Photogrammetric 3D mobile mapping of rail tracks
ISPRS Journal of Photogrammetry and Remote Sensing(2022)
摘要
Recent developments in the field of rail vehicles increased the demand for accurate and up-to-date 3D maps of rail track networks. Collision avoidance systems, semi-automated, or fully autonomous rail vehicles strongly benefit from such high quality maps. In this work, we present a fully automatic, photogrammetric method for the 3D reconstruction of rail track segments. More specifically, the center line of the rail track is reconstructed as a georeferenced and continuous 3D cubic spline. The main data inputs are collected while driving the rail vehicle along the segment: (a) images from a front-looking camera and (b) observations from a low-cost GNSS receiver. Optional data inputs can be used to increase the reconstruction accuracy, namely (c) an a priori rail track (e.g. from OpenStreetMap), (d) a digital height model (DHM), and (e) ground control points (GCPs). The rail track is estimated in post processing (offline) by a weighted least squares adjustment (LSA). The core of the LSA is the bundle adjustment of images. It is extended by additional geometric constraints which exploit the geometric relations between the rail track, the rail vehicle, and the camera trajectory. As a consequence, in contrast to many related methods, the rails need not to be visible in the images to map the rail track. We applied the method to reconstruct a 13 km long tram line in Vienna (Austria). We found that the local geometry of the track can be well reconstructed from the image sequence. However, if the low-cost GNSS receiver is used as single georeferencing source, the track shows a strong drift behavior. This drift can significantly be minimized over the entire track if the above mentioned optional data inputs are used.
更多查看译文
关键词
Photogrammetry,Mobile mapping,Least squares adjustment,Calibration,Rail mapping
AI 理解论文
溯源树
样例
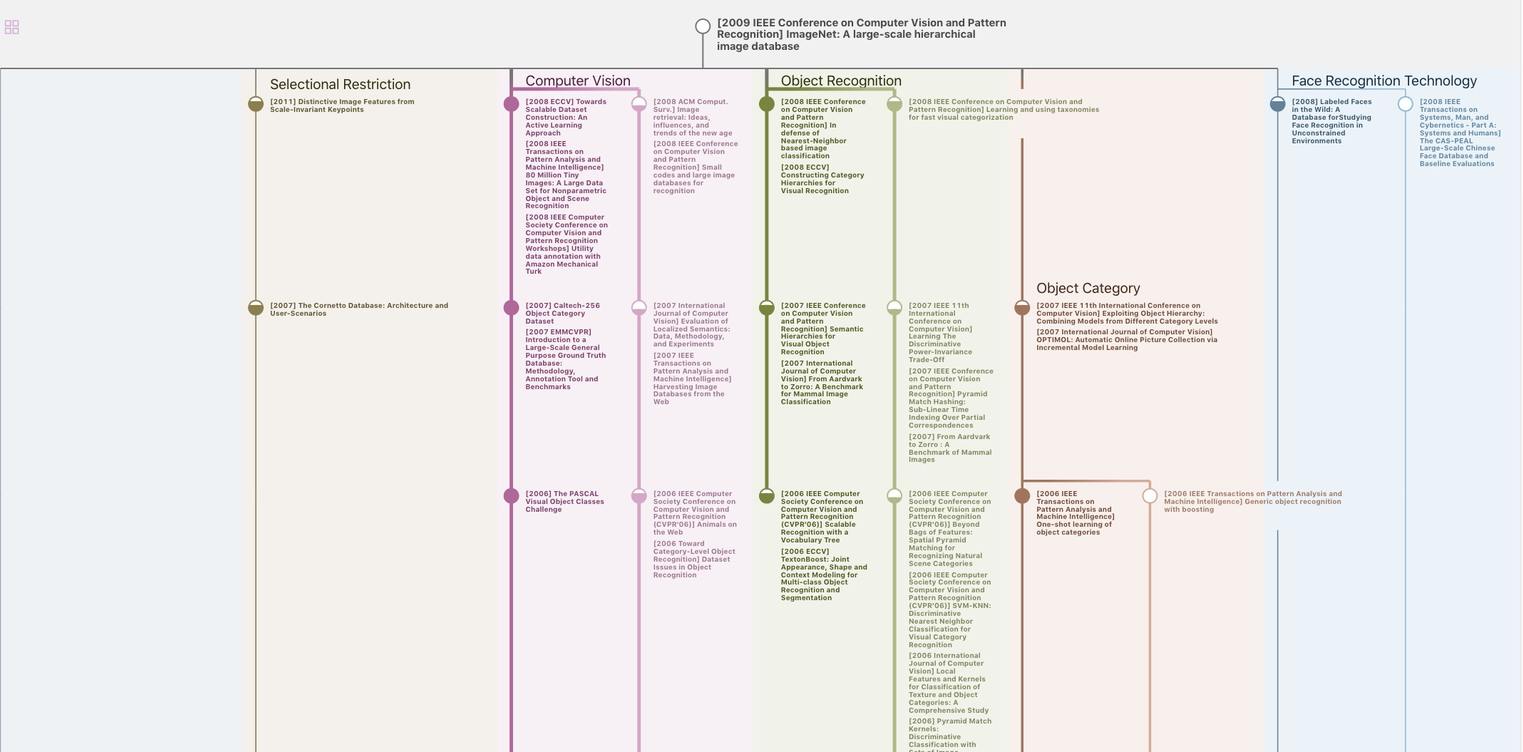
生成溯源树,研究论文发展脉络
Chat Paper
正在生成论文摘要