CT-based deep learning enables early postoperative recurrence prediction for intrahepatic cholangiocarcinoma
SCIENTIFIC REPORTS(2022)
摘要
Preoperatively accurate evaluation of risk for early postoperative recurrence contributes to maximizing the therapeutic success for intrahepatic cholangiocarcinoma (iCCA) patients. This study aimed to investigate the potential of deep learning (DL) algorithms for predicting postoperative early recurrence through the use of preoperative images. We collected the dataset, including preoperative plain computed tomography (CT) images, from 41 patients undergoing curative surgery for iCCA at multiple institutions. We built a CT patch-based predictive model using a residual convolutional neural network and used fivefold cross-validation. The prediction accuracy of the model was analyzed. We defined early recurrence as recurrence within a year after surgical resection. Of the 41 patients, early recurrence was observed in 20 (48.8%). A total of 71,081 patches were extracted from the entire segmented tumor area of each patient. The average accuracy of the ResNet model for predicting early recurrence was 98.2% for the training dataset. In the validation dataset, the average sensitivity, specificity, and accuracy were 97.8%, 94.0%, and 96.5%, respectively. Furthermore, the area under the receiver operating characteristic curve was 0.994. Our CT-based DL model exhibited high predictive performance in projecting postoperative early recurrence, proposing a novel insight into iCCA management.
更多查看译文
关键词
intrahepatic cholangiocarcinoma,postoperative recurrence prediction,deep learning,ct-based
AI 理解论文
溯源树
样例
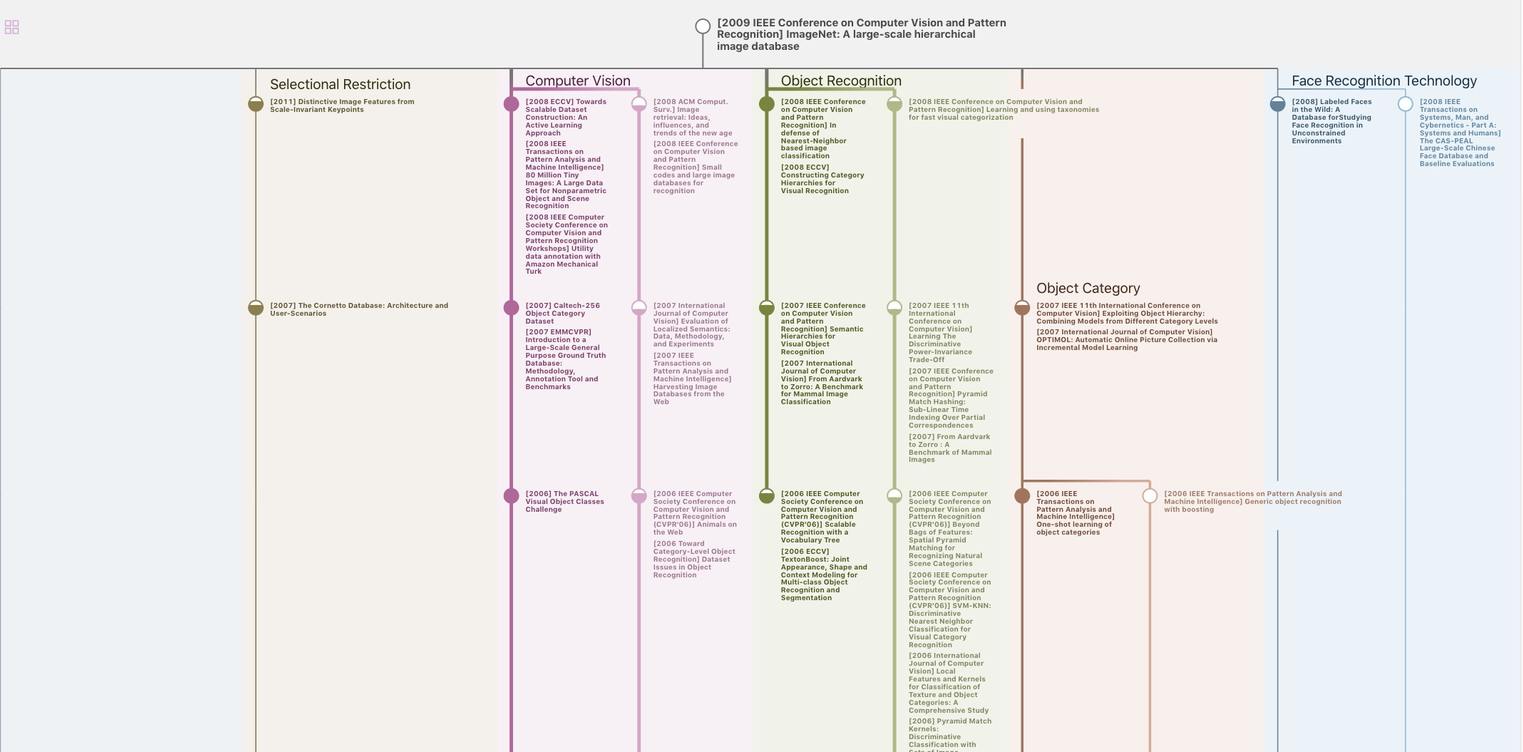
生成溯源树,研究论文发展脉络
Chat Paper
正在生成论文摘要