Early Prediction of Remaining Useful Life for Grid-Scale Battery Energy Storage System
JOURNAL OF ENERGY ENGINEERING(2021)
摘要
The grid-scale battery energy storage system (BESS) plays an important role in improving power system operation performance and promoting renewable energy integration. However, operation safety and system maintenance have been considered as significant challenges for grid-scale use of BESS. Remaining useful life (RUL) is a useful indicator of the health condition of batteries but it is especially difficult to estimate because it is dependent on many monitoring quantities from BESS. This work presents a data-driven approach that is able to fully utilize BESS monitoring data obtained from the battery management system (BMS) in order to provide an accurate and robust estimation of RUL for each individual battery cells inside a BESS. Based on raw data from historical cycling records, the proposed approach employs elastic net regression to extract characteristic features from both primary and secondary data; a back-propagation neural network based model is then established to build the relationship of refined features and the resultant RUL. The effectiveness of the RUL predictor model is verified using a large-scale data set from real-world lithium-ion battery cells and expected to be applicable to practical grid-scale BESS. (c) 2021 American Society of Civil Engineers.
更多查看译文
关键词
Battery Management Systems,Energy Storage
AI 理解论文
溯源树
样例
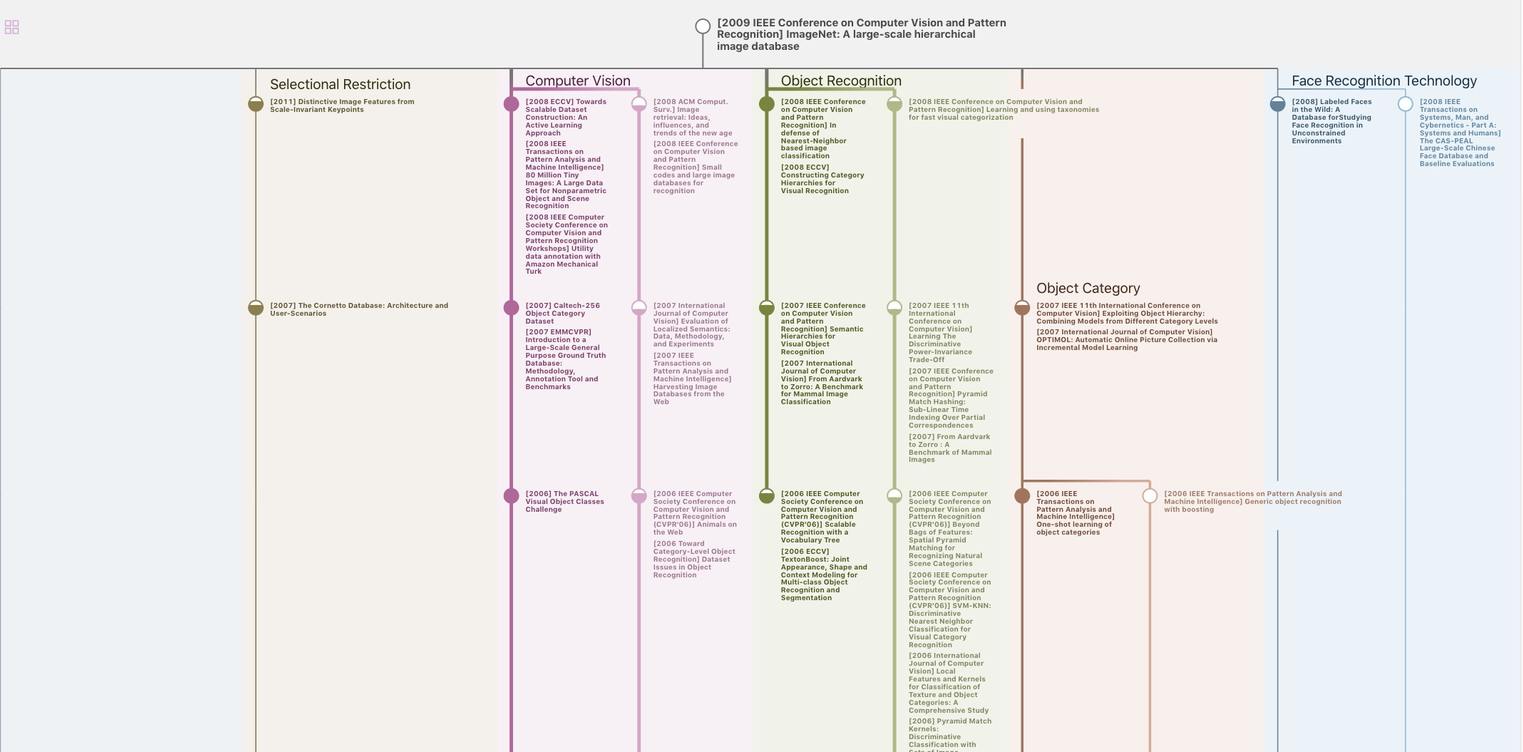
生成溯源树,研究论文发展脉络
Chat Paper
正在生成论文摘要