SimTriplet: Simple Triplet Representation Learning with a Single GPU
Lecture notes in computer science(2021)
关键词
Contrastive learning,SimTriplet,Classification,Pathology
AI 理解论文
溯源树
样例
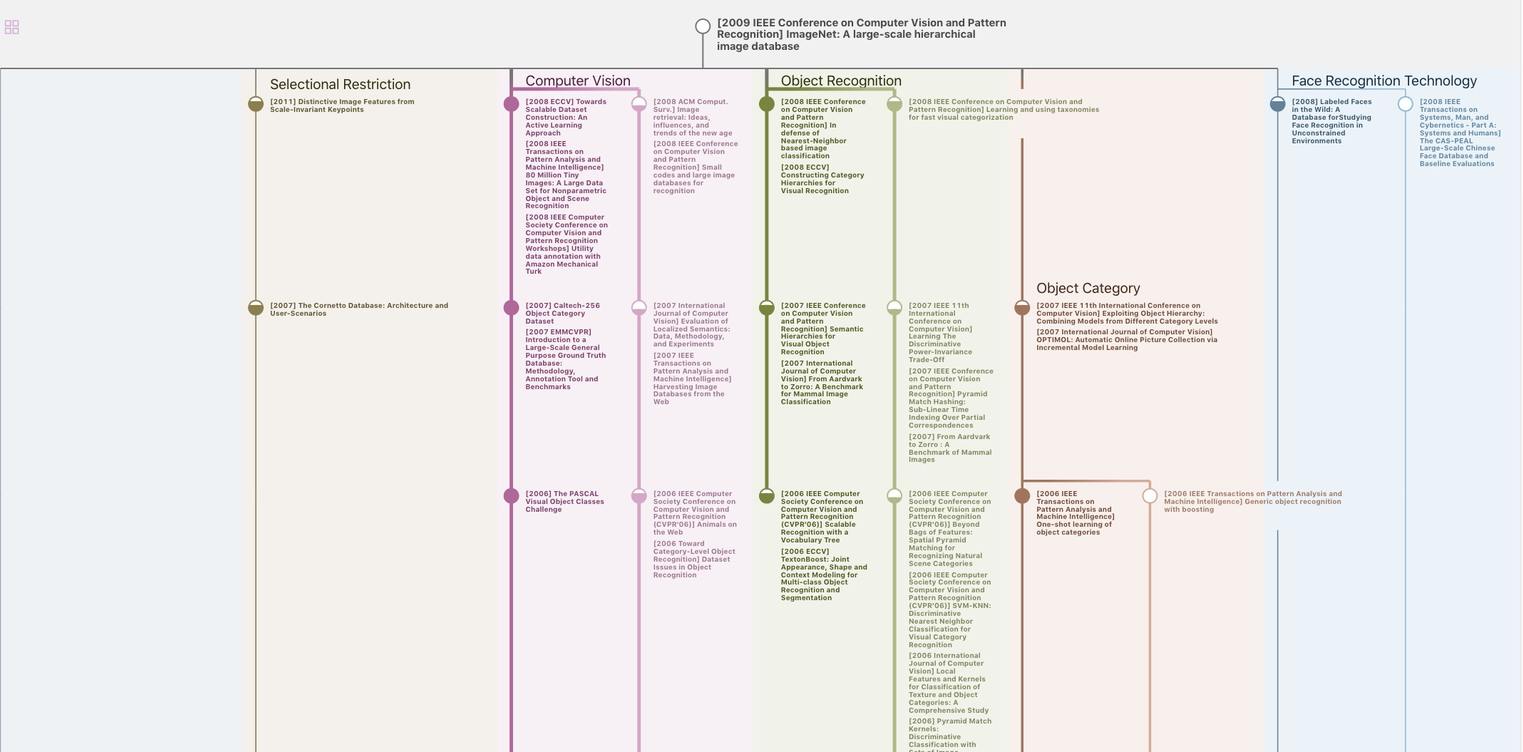
生成溯源树,研究论文发展脉络
Chat Paper
正在生成论文摘要
Lecture notes in computer science(2021)