Investigation of satellite rainfall-driven rainfall-runoff model using deep learning approaches in two different catchments in India
JOURNAL OF HYDROINFORMATICS(2022)
摘要
Rainfall-runoff models are valuable tools for flood forecasting, management of water resources, and drought warning. With the advancement in space technology, a plethora of satellite precipitation products (SPPs) are available publicly. However, the application of the satellite data for the data-driven rainfall-runoff model is emerging and requires careful investigation. In this work, two satellite rainfall data sets, namely Global Precipitation Measurement-Integrated Multi-Satellite Retrieval Product V6 (GPM-IMERG) and Climate Hazards Group Infrared Precipitation with Station (CHIRPS), are evaluated for the development of rainfall-runoff models and the prediction of 1-day ahead streamflow. The accuracy of the data from the SPPs is compared to the India Meteorological Department (IMD)-gridded precipitation data set. Detection metrics showed that for light rainfall (1-10 mm), the probability of detection (POD) value ranges between 0.67 and 0.75 and with an increasing rainfall range, i.e., medium and heavy rainfall (10-50 mm and >50 mm), the POD values ranged from 0.24 to 0.45. These results indicate that the satellite precipitation performs satisfactorily with reference to the IMD-gridded data set. Using the daily precipitation data of nearly two decades (2000-2018) over two river basins in India's Eastern part, artificial neural network, extreme learning machine (ELM), and long short-time memory (LSTM) models are developed for rainfall-runoff modelling. One-day ahead runoff prediction using the developed rainfall-runoff modelling confirmed that both the SPPs are sufficient to drive the rainfall-runoff models with a reasonable accuracy estimated using the Nash-Sutcliffe Efficiency coefficient, correlation coefficient, and the root-mean-squared error. In particular, the 1-day streamflow forecasts for the Vamsadhara river basin (VRB) using LSTM with GPM-IMERG inputs resulted in NSC values of 0.68 and 0.67, while ELM models for Mahanadhi river basin (MRB) with the same input resulted in NSC values of 0.86 and 0.87, respectively, during training and validation stages. At the same time, the LSTM model with CHIRPS inputs for the VRB resulted in NSC values of 0.68 and 0.65, and the ELM model with CHIRPS inputs for the MRB resulted in NSC values of 0.89 and 0.88, respectively, in training and validation stages. These results indicated that both the SPPs could reliably be used with LSTM and ELM models for rainfall-runoff modelling and streamflow prediction. This paper highlights that deep learning models, such as ELM and LSTM, with the GPM-IMERG products can lead to a new horizon to provide flood forecasting in flood-prone catchments.
更多查看译文
关键词
Artificial neural networks (ANN), deep learning, extreme learning machines (ELM), flood forecasting and streamflow prediction, long short-time memory (LSTM), rainfall-runoff modelling, satellite rainfall products
AI 理解论文
溯源树
样例
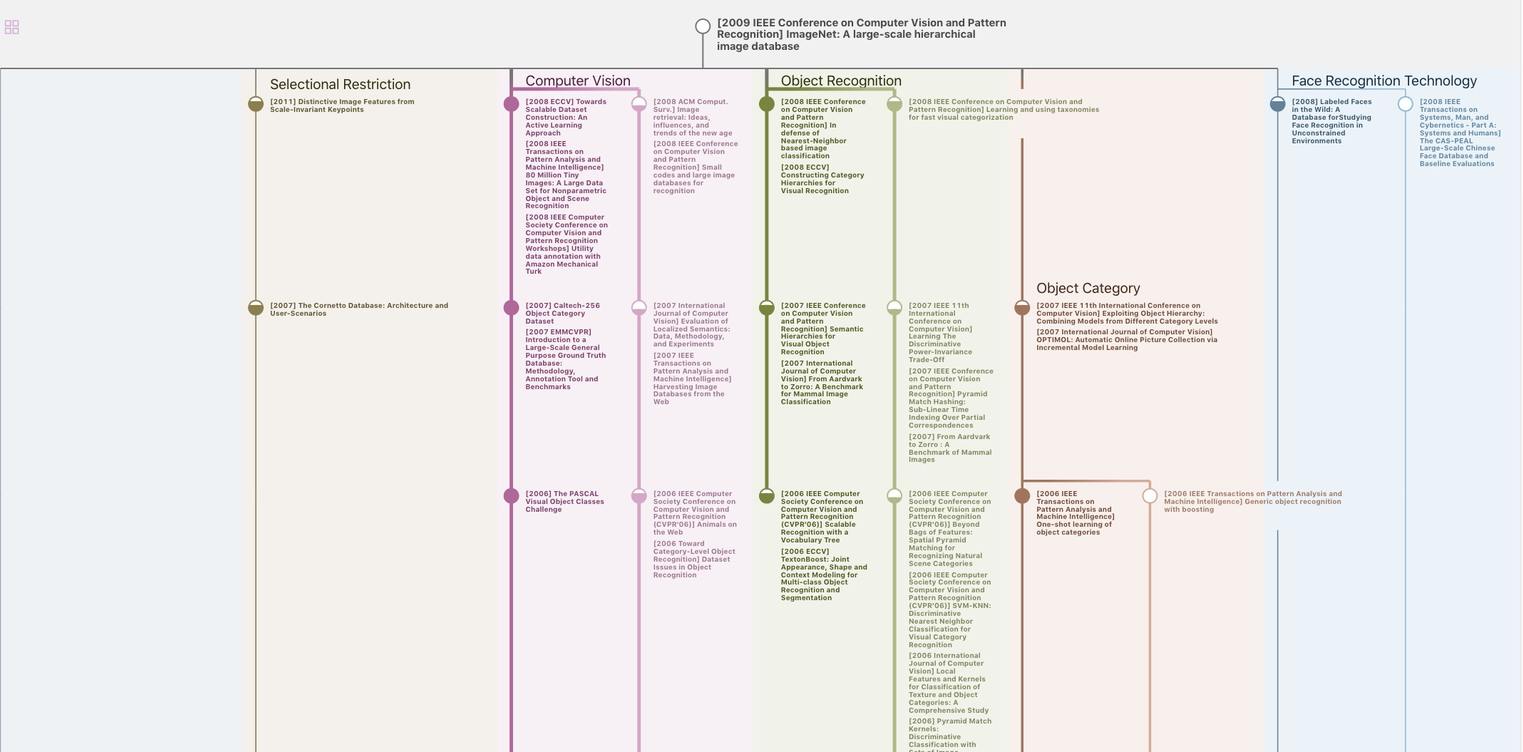
生成溯源树,研究论文发展脉络
Chat Paper
正在生成论文摘要