Deep-Neural-Network Discrimination of Multiplexed Superconducting-Qubit States
PHYSICAL REVIEW APPLIED(2022)
摘要
Demonstrating a quantum computational advantage will require high-fidelity control and readout of multiqubit systems. As system size increases, multiplexed qubit readout becomes a practical necessity to limit the growth of resource overhead. Many contemporary qubit-state discriminators presume single-qubit operating conditions or require considerable computational effort, limiting their potential extensibility. Here, we present multiqubit readout using neural networks as state discriminators. We compare our approach to contemporary methods employed on a quantum device with five superconducting qubits and frequency-multiplexed readout. We find that fully connected feedforward neural networks increase the qubit-state-assignment fidelity for our system. Relative to contemporary discriminators, the assignment error rate is reduced by up to 25% due to the compensation of system-dependent nonidealities such as readout crosstalk, which is reduced by up to one order of magnitude. Our work demonstrates a poten-tially extensible building block for high-fidelity readout relevant to both near-term devices and future
更多查看译文
AI 理解论文
溯源树
样例
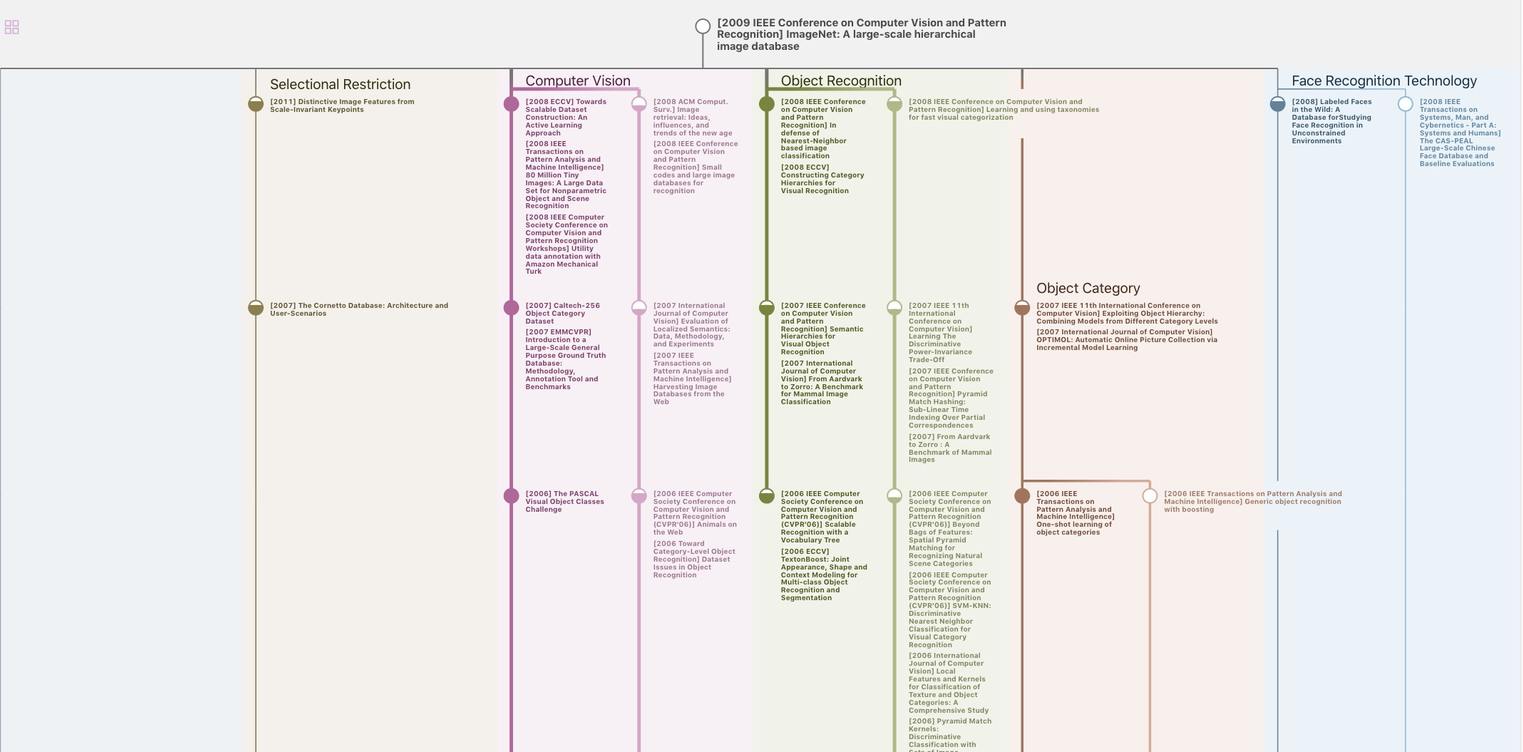
生成溯源树,研究论文发展脉络
Chat Paper
正在生成论文摘要