A Comparative Study of Deep Learning Approaches for Day-Ahead Load Forecasting of an Electric Car Fleet
DATABASE AND EXPERT SYSTEMS APPLICATIONS - DEXA 2021 WORKSHOPS(2021)
摘要
The charging of electric cars affects the performance, efficiency, and required capacity of the electric grid especially where a large electric car fleet located close together simultaneously charges off the same local transformer. Therefore, an accurate load forecasting is required for the reliable and efficient operation of a power system. In this study, three deep learning algorithms, including long short term memory, bidirectional long short term memory, and gated recurrent units are employed and compared in forecasting the aggregate load for the charging of a fleet of electric cars. The developed models were trained and tested on a real-world data set that was collected from 1000 electric vehicles across Canada during 2017-2019. The bidirectional long short term memory algorithm possesses the lowest mean absolute error, mean absolute percentage error and root mean square error among the used methods and is best suited for forecasting the load of electric cars fleet.
更多查看译文
关键词
Electric cars,Charging load,LSTM,Bi-LSTM,GRU,Long range BEVs,Mid-range EVs,Short range EVs
AI 理解论文
溯源树
样例
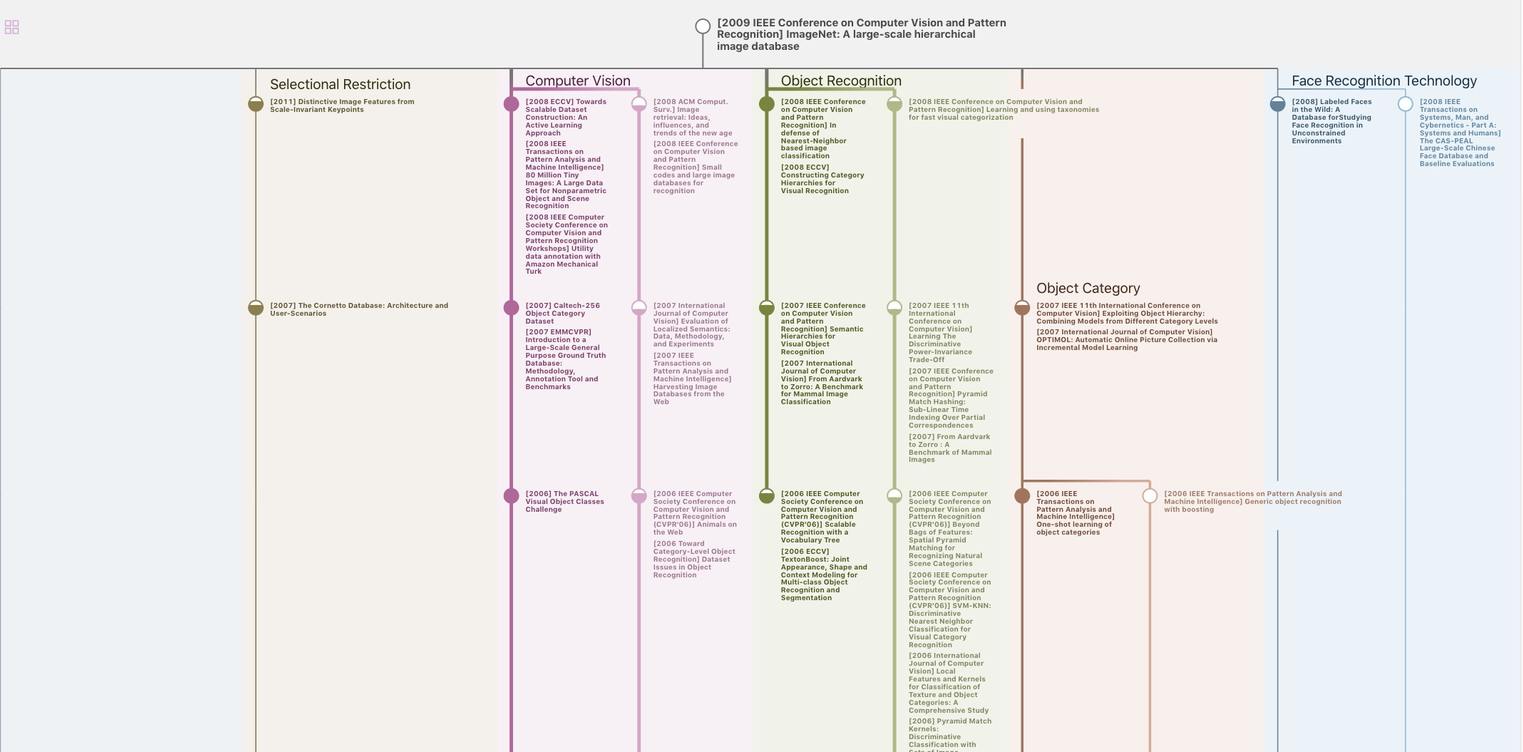
生成溯源树,研究论文发展脉络
Chat Paper
正在生成论文摘要