Data Management Opportunities for Foundation Models
Conference on Innovative Data Systems Research (CIDR)(2022)
摘要
There is a paradigm shift in industrial machine learning pipelines where data is becoming one of the most important factors when building performant systems [5, 18, 17, 13, 9, 3]. Previously, ML pipelines followed a more“model-centric” paradigm, where engineers customized model architectures and hand curated features for training. These pipelines are being replaced by“foundation model”[4] ecosystems that follow a “data-centric” [14] viewpoint—commoditized architectures (e.g., Transformers [20] or MLPs) are trained without manual labels (i.e., with self-supervision) on massive corpora and adapted to hundreds of downstream tasks. In this new paradigm, the differentiating factor between models is the data they are “fed”, not the architecture. Managing these foundation models is essentially the problem of managing their data lifecycle.
更多查看译文
AI 理解论文
溯源树
样例
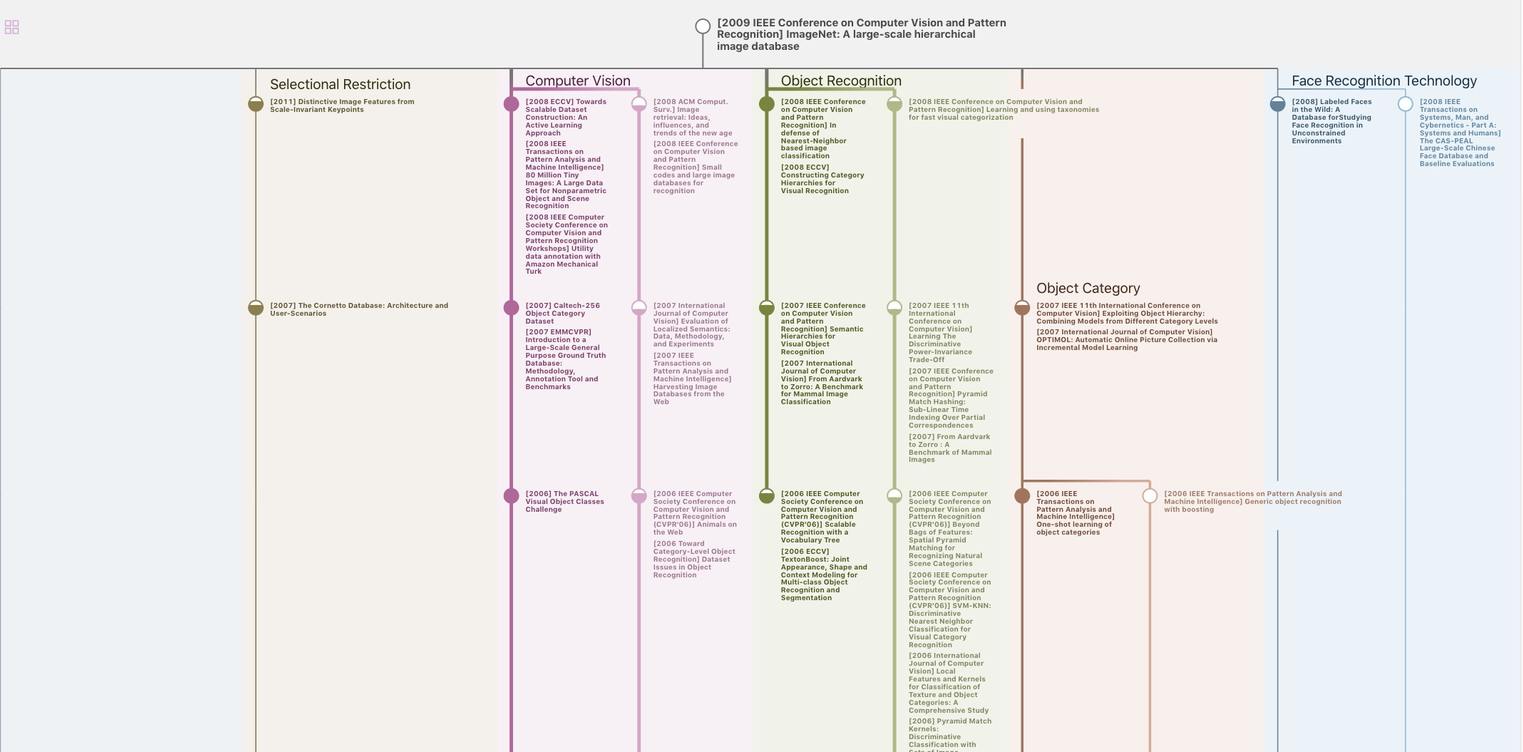
生成溯源树,研究论文发展脉络
Chat Paper
正在生成论文摘要