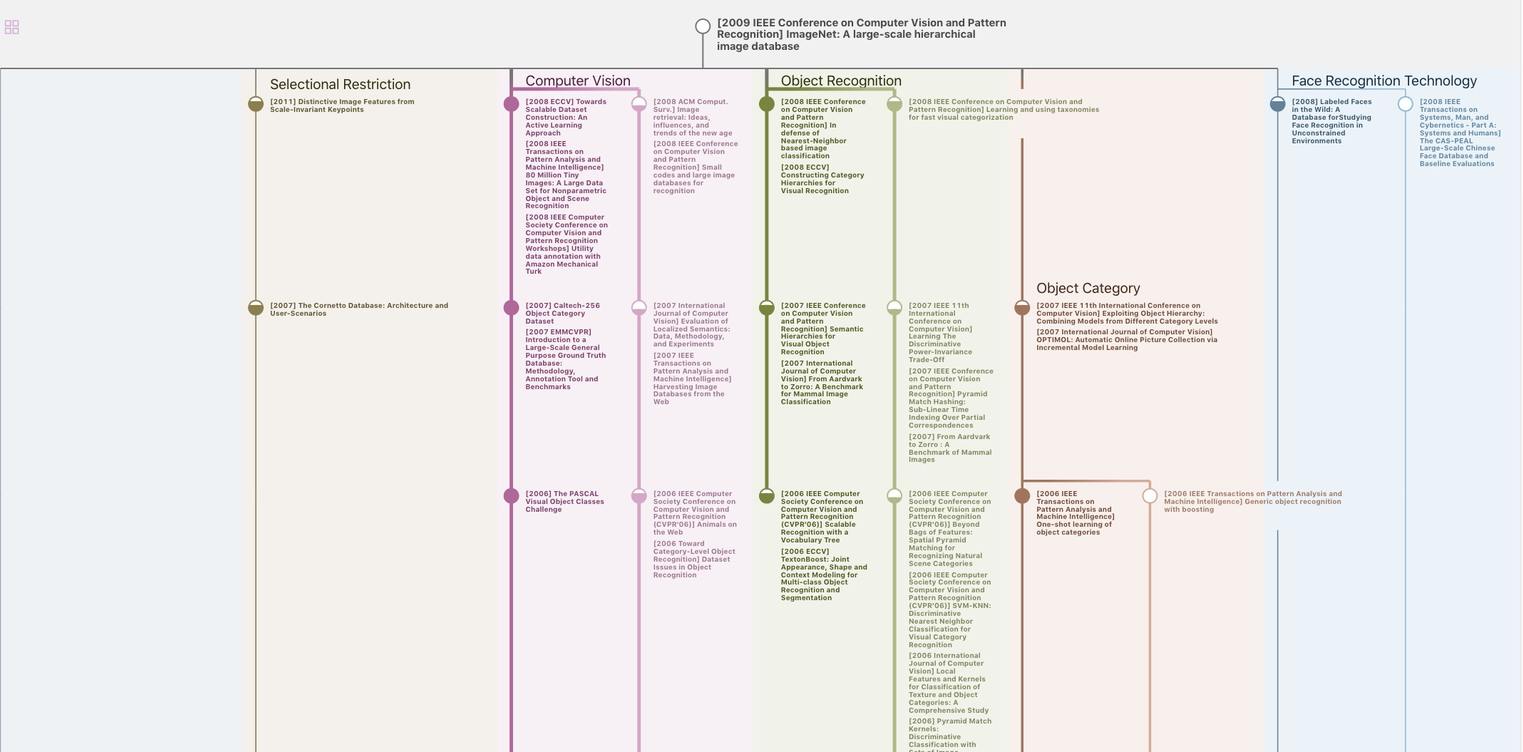
Generate MRT to find the research sequence of this paper
Chat Paper
Summary is being generated by the instructions you defined