Combined Remaining Life Prediction of Multiple Bearings Based on EEMD-BILSTM.
Symmetry(2022)
摘要
To improve the accuracy of a symmetrical structural rolling bearing life prediction under noise interference, a multi-bearing life prediction method combining Ensemble Empirical Mode Decomposition (EEMD) and Bi-directional Long Short-Term Memory (BiLSTM) is proposed. First, EEMD is proposed to decompose the original vibration signal to obtain a finite number of Intrinsic Mode Function (IMF), and the IMFs are further filtered by combining the correlation criterion and kurtosis criterion. Then, the time domain features and frequency domain features of the reconstructed signal are filtered by monotonicity index to obtain the set of features containing key information. Finally, the BiLSTM network is trained on the filtered features set, and the method is proved to accurately predict the remaining life of rolling bearings under different operating conditions through rolling bearing full-life experiments, and the effectiveness of the method is verified by comparing the prediction results with several main recurrent neural networks.
更多查看译文
关键词
rolling bearing,ensemble empirical mode decomposition,bidirectional long-short term memory network,life prediction
AI 理解论文
溯源树
样例
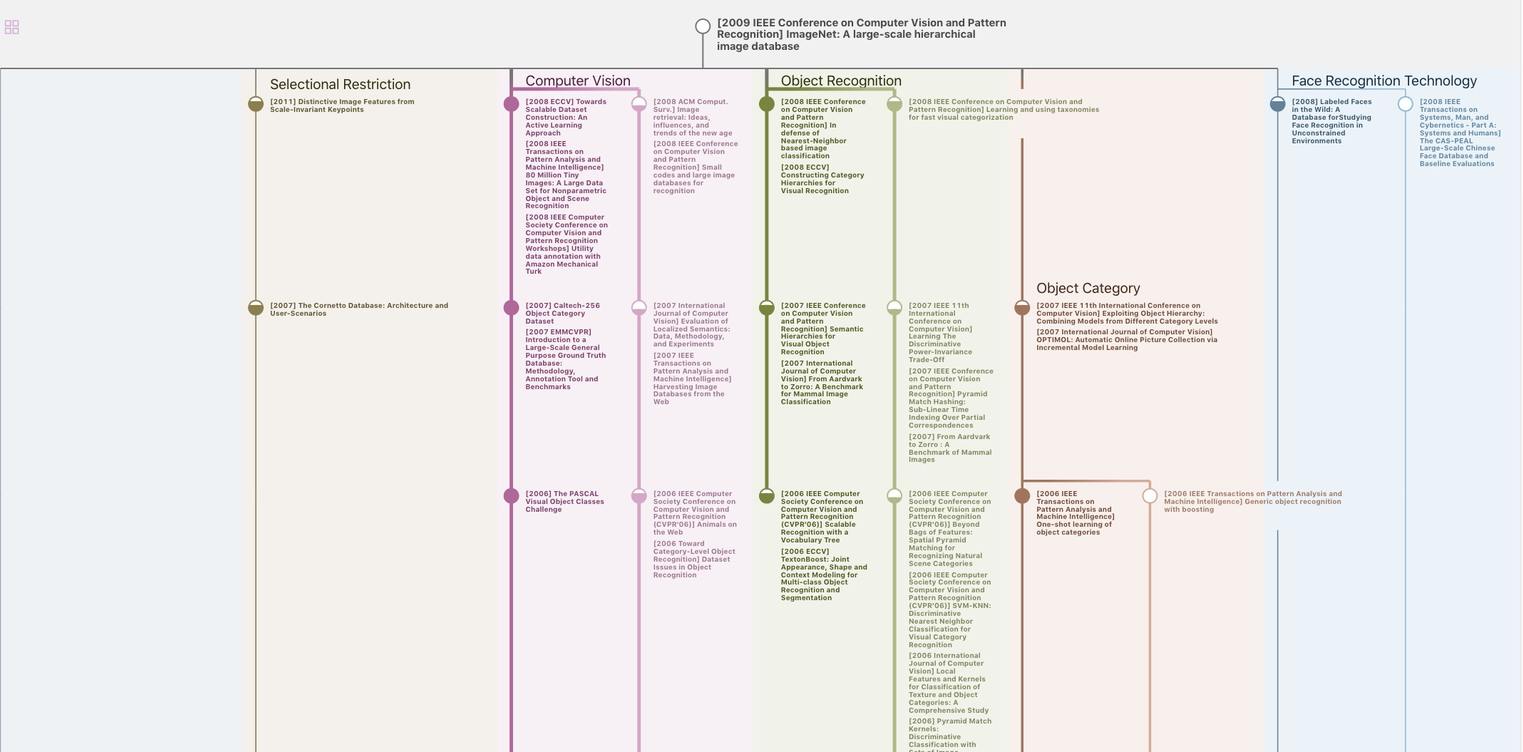
生成溯源树,研究论文发展脉络
Chat Paper
正在生成论文摘要