Mask-guided Vision Transformer (Mg-Vit) for Few-Shot Learning
IEEE Transactions on Neural Networks and Learning Systems(2024)
摘要
Learning with little data is challenging but often inevitable in various application scenarios where the labeled data is limited and costly. Recently, few-shot learning (FSL) gained increasing attention because of its generalizability of prior knowledge to new tasks that contain only a few samples. However, for data-intensive models such as vision transformer (ViT), current fine-tuning based FSL approaches are inefficient in knowledge generalization and thus degenerate the downstream task performances. In this paper, we propose a novel mask-guided vision transformer (MG-ViT) to achieve an effective and efficient FSL on ViT model. The key idea is to apply a mask on image patches to screen out the task-irrelevant ones and to guide the ViT to focus on task-relevant and discriminative patches during FSL. Particularly, MG-ViT only introduces an additional mask operation and a residual connection, enabling the inheritance of parameters from pre-trained ViT without any other cost. To optimally select representative few-shot samples, we also include an active learning based sample selection method to further improve the generalizability of MG-ViT based FSL. We evaluate the proposed MG-ViT on both Agri-ImageNet classification task and ACFR apple detection task with gradient-weighted class activation mapping (Grad-CAM) as the mask. The experimental results show that the MG-ViT model significantly improves the performance when compared with general fine-tuning based ViT models, providing novel insights and a concrete approach towards generalizing data-intensive and large-scale deep learning models for FSL.
更多查看译文
关键词
Few-Shot Learning,Semi-Supervised Learning,Machine Vision,Unsupervised Learning,Transfer Learning
AI 理解论文
溯源树
样例
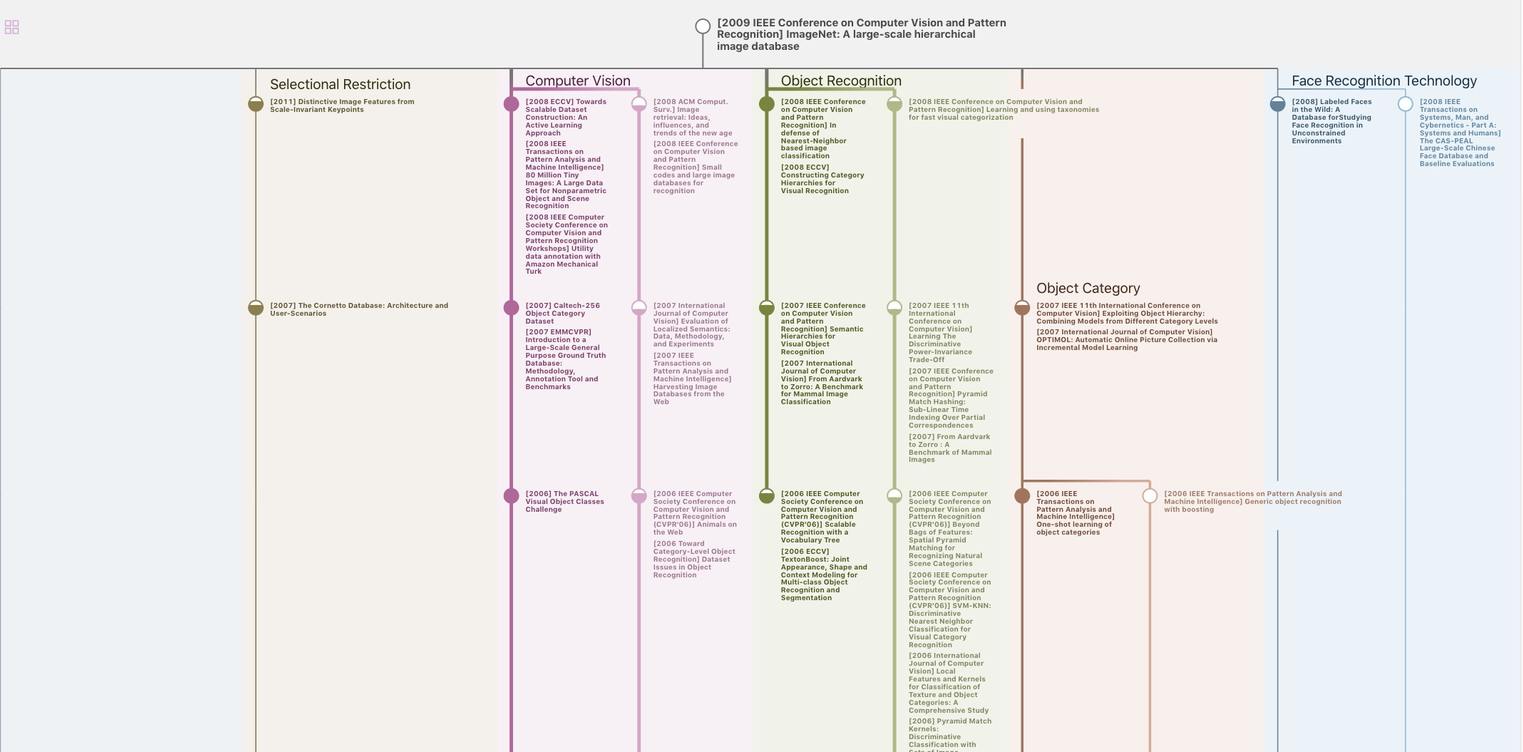
生成溯源树,研究论文发展脉络
Chat Paper
正在生成论文摘要