An Unsupervised Data-Driven Approach for Wind Turbine Blade Damage Detection under Passive Acoustics-Based Excitation
Wind engineering(2022)
摘要
Existing passive acoustics-based techniques for wind turbine blade damage detection lack the robustness and adaptability necessary for an operational implementation due to their physics- and model-based dependency. In contrast, this study develops an entirely unsupervised, data-driven damage detection technique. The novelty of the technique lies in (i) the development and comparison of spectral and cepstral-domain features for the robust characterization of the cavity-internal acoustics, (ii) the use of autoencoder networks to reduce the effects of non-stationary acoustic excitation, and (iii) the exclusion of labeled or damage-case data in the training set. The technique was successfully demonstrated on a wind turbine blade section inflicted with damage of various sizes, types, and locations, and subjected to airflow-induced passive acoustic excitation provided by a wind tunnel. Damage detection accuracy up to 99.82% was achieved for some damage types.
更多查看译文
关键词
Data-driven,acoustics,structural health monitoring,wind turbine blades,unsupervised
AI 理解论文
溯源树
样例
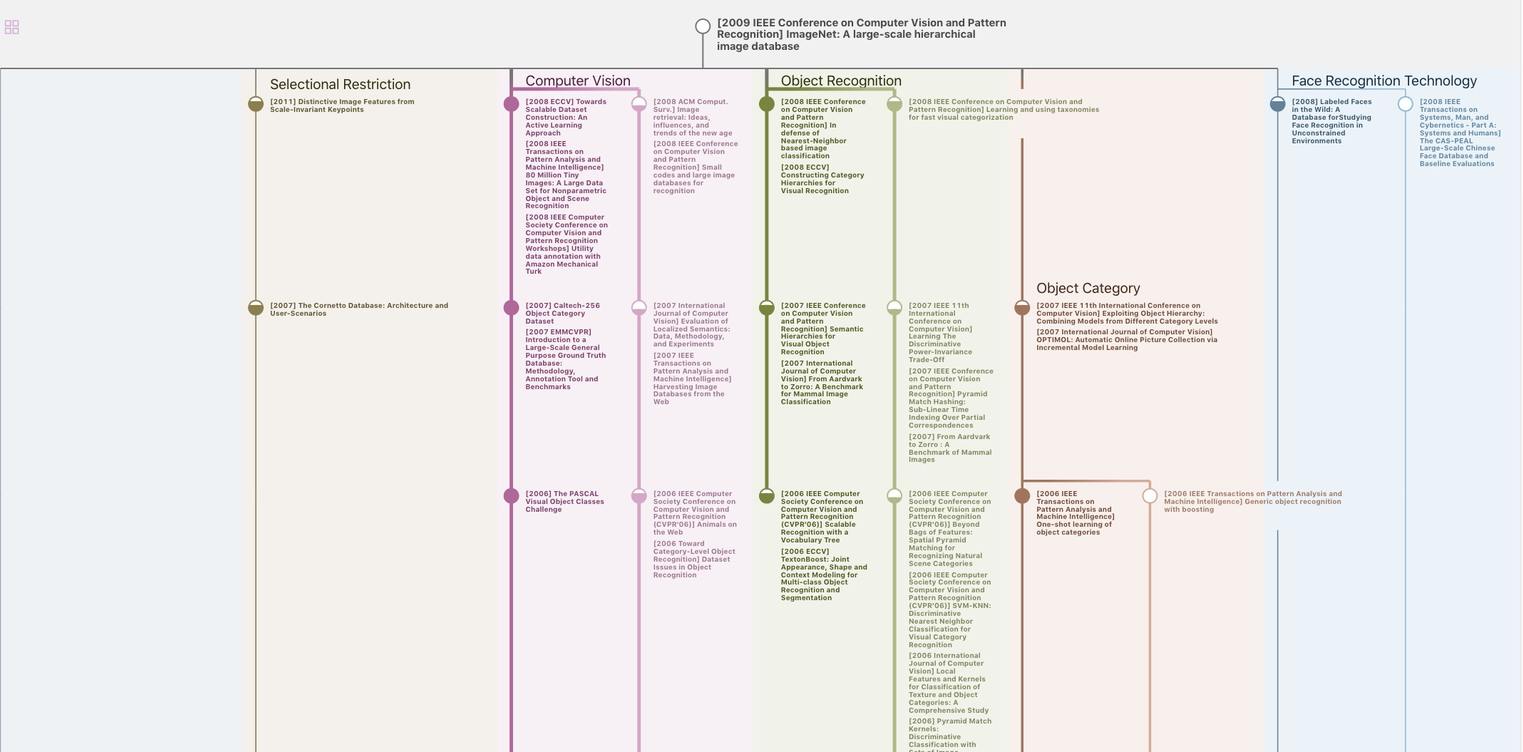
生成溯源树,研究论文发展脉络
Chat Paper
正在生成论文摘要