Deep Reinforcement Learning Model to Mitigate Congestion in Real-Time Traffic Light Networks
INFRASTRUCTURES(2021)
摘要
Urban traffic congestion has a significant detrimental impact on the environment, public health and the economy, with at a high cost to society worldwide. Moreover, it is not possible to continually modify urban road infrastructure in order to mitigate increasing traffic demand. Therefore, it is important to develop traffic control models that can handle high-volume traffic data and synchronize traffic lights in an urban network in real time, without interfering with other initiatives. Within this context, this study proposes a model, based on deep reinforcement learning, for synchronizing the traffic signals of an urban traffic network composed of two intersections. The calibration of this model, including training of its neural network, was performed using real traffic data collected at the approach to each intersection. The results achieved through simulations were very promising, yielding significant improvements in indicators measured in relation to the pre-existing conditions in the network. The model was able to deal with a broad spectrum of traffic flows and, in peak demand periods, reduced delays and queue lengths by more than 28% and 42%, respectively.
更多查看译文
关键词
smart cities,smart infrastructure,infrastructure optimization,real-time urban traffic control,traffic light synchronization,traffic light plan optimization,artificial intelligence techniques,deep reinforcement learning,inductive loop detectors
AI 理解论文
溯源树
样例
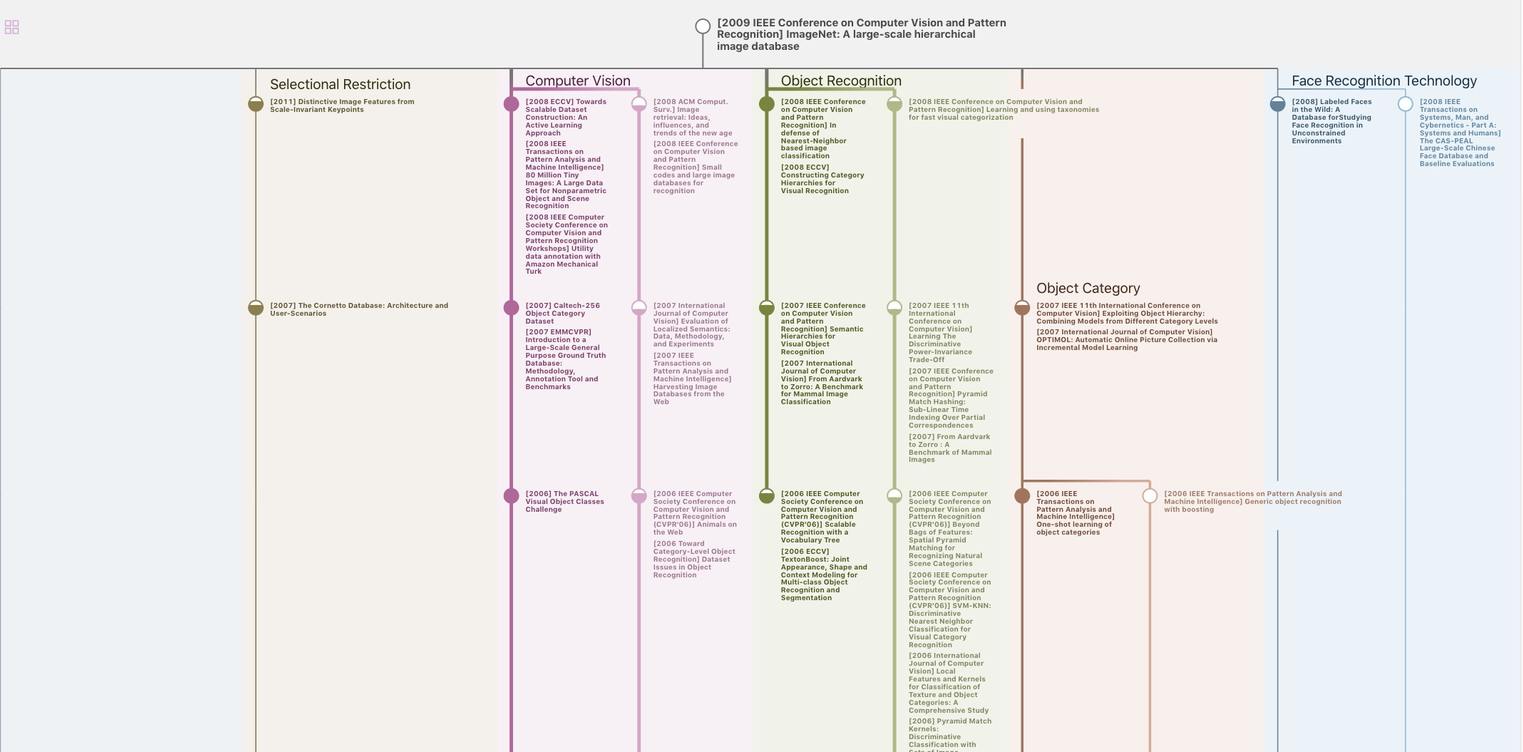
生成溯源树,研究论文发展脉络
Chat Paper
正在生成论文摘要