Development of CNN models for the enteral feeding tube positioning assessment on a small scale data set
BMC MEDICAL IMAGING(2022)
摘要
Background Enteral nutrition through feeding tubes serves as the primary method of nutritional supplementation for patients unable to feed themselves. Plain radiographs are routinely used to confirm the position of the Nasoenteric feeding tubes the following insertion and before the commencement of tube feeds. Convolutional neural networks (CNNs) have shown encouraging results in assisting the tube positioning assessment. However, robust CNNs are often trained using large amounts of manually annotated data, which challenges applying CNNs on enteral feeding tube positioning assessment. Method We build a CNN model for feeding tube positioning assessment by pre-training the model under a weakly supervised fashion on large quantities of radiographs. Since most of the model was pre-trained, a small amount of labeled data is needed when fine-tuning the model for tube positioning assessment. We demonstrate the proposed method using a small dataset with 175 radiographs. Result The experimental result shows that the proposed model improves the area under the receiver operating characteristic curve (AUC) by up to 35.71% , from 0.56 to 0.76, and 14.49% on the accuracy, from 0.69 to 0.79 when compared with the no pre-trained method. The proposed method also has up to 40% less error when estimating its prediction confidence. Conclusion Our evaluation results show that the proposed model has a high prediction accuracy and a more accurate estimated prediction confidence when compared to the no pre-trained model and other baseline models. The proposed method can be potentially used for assessing the enteral tube positioning. It also provides a strong baseline for future studies.
更多查看译文
关键词
Weakly supervised, Pre-training, Annotation-efficient modeling
AI 理解论文
溯源树
样例
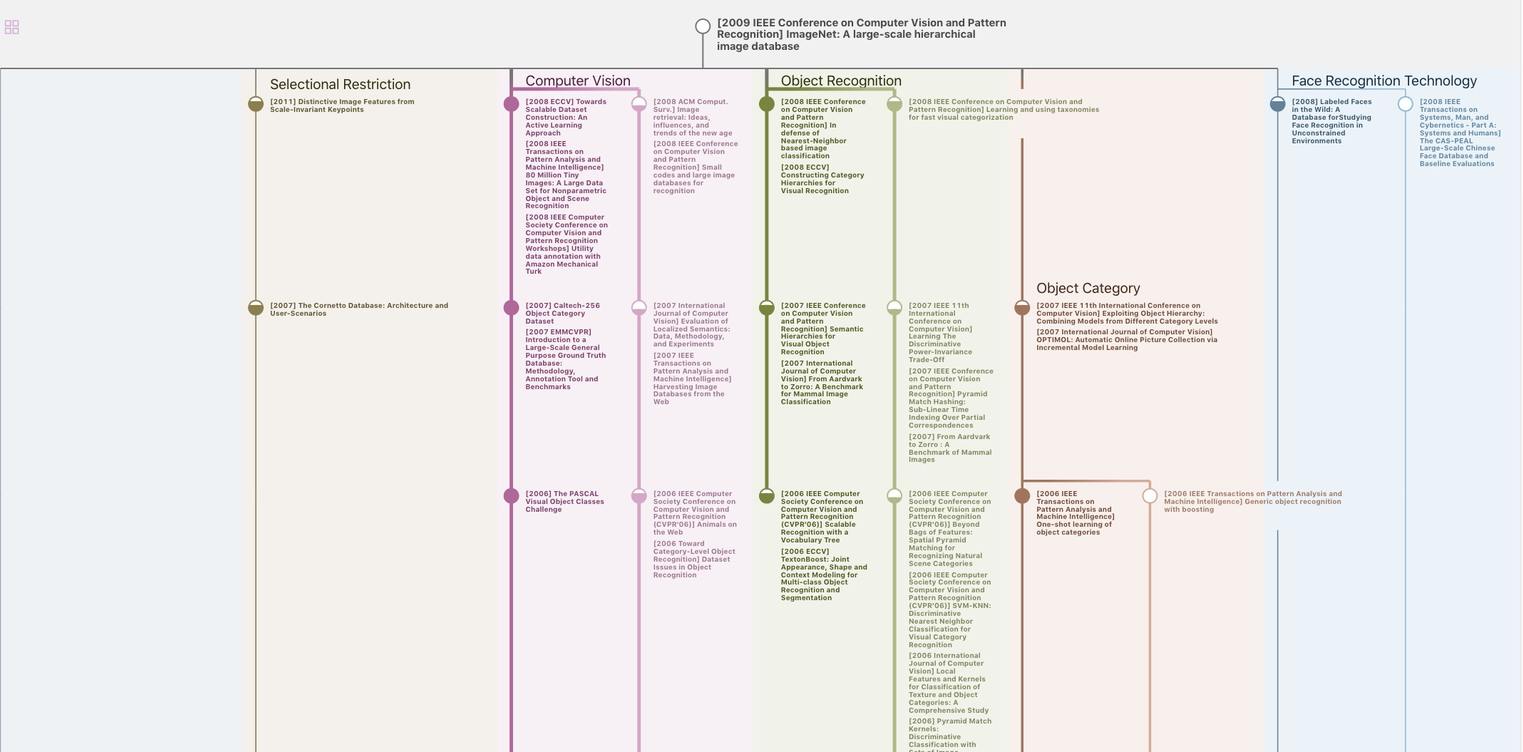
生成溯源树,研究论文发展脉络
Chat Paper
正在生成论文摘要