Sam’s Net: A Self-Augmented Multistage Deep-Learning Network for End-to-End Reconstruction of Limited Angle CT
IEEE Transactions on Medical Imaging(2022)
摘要
Limited angle reconstruction is a typical ill-posed problem in computed tomography (CT). Given incomplete projection data, images reconstructed by conventional analytical algorithms and iterative methods suffer from severe structural distortions and artifacts. In this paper, we proposed a self-augmented multi-stage deep-learning network (Sam’s Net) for end-to-end reconstruction of limited angle CT. With the merit of the alternating minimization technique, Sam’s Net integrates multi-stage self-constraints into cross-domain optimization to provide additional constraints on the manifold of neural networks. In practice, a sinogram completion network (SCNet) and artifact suppression network (ASNet), together with domain transformation layers constitute the backbone for cross-domain optimization. An online self-augmentation module was designed following the manner defined by alternating minimization, which enables a self-augmented learning procedure and multi-stage inference manner. Besides, a substitution operation was applied as a hard constraint for the solution space based on the data fidelity and a learnable weighting layer was constructed for data consistency refinement. Sam’s Net forms a new framework for ill-posed reconstruction problems. In the training phase, the self-augmented procedure guides the optimization into a tightened solution space with enriched diverse data distribution and enhanced data consistency. In the inference phase, multi-stage prediction can improve performance progressively. Extensive experiments with both simulated and practical projections under 90-degree and 120-degree fan-beam configurations validate that Sam’s Net can significantly improve the reconstruction quality with high stability and robustness.
更多查看译文
关键词
Computed tomography (CT),limited angle reconstruction,deep learning,alternating minimization,online augmentation
AI 理解论文
溯源树
样例
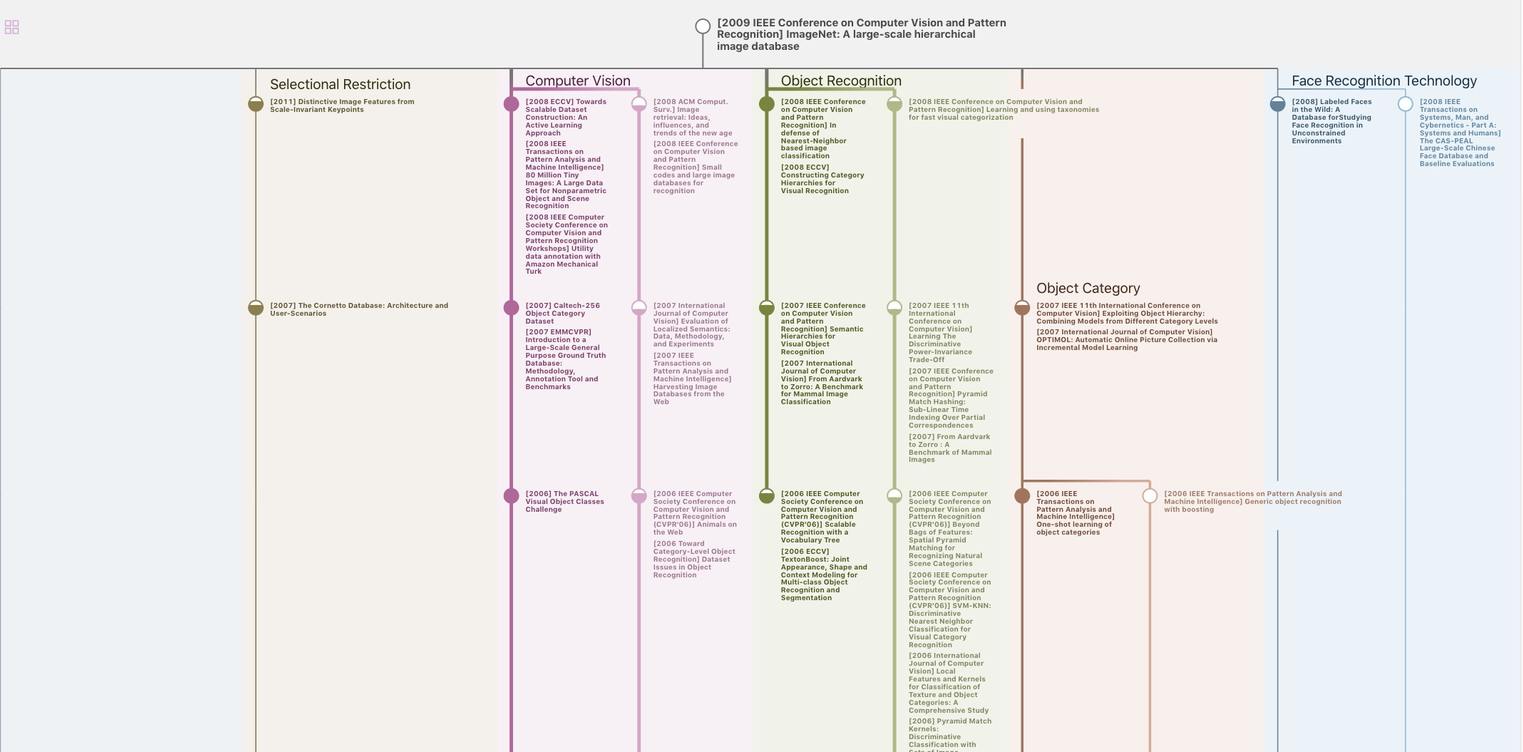
生成溯源树,研究论文发展脉络
Chat Paper
正在生成论文摘要