BPE beyond Word Boundary: How NOT to use Multi Word Expressions in Neural Machine Translation
PROCEEDINGS OF THE THIRD WORKSHOP ON INSIGHTS FROM NEGATIVE RESULTS IN NLP (INSIGHTS 2022)(2022)
摘要
BPE tokenization merges characters into longer tokens by finding frequently occurring contiguous patterns within the word boundary. An intuitive relaxation would be to extend a BPE vocabulary with multi-word expressions (MWEs): bigrams (in_a), trigrams (out_of_the), and skip-grams (he.his). In the context of Neural Machine Translation (NMT), we replace the least frequent subword/whole-word tokens with the most frequent MWEs. We find that these modifications to BPE end up hurting the model, resulting in a net drop of BLEU and chrF scores across two language pairs. We observe that naively extending BPE beyond word boundaries results in incoherent tokens which are themselves better represented as individual words. Moreover, we find that Pointwise Mutual Information (PMI) instead of frequency finds better MWEs (e.g., New_Y ork, Statue_of_Liberty, neither.nor) which consistently improves translation performance. We release all code at https://github.com/pegasus-lynx/mwe-bpe.
更多查看译文
AI 理解论文
溯源树
样例
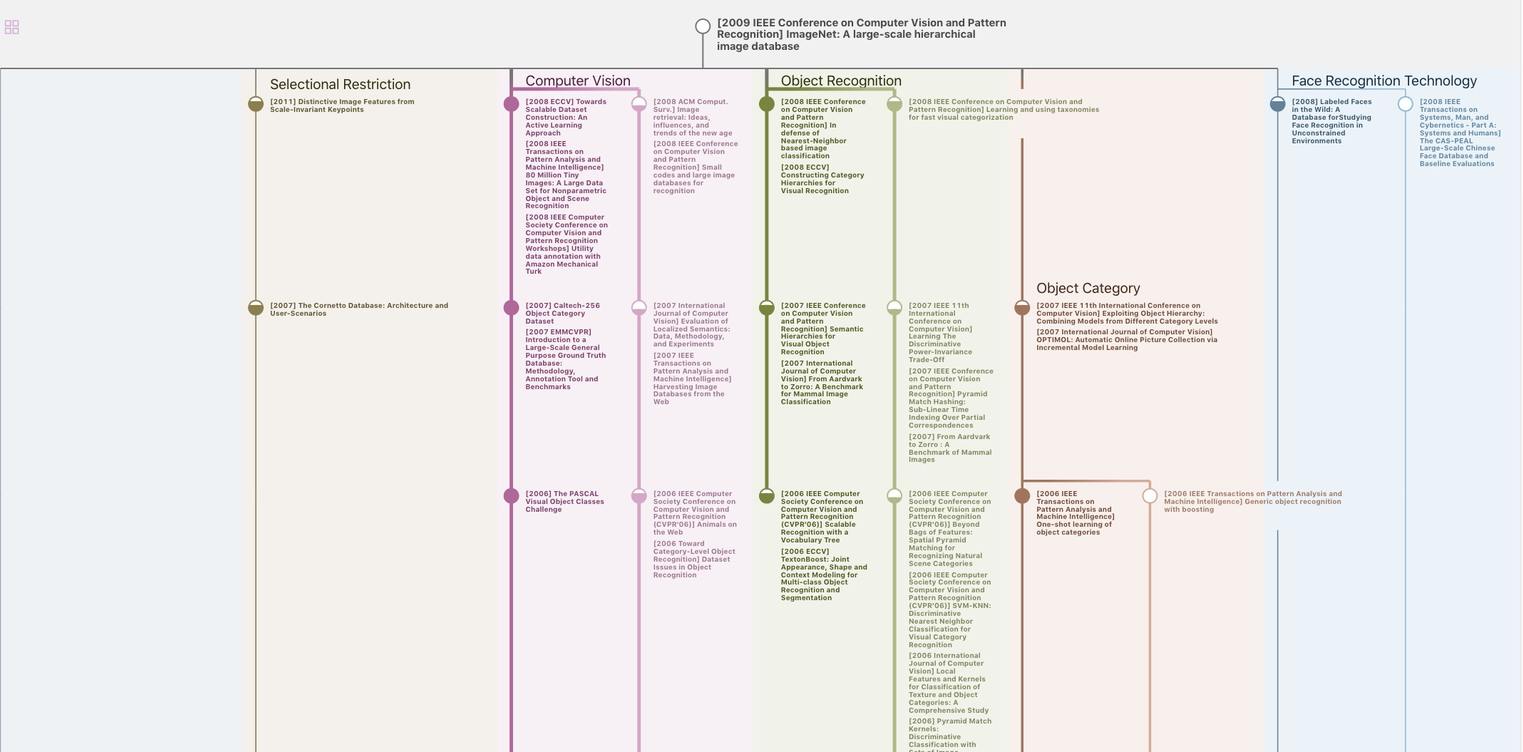
生成溯源树,研究论文发展脉络
Chat Paper
正在生成论文摘要