Personalized Federated Learning via Maximizing Correlation with Sparse and Hierarchical Extensions
ArXiv(2021)
摘要
Federated Learning (FL) is a collaborative machine learning technique to train a global model without obtaining clients’ private data. The main challenges in FL are statistical diversity among clients, limited computing capability among client equipments and the excessive communication overhead and long latency between server and clients. To address these problems, we propose a novel personalized federated learning via maximizing correlation (pFedMac), and further extend it to sparse and hierarchical models. By minimizing loss functions including the properties of an approximated `1-norm and the hierarchical correlation, the performance on statistical diversity data is improved and the communicational and computational loads required in the network are reduced. Theoretical proofs show that pFedMac performs better than the `2-norm distance based personalization methods. Experimentally, we demonstrate the benefits of this sparse hierarchical personalization architecture compared with the state-of-the-art personalization methods and their extensions (e.g. pFedMac achieves 99.75% accuracy on MNIST and 87.27% accuracy on Synthetic under heterogeneous and non-i.i.d data distributions).
更多查看译文
AI 理解论文
溯源树
样例
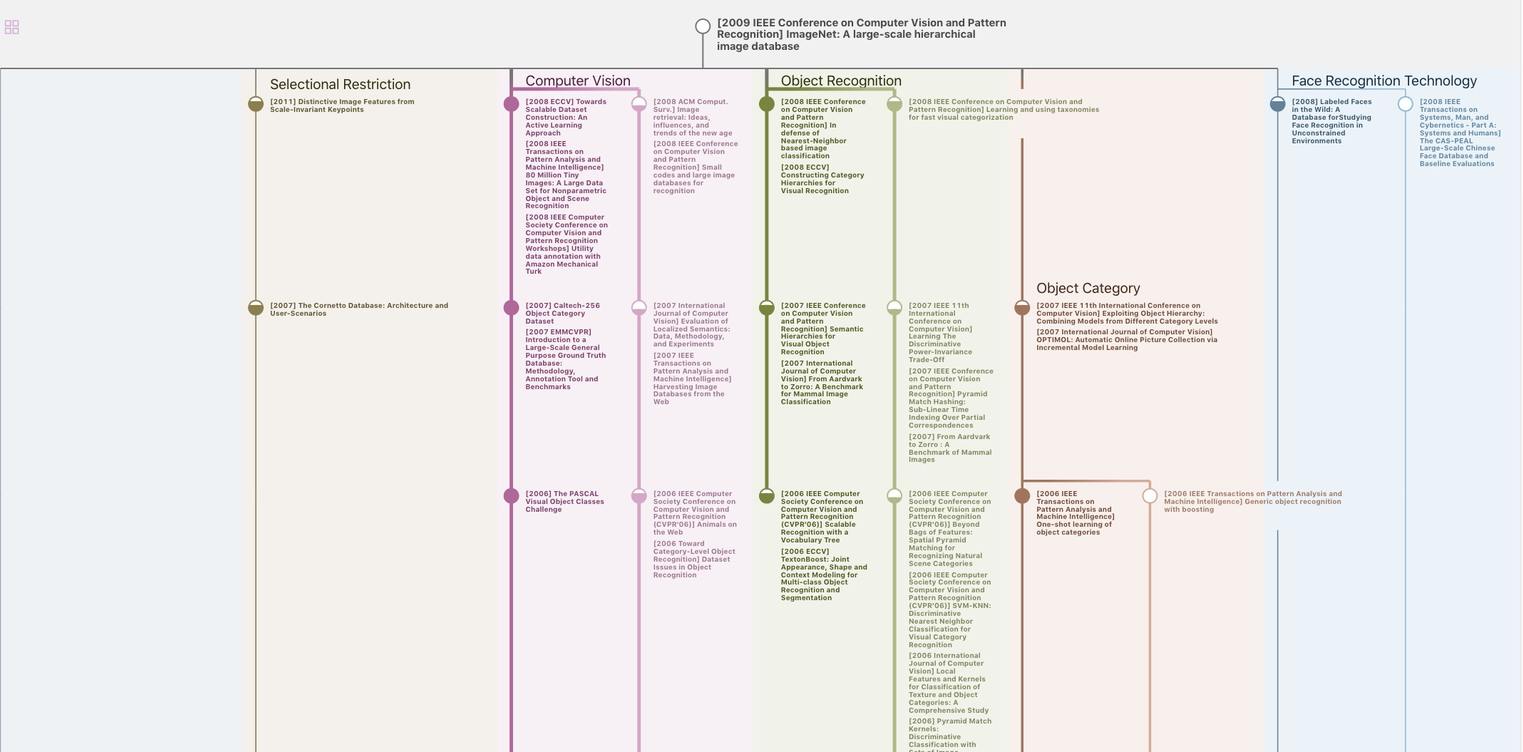
生成溯源树,研究论文发展脉络
Chat Paper
正在生成论文摘要