Predicting Perovskite Bandgap and Solar Cell Performance with Machine Learning
SOLAR RRL(2022)
摘要
Perovskites as semiconductors are of profound interest and arguably, the investigation on the distinctive perovskite composition is paramount to fabricate efficient devices and solar cells. The role of anion and cations and their impact on optoelectronic and photovoltaic properties is probed. A machine learning (ML) approach to predict the bandgap and power conversion efficiency (PCE) using eight different perovskites compositions is reported. The predicted solar cell parameters validate the experimental data. The adopted Random forest model presents a good match with high R-2 scores of >0.99 and >0.82 for predicted absorption and J-V datasets, respectively, and show minimal error rates with a precise prediction of bandgap and PCEs. The results suggest that the ML technique is an innovative approach to aid the preparation of the perovskite and can accelerate the commercial aspects of perovskite solar cells without fabricating working devices and minimize the fabrication steps and save cost.
更多查看译文
关键词
machine learning,optoelectrical properties,perovskite solar cells,perovskites,random forest model
AI 理解论文
溯源树
样例
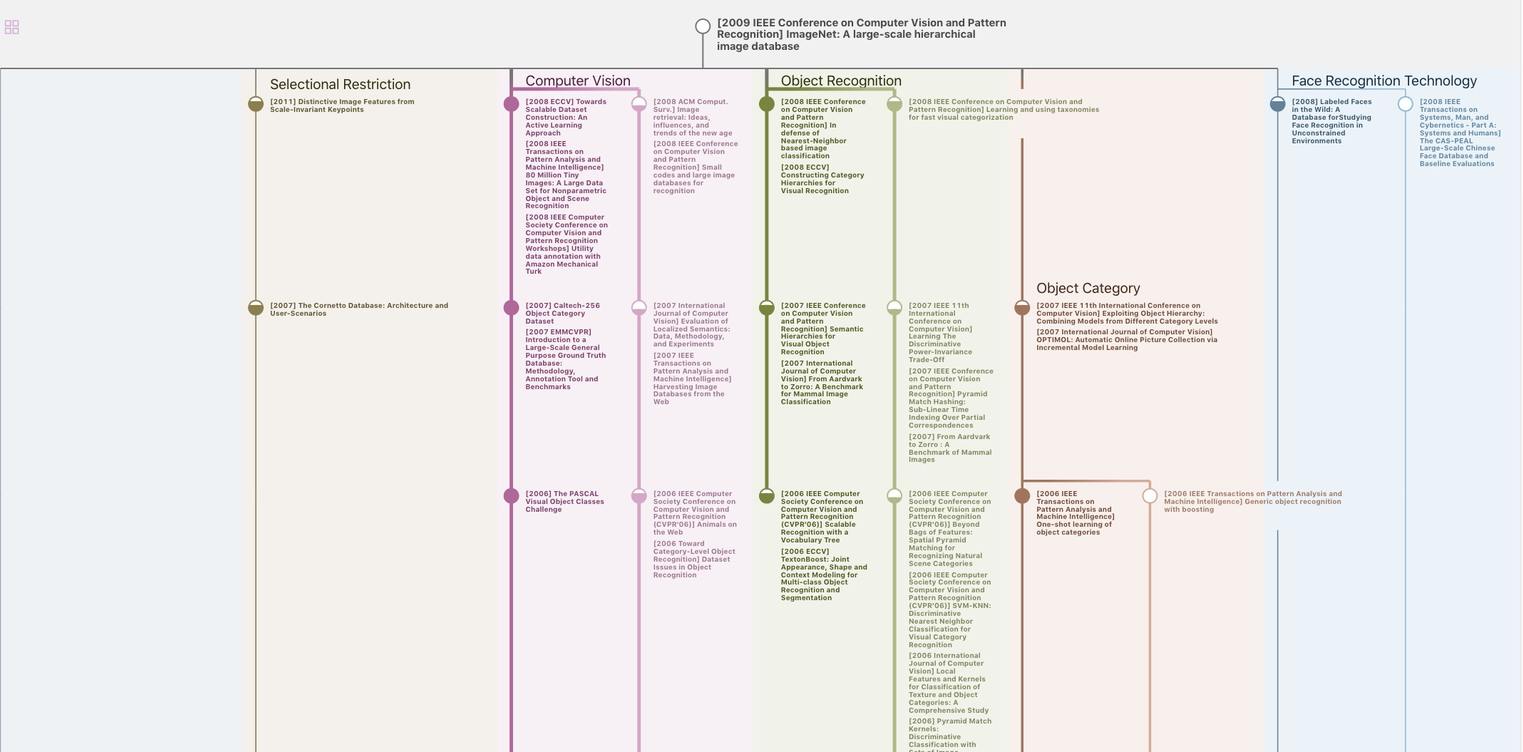
生成溯源树,研究论文发展脉络
Chat Paper
正在生成论文摘要