Active Learning for Deep Gaussian Process Surrogates.
TECHNOMETRICS(2023)
摘要
Deep Gaussian processes (DGPs) are increasingly popular as predictive models in machine learning for their nonstationary flexibility and ability to cope with abrupt regime changes in training data. Here, we explore DGPs as surrogates for computer simulation experiments whose response surfaces exhibit similar characteristics. In particular, we transport a DGP's automatic warping of the input space and full uncertainty quantification, via a novel elliptical slice sampling Bayesian posterior inferential scheme, through to active learning strategies that distribute runs nonuniformly in the input space-something an ordinary (stationary) GP could not do. Building up the design sequentially in this way allows smaller training sets, limiting both expensive evaluation of the simulator code and mitigating cubic costs of DGP inference. When training data sizes are kept small through careful acquisition, and with parsimonious layout of latent layers, the framework can be both effective and computationally tractable. Our methods are illustrated on simulation data and two real computer experiments of varying input dimensionality. We provide an open source implementation in the deepgp package on CRAN.
更多查看译文
关键词
Computer model,Elliptical slice sampling,Emulator,Kriging,Sequential design
AI 理解论文
溯源树
样例
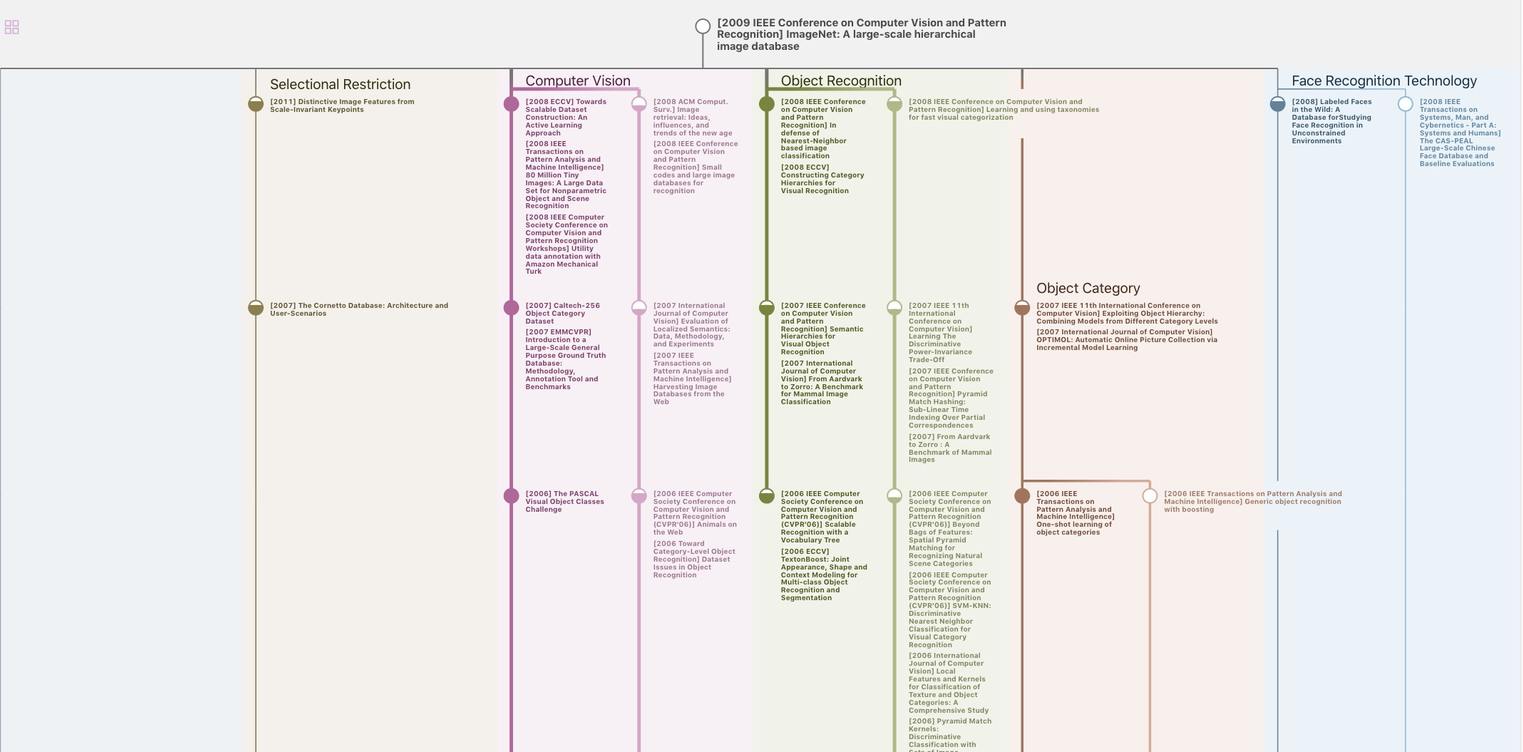
生成溯源树,研究论文发展脉络
Chat Paper
正在生成论文摘要