Improved Deep Convolutional Embedded Clustering with Re-Selectable Sample Training.
Pattern recognition(2022)
摘要
The deep clustering algorithm can learn the latent features of the embedded subspace, and further realize the clustering of samples in the feature space. The existing deep clustering algorithms mostly integrate neural networks and traditional clustering algorithms. However, for sample sets with many noise points, the effect of the clustering remains unsatisfactory. To address this issue, we propose an improved deep convolutional embedded clustering algorithm using reliable samples (IDCEC) in this paper. The algorithm first uses the convolutional autoencoder to extract features and cluster the samples. Then we select reliable samples with pseudo-labels and pass them to the convolutional neural network for training to get a better clustering model. We construct a new loss function for backpropagation training and implement an unsupervised deep clustering method. To verify the performance of the method proposed in this paper, we conducted experimental tests on standard data sets such as MNIST and USPS. Experimental results show that our method has better performance compared to traditional clustering algorithms and the state-of-the-art deep clustering algorithm under four clustering metrics.(c) 2022 Elsevier Ltd. All rights reserved.
更多查看译文
关键词
Unsupervised clustering,Deep embedded clustering,Autoencoder,Reliable samples
AI 理解论文
溯源树
样例
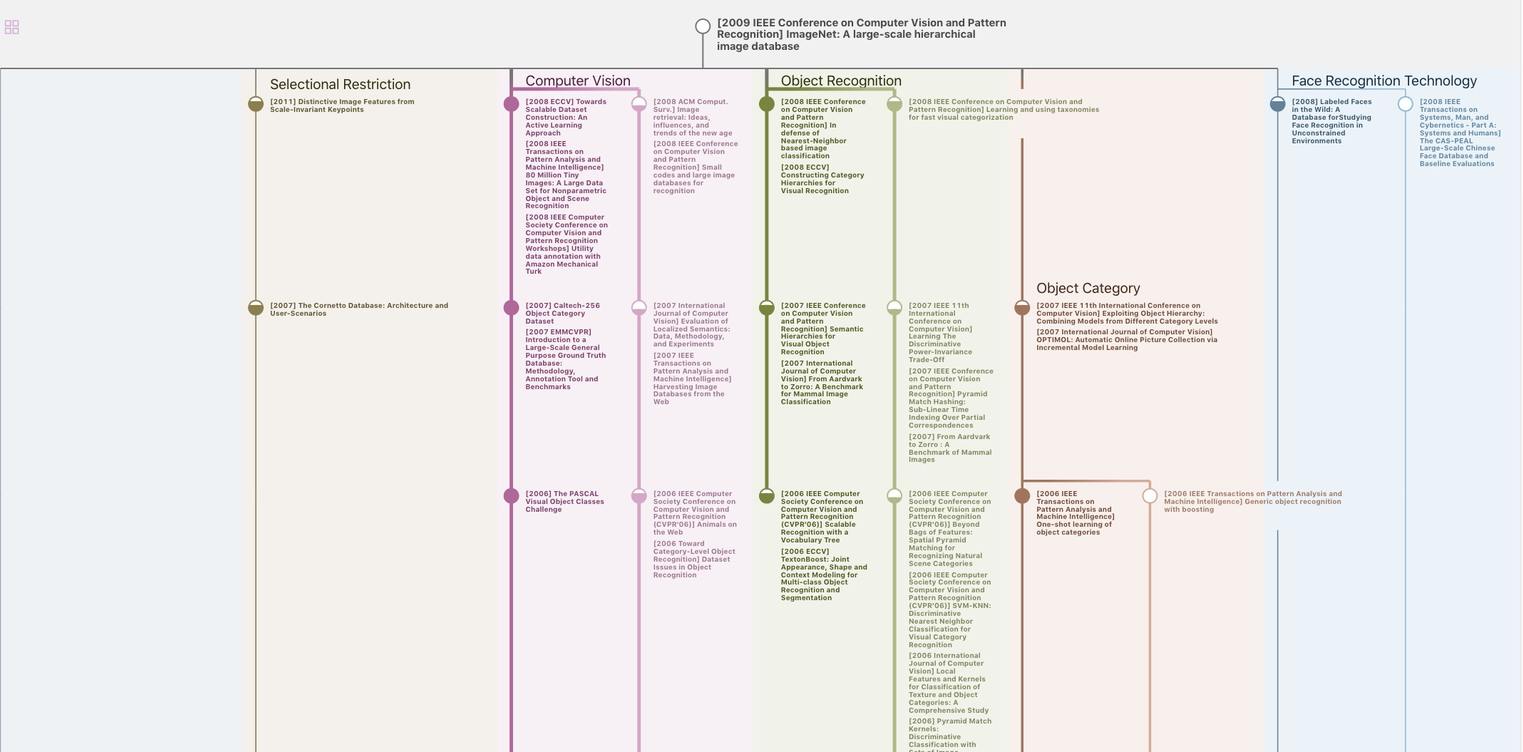
生成溯源树,研究论文发展脉络
Chat Paper
正在生成论文摘要